AI algorithms for fitting GARCH parameters to empirical financial data
Physica A: Statistical Mechanics and its Applications(2022)
摘要
We use Deep Artificial Neural Networks (ANNs) to estimate GARCH parameters for empirical financial time series. The algorithm we develop, allows us to fit autocovariance of squared returns of financial data, with certain time lags, the second order statistical moment, and the fourth order standardised moment. We have compared the time taken for the ANN algorithm to predict parameters for many time windows (around 4000), to that of the time taken for the Maximum Likelihood Estimation (MLE) methods of MatLabs’s inbuilt statistical and econometric toolbox. The algorithm developed predicts all GARCH parameters in around 0.1 s, compared to the 11 seconds of the MLE method. Furthermore, we use a Model Confidence Set analysis to determine how accurate our parameter prediction algorithm is, when predicting volatility. The volatility prediction of different securities obtained employing the ANN has an error of around 25%, compared to 40% for the MLE methods.
更多查看译文
关键词
C14,C22
AI 理解论文
溯源树
样例
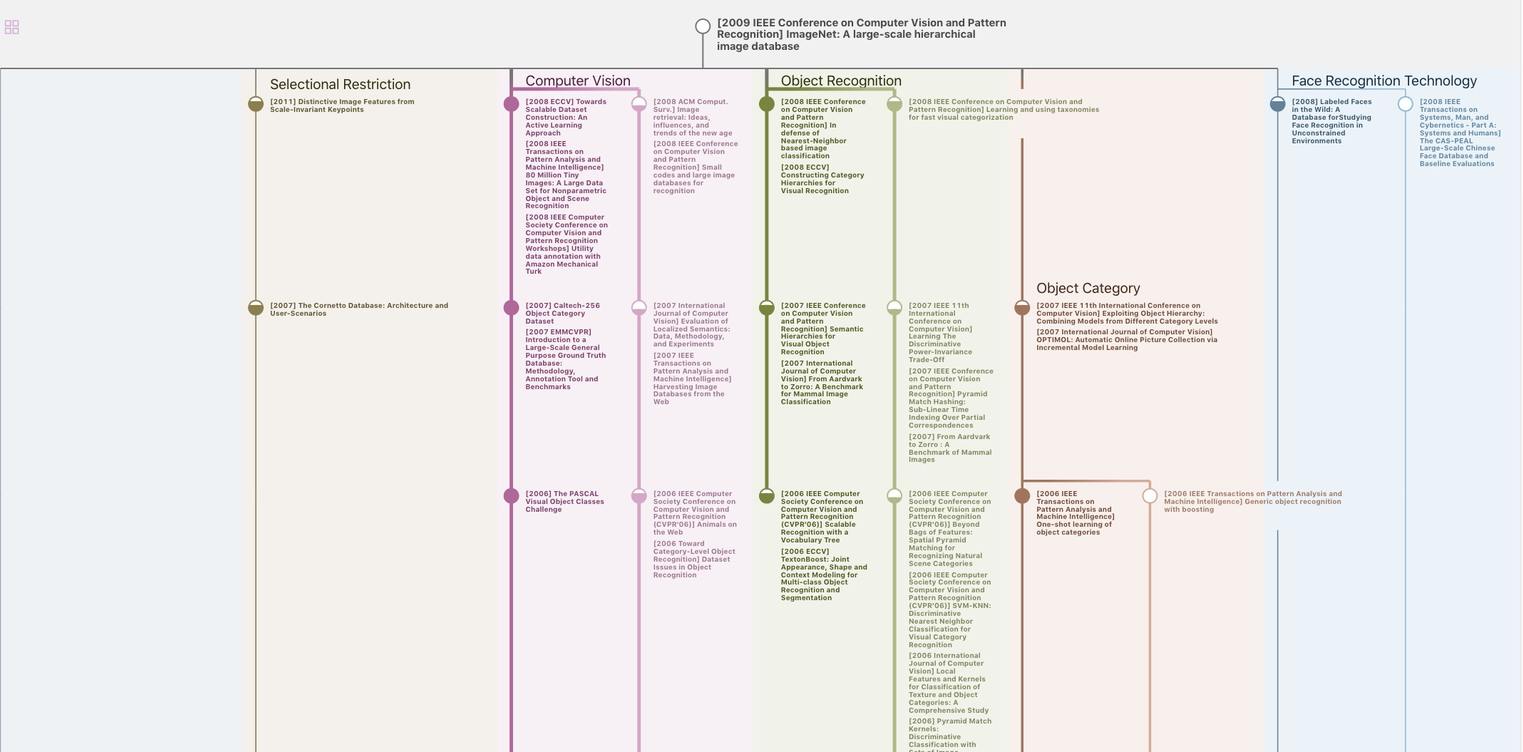
生成溯源树,研究论文发展脉络
Chat Paper
正在生成论文摘要