System-level optimization in spectroscopic photoacoustic imaging of prostate cancer
Photoacoustics(2022)
摘要
This study presents a system-level optimization of spectroscopic photoacoustic (PA) imaging for prostate cancer (PCa) detection in three folds. First, we present a spectral unmixing model to segregate spectral system error (SSE). We constructed two noise models (NMs) for the laser spectrotemporal fluctuation and the ultrasound system noise. We used these NMs in linear spectral unmixing to denoise and to achieve high temporal resolution. Second, we employed a simulation-aided wavelength optimization to select the most effective subset of wavelengths. NMs again were considered so that selected wavelengths were not only robust to the collinearity of optical absorbance, but also to noise. Third, we quantified the effect of frame averaging on improving spectral unmixing accuracy through theoretical analysis and numerical validation. To validate the whole framework, we performed comprehensive studies in simulation and an in vivo experiment which evaluated prostate-specific membrane antigen (PSMA) expression in PCa on a mice model. Both simulation analysis and in vivo studies confirmed that the proposed framework significantly enhances image signal-to-noise ratio (SNR) and spectral unmixing accuracy. It enabled more sensitive and faster PCa detection. Moreover, the proposed framework can be generalized to other spectroscopic PA imaging studies for noise reduction, wavelength optimization, and higher temporal resolution.
更多查看译文
关键词
Spectroscopic photoacoustic imaging,Prostate cancer,Spectral unmixing,Spectral system error,Frame averaging,Wavelength selection
AI 理解论文
溯源树
样例
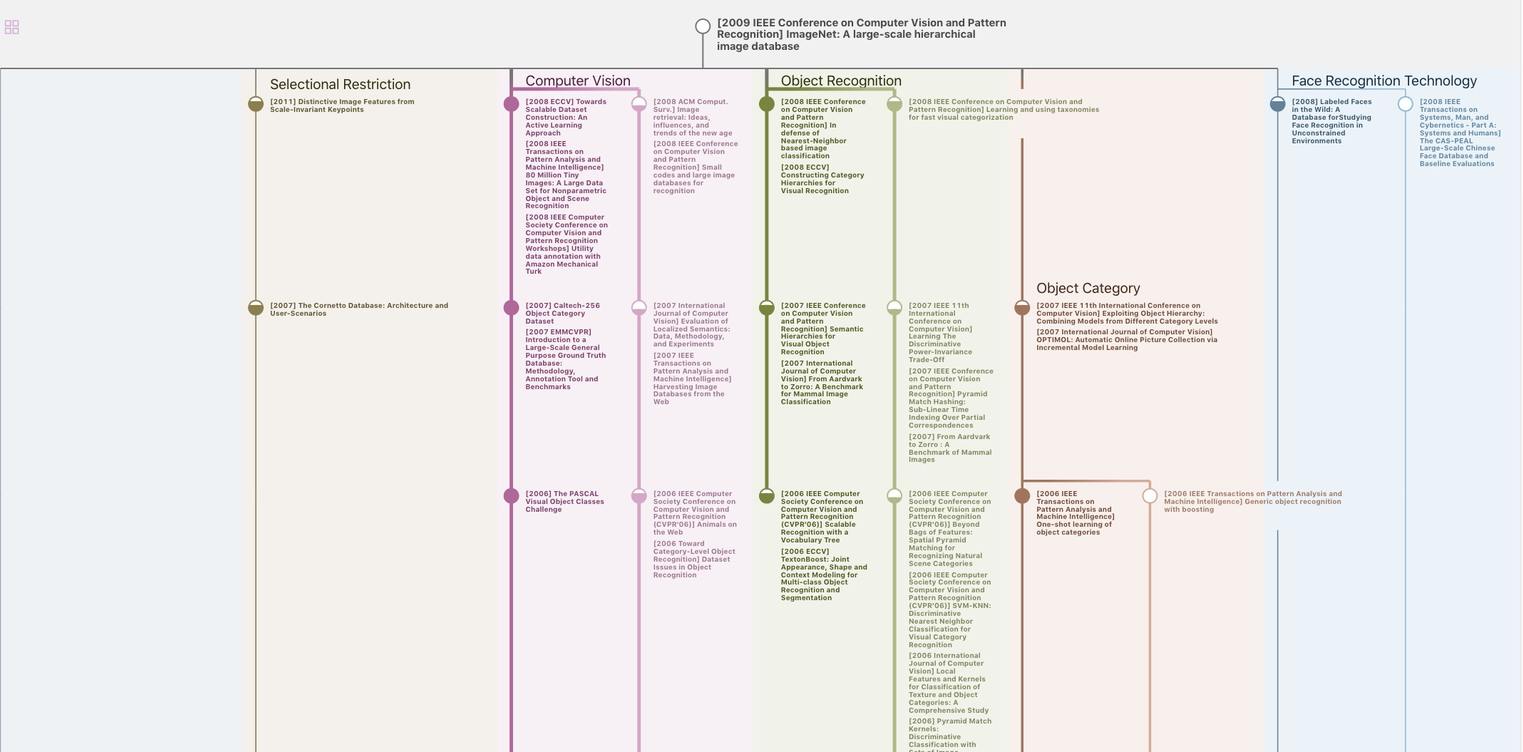
生成溯源树,研究论文发展脉络
Chat Paper
正在生成论文摘要