Load Prediction Based on Optimization Ant Colony Algorithm
JOURNAL OF ELECTRICAL ENGINEERING & TECHNOLOGY(2022)
摘要
Short-term load in the power system is associated with huge computational consumption and low model utilization. Large input fluctuation tends to increase the training error of the neural network prediction model and reduce its generalization ability. To cope with this problem, this study aimed to introduce a method of radial basis function neural network algorithm based on least squares support vector machine algorithm. Based on the electricity market in an area of Yunnan province, the short-term loads were forecasted with historical data. First, the ant colony algorithm was improved using the chaos theory. Second, the improved ant colony was used to search least squares support vector machine and output the optimal parameters of the model. Then, the optimized model was used to train the data samples, and the output regression machine was used to provide better structures and parameters for the radial basis function neural network. The findings showed that compared with multiple prediction methods, the algorithm in this paper reduces the learning time and improves the fitting degree of the algorithm on the basis of improving the prediction accuracy. It shows that the algorithm in this paper has great advantages and good application prospects.
更多查看译文
关键词
Ant colony algorithm, Load forecasting, Least squares support vector machine, Optimization, Radial basis function neural network
AI 理解论文
溯源树
样例
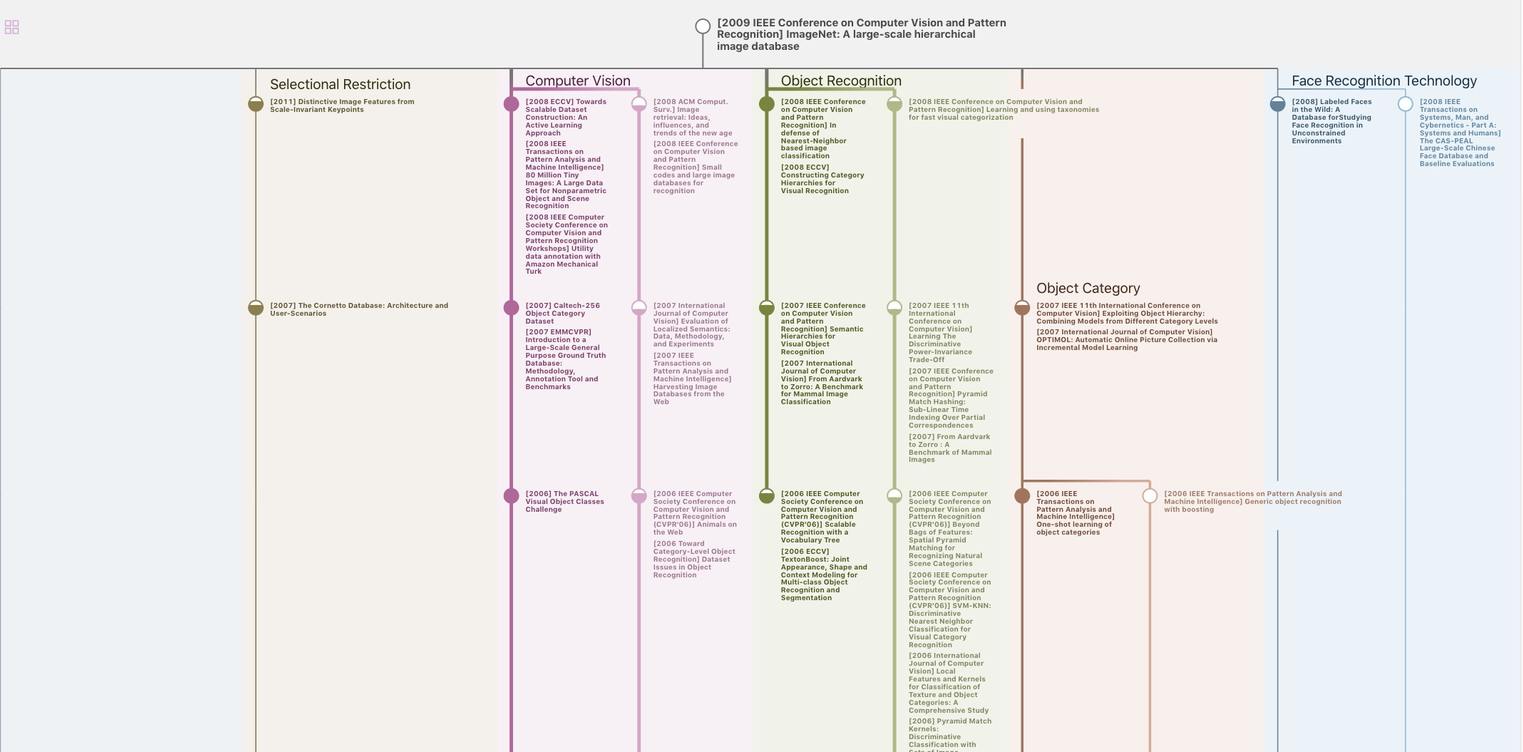
生成溯源树,研究论文发展脉络
Chat Paper
正在生成论文摘要