A large-scale real-world comparative study using pre-COVID lockdown and post-COVID lockdown data on predicting shipment times of therapeutics in e-pharmacy supply chains
INTERNATIONAL JOURNAL OF PHYSICAL DISTRIBUTION & LOGISTICS MANAGEMENT(2022)
摘要
Purpose The purpose of this study is to present a large-scale real-world comparative study using pre-COVID lockdown data versus post-COVID lockdown data on predicting shipment times of therapeutic supplies in e-pharmacy supply chains and show that our proposed methodology is robust to lockdown effects. Design/methodology/approach The researchers used organic data of over 5.9 million records of therapeutic shipments, with 2.87 million records collected pre-COVID lockdown and 3.03 million records collected post-COVID lockdown. The researchers built various Machine Learning (ML) classifier models on the two datasets, namely, Random Forest (RF), Extra Trees (XRT), Decision Tree (DT), Multi-Layer Perceptron (MLP), XGBoost (XGB), CatBoost (CB), Linear Stochastic Gradient Descent (SGD) and the Linear Naive Bayes (NB). Then, the researchers stacked these base models and built meta models on top of them. Further, the researchers performed a detailed comparison of the performances of ML models on pre-COVID lockdown and post-COVID lockdown datasets. Findings The proposed approach attains performance of 93.5% on real-world post-COVID lockdown data and 91.35% on real-world pre-COVID lockdown data. In contrast, the turn-around times (TAT) provided by therapeutic supply logistics providers are 62.91% accurate compared to reality in post-COVID lockdown times and 73.68% accurate compared to reality pre-COVID lockdown times. Hence, it is clear that while the TAT provided by logistics providers has deteriorated in the post-pandemic business climate, the proposed method is robust to handle pandemic lockdown effects on e-pharmacy supply chains. Research limitations/implications The implication of the study provides a novel ML-based framework for predicting the shipment times of therapeutics, diagnostics and vaccines, and it is robust to COVID-19 lockdown effects. Practical implications E-pharmacy companies can readily adopt the proposed approach to enhance their supply chain management (SCM) capabilities and build resilience during COVID lockdown times. Originality/value The present study is one of the first to perform a large-scale real-world comparative analysis on predicting therapeutic supply shipment times in the e-pharmacy supply chain with novel ML ensemble stacking, obtaining robust results in these COVID lockdown times.
更多查看译文
关键词
Real-world comparative study, Pre-COVID lockdown, Post-COVID lockdown, e-pharmacy supply chain, Shipment time prediction, Analytics, Machine Learning
AI 理解论文
溯源树
样例
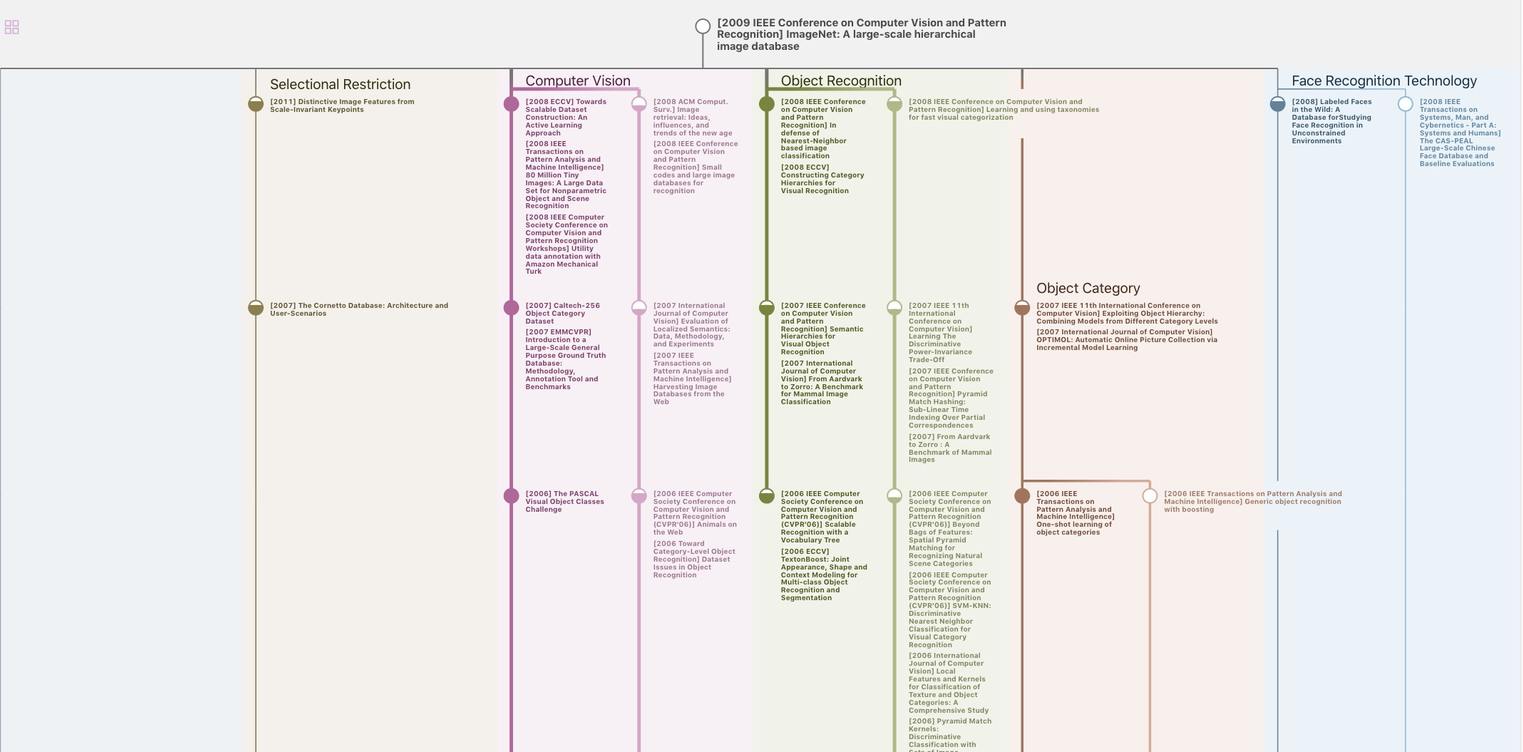
生成溯源树,研究论文发展脉络
Chat Paper
正在生成论文摘要