Privacy-Preserving Distributed Kalman Filtering
IEEE TRANSACTIONS ON SIGNAL PROCESSING(2022)
摘要
Distributed Kalman filtering techniques enable agents of a multiagent network to enhance their ability to track a system and learn from local cooperation with neighbors. Enabling this cooperation, however, requires agents to share information, which raises the question of privacy. This paper proposes a privacy-preserving distributed Kalman filter (PP-DKF) that protects local information of agents by restricting and obfuscating the information exchanged. The derived PP-DKF embeds two state-of-the-art average consensus techniques that guarantee agent privacy. The resulting PP-DKF utilizes noise injection-based and decomposition-based privacy-preserving techniques to implement a robust distributed Kalman filtering solution against perturbation. We characterize the performance and convergence of the proposed PP-DKF and demonstrate its robustness against the injected noise variance. We also assess the privacy-preserving properties of the proposed algorithm for two types of adversaries, namely, an external eavesdropper and an honest-but-curious (HBC) agent, by providing bounds on the privacy leakage for both adversaries. Finally, several simulation examples illustrate that the proposed PP-DKF achieves better performance and higher privacy levels than the distributed Kalman filtering solutions employing contemporary privacy-preserving techniques.
更多查看译文
关键词
Kalman filters, Privacy, Estimation, State estimation, Perturbation methods, Optimization, Convergence, Sensor networks, privacy, information fusion, average consensus, distributed Kalman filtering, multiagent systems
AI 理解论文
溯源树
样例
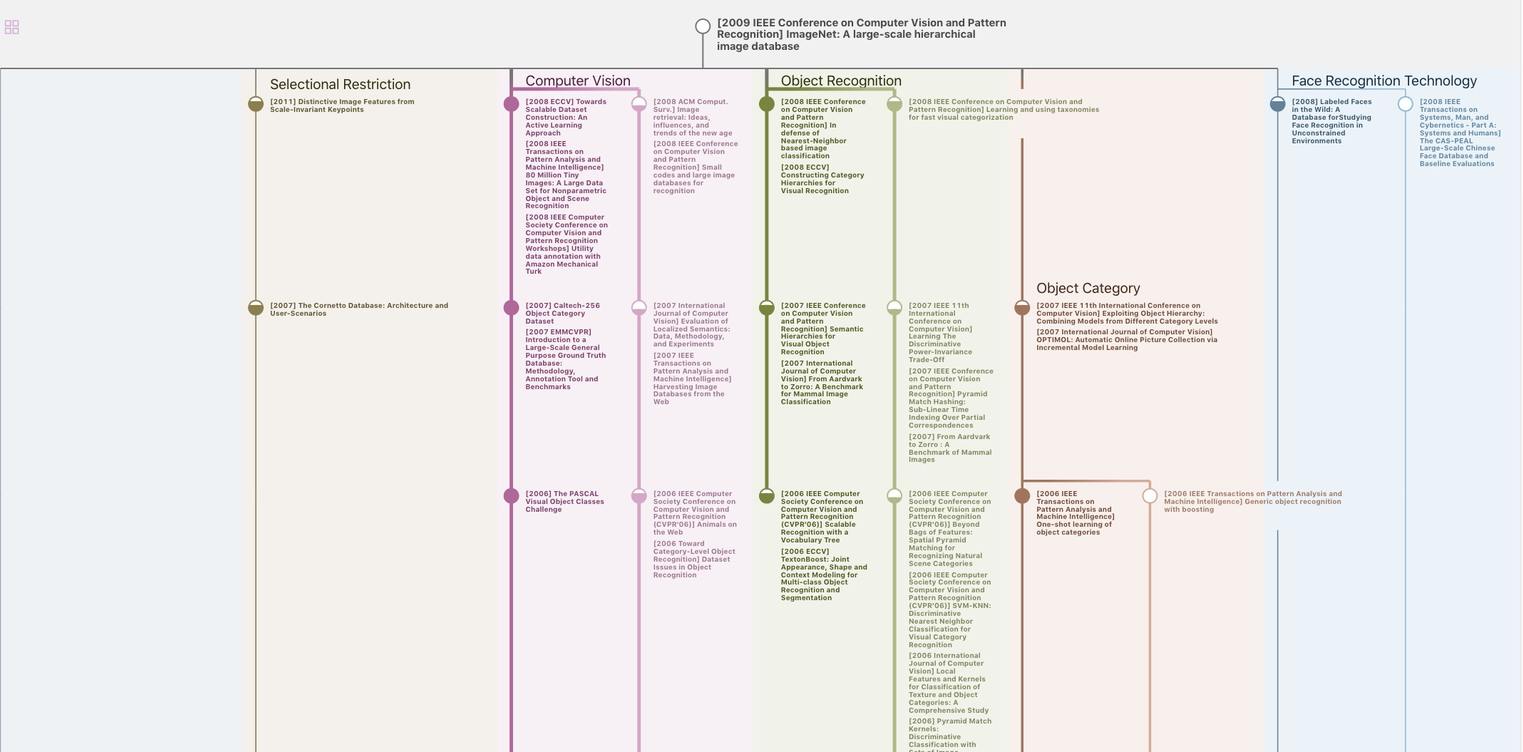
生成溯源树,研究论文发展脉络
Chat Paper
正在生成论文摘要