Recommendation Model Based on Enhanced Graph Convolution That Fuses Review Properties
IEEE TRANSACTIONS ON COMPUTATIONAL SOCIAL SYSTEMS(2023)
摘要
In rating prediction research, how to capture user and item features from review text is a key to improving model prediction accuracy. The sparsity of review text and the accuracy of the description of items in the review text make it difficult to obtain accurate feature representations of users and items by modeling the text content alone. Therefore, it is important to evaluate the usefulness of the reviews at first because not all review texts are valuable. How to analyze the usefulness of a review is a key to modeling the review text. The way previous models use attention to inscribe semantic weights on the review text is not sufficient to indicate the degree of usefulness of a review, so we suggest adding property information to model reviews. Based on this, we propose an interaction recommendation model that is based on enhanced graph convolution and fuses review properties (PGIR), which incorporates property information into text modeling by different activations and matches useful property feature interaction pairs for review text in a self-supervised manner. This allows the model to obtain an accurate feature representation of the review text. In addition, we analyze the high-order connectivity among user-item pairs. Then, we design an enhanced graph convolution method to capture the collaborative signals between users and items and model the dynamic features of users and items on this basis. After extensive experiments conducted on five standard datasets based on Amazon, the results show that the PGIR model achieves a substantial improvement over existing state-of-the-art models in terms of rating prediction. In addition, we experimentally demonstrate the superiority of our proposed property activation method, which further improves the rating prediction performance of the PGIR model.
更多查看译文
关键词
Predictive models, Convolution, Feature extraction, Collaboration, Fuses, Data mining, Bipartite graph, Graph convolutional network, property information, rating prediction, recommendation system, self-supervised
AI 理解论文
溯源树
样例
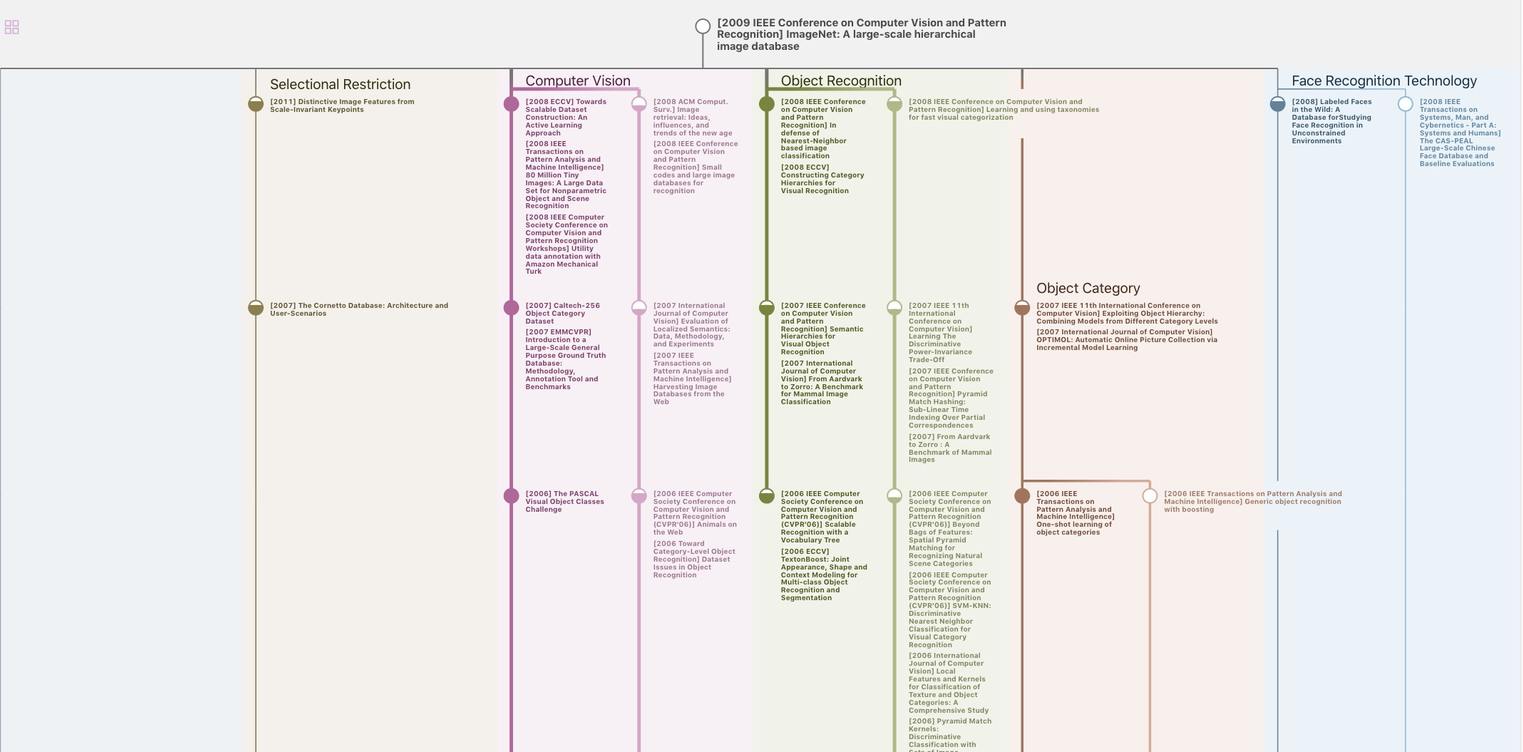
生成溯源树,研究论文发展脉络
Chat Paper
正在生成论文摘要