Fusing frequent sub-sequences in the session-based recommender system
Expert Systems with Applications(2022)
摘要
A session-based recommender system (RS) forecasts the item to be clicked next by taking advantage of the item sequence in the current session. The deep learning models, such as the recurrent neural network (RNN) and the graph neural network (GNN), are recently applied in the session-based recommendation. However, to the best of our knowledge, the existing methods ignore the fusion of the frequent sub-sequences which refer to those item sub-sequences that appear frequently in different sessions. Intuitively, the more frequently an item sequence appears, the more important it could be. In this paper, we propose the frequent sub-sequence modeling (FSM) method, by utilizing both the local and global information of item sequences. More specifically, we use the item sequence in the current session to build a local session graph and the frequent sub-sequences to construct a global session graph. In addition, we design a gated layer to control the contributions of these two session graphs. Our method is capable of learning both the session-level and global-level item embedding, and thus supports accurate predictions. It is tested on four benchmark datasets and the results show that our method is superior to state-of-the-art approaches consistently.
更多查看译文
关键词
Graph neural network,Session-based recommender system,Frequent sub-sequences
AI 理解论文
溯源树
样例
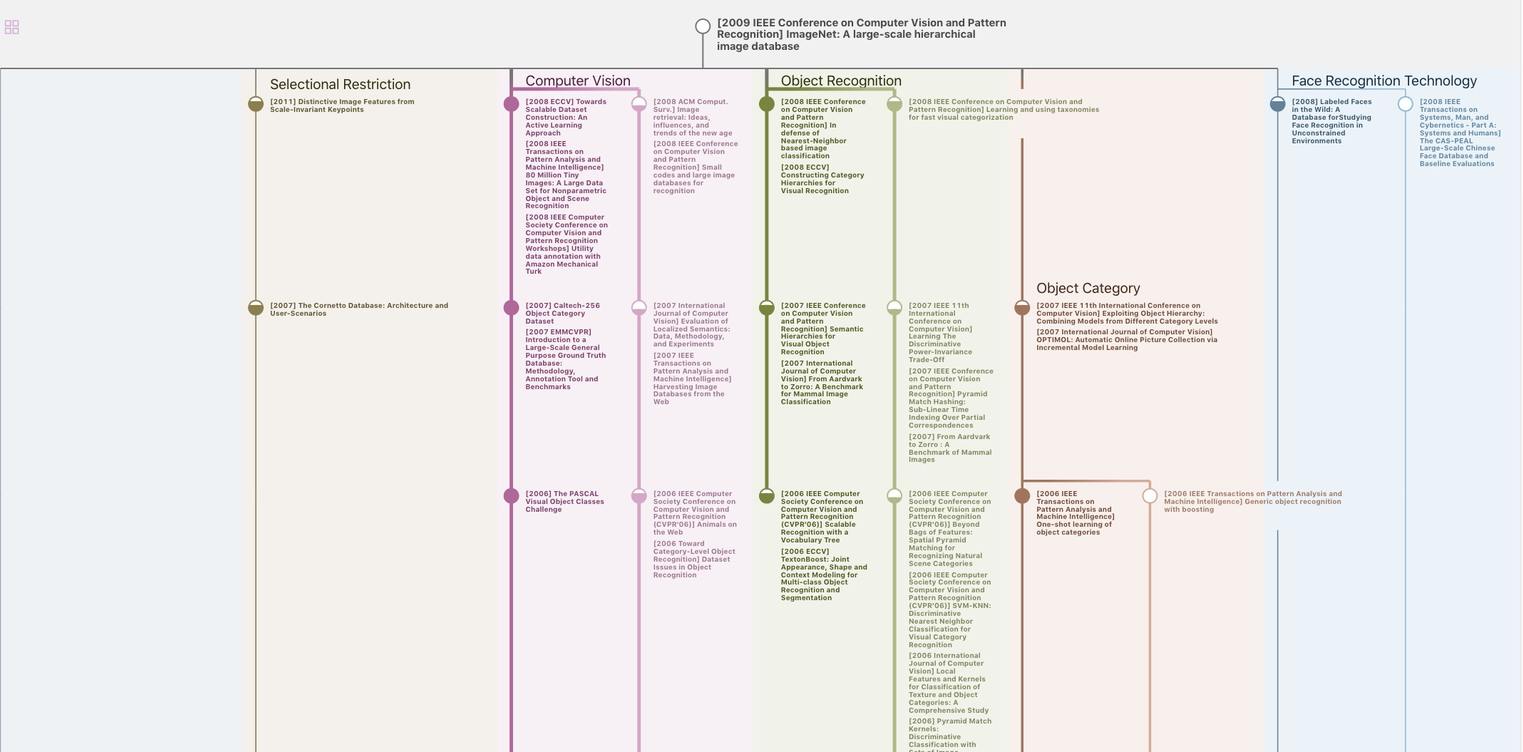
生成溯源树,研究论文发展脉络
Chat Paper
正在生成论文摘要