Online Tuning of Control Parameters for Off-Road Mobile Robots Novel Deterministic and Neural Network-Based Approaches
IEEE ROBOTICS & AUTOMATION MAGAZINE(2023)
摘要
This article addresses the problem of online adaptation of control parameters, dedicated to a path tracking problem in off-road conditions. Two approaches are offered to modify the tuning gain of a previously developed adaptive and predictive control law. The first approach is a deterministic method based on dynamic equations of the system, allowing the adaptation of the settling distance with respect to robot capabilities depending on grip conditions and velocity. The second strategy uses a neural network trained with a covariance matrix adaptation evolution strategy (CMA-ES) algorithm to optimize the robot’s behavior with respect to an objective (
obj
) function. Each approach uses as input dynamic parameters, estimated from sliding angles and cornering stiffness observers. Both methods are described and compared to results obtained when using constant parameters to identify their respective strengths and weaknesses. They have been implemented and tested in real conditions on an off-road mobile robot with varying terrain and trajectories. An in-depth analysis of the proposed techniques is done, and further insights are obtained in the context of gain tuning for steering controllers in dynamic environments. The performance and transferability of these methods are demonstrated as well as their robustness to changes in terrain properties. As a result, tracking errors are reduced while preserving the stability and explainability of the control architecture.
更多查看译文
关键词
control parameters,online tuning,off-road,network-based
AI 理解论文
溯源树
样例
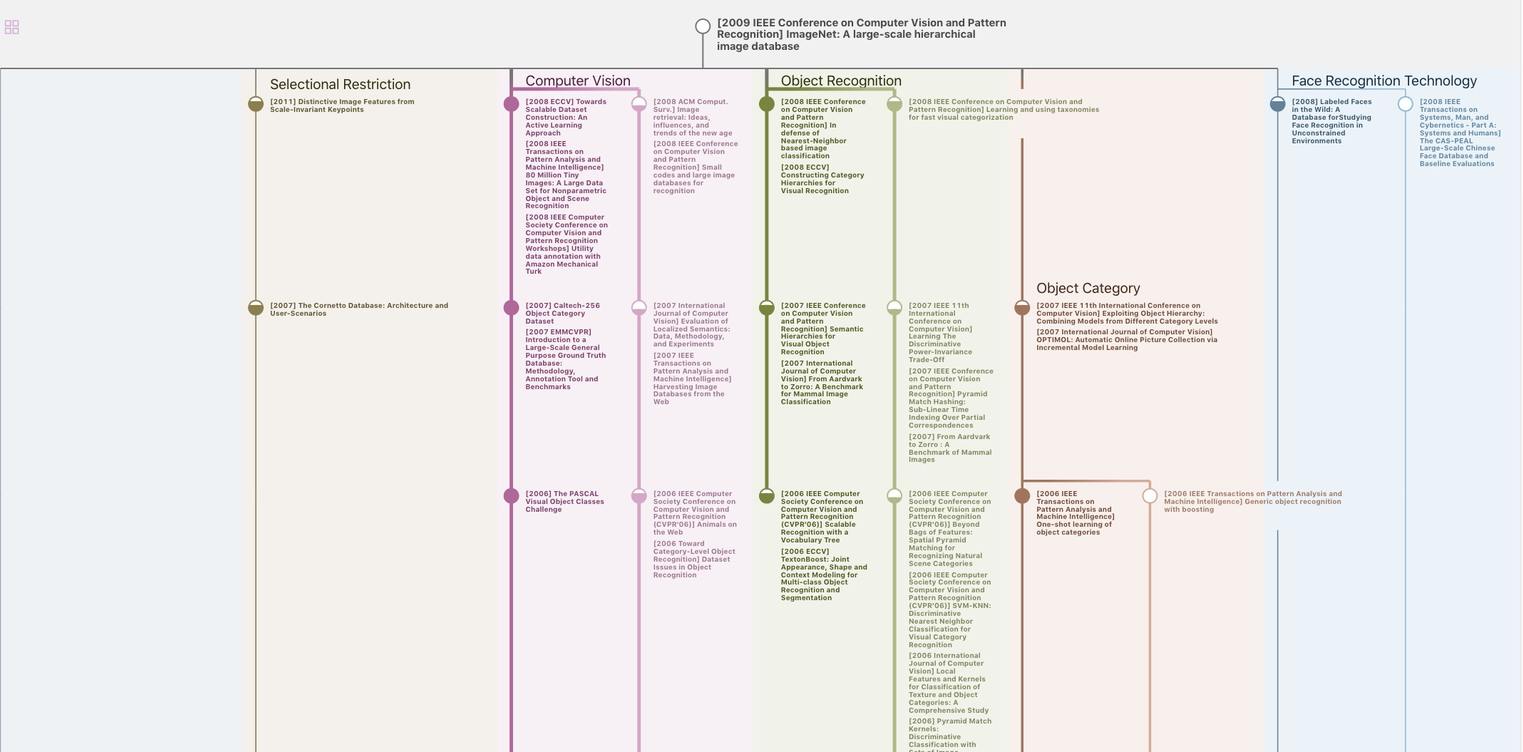
生成溯源树,研究论文发展脉络
Chat Paper
正在生成论文摘要