ContactLib-ATT: A Structure-Based Search Engine for Homologous Proteins
IEEE-ACM TRANSACTIONS ON COMPUTATIONAL BIOLOGY AND BIOINFORMATICS(2023)
摘要
General-purpose protein structure embedding can be used for many important protein biology tasks, such as protein design, drug design and binding affinity prediction. Recent researches have shown that attention-based encoder layers are more suitable to learn high-level features. Based on this key observation, we propose a two-level general-purpose protein structure embedding neural network, called ContactLib-ATT. On local embedding level, a biologically more meaningful contact context is introduced. On global embedding level, attention-based encoder layers are employed for better global representation learning. Our general-purpose protein structure embedding framework is trained and tested on the SCOP40 2.07 dataset. As a result, ContactLib-ATT achieves a SCOP superfamily classification accuracy of 82.4% (i.e., 6.7% higher than state-of-the-art method). On the same dataset, ContactLib-ATT is used to simulate a structure-based search engine for remote homologous proteins, and our top-10 candidate list contains at least one remote homolog with a probability of 91.9%.
更多查看译文
关键词
Proteins,Convolution,Hydrogen,Databases,Task analysis,Transformers,Peptides,Protein structure embedding,alignment-free homology search,hydrogen bonds,self-attention
AI 理解论文
溯源树
样例
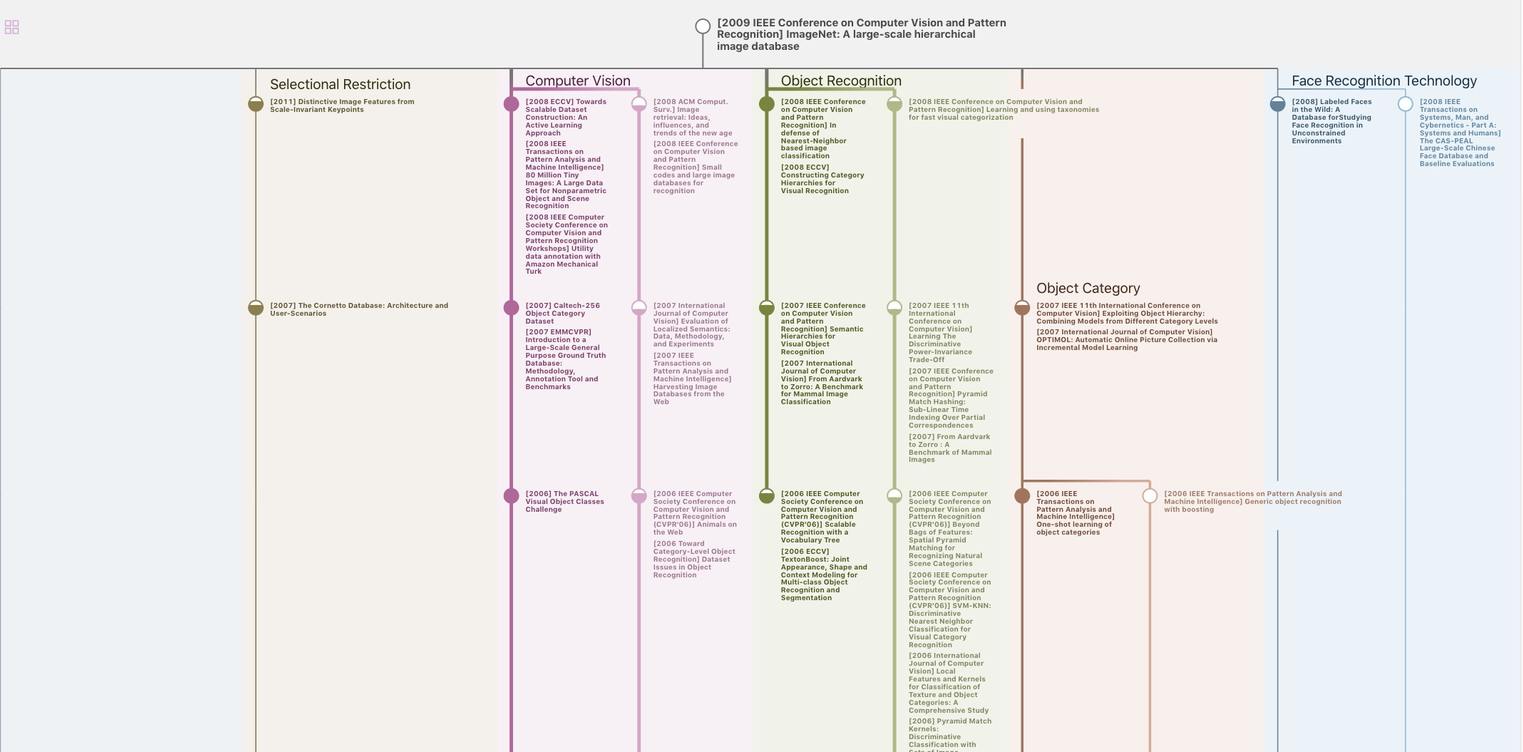
生成溯源树,研究论文发展脉络
Chat Paper
正在生成论文摘要