Behavioural specialization and learning in social networks
PROCEEDINGS OF THE ROYAL SOCIETY B-BIOLOGICAL SCIENCES(2022)
摘要
Interactions in social groups can promote behavioural specialization. One way this can happen is when individuals engage in activities with two behavioural options and learn which option to choose. We analyse interactions in groups where individuals learn from playing games with two actions and negatively frequency-dependent payoffs, such as producer-scrounger, caller-satellite, or hawk-dove games. Group members are placed in social networks, characterized by the group size and the number of neighbours to interact with, ranging from just a few neighbours to interactions between all group members. The networks we analyse include ring lattices and the much-studied small-world networks. By implementing two basic reinforcement-learning approaches, action-value learning and actor-critic learning, in different games, we find that individuals often show behavioural specialization. Specialization develops more rapidly when there are few neighbours in a network and when learning rates are high. There can be learned specialization also with many neighbours, but we show that, for action-value learning, behavioural consistency over time is higher with a smaller number of neighbours. We conclude that frequency-dependent competition for resources is a main driver of specialization. We discuss our theoretical results in relation to experimental and field observations of behavioural specialization in social situations.
更多查看译文
关键词
behavioural consistency, animal personality, reinforcement learning, game theory
AI 理解论文
溯源树
样例
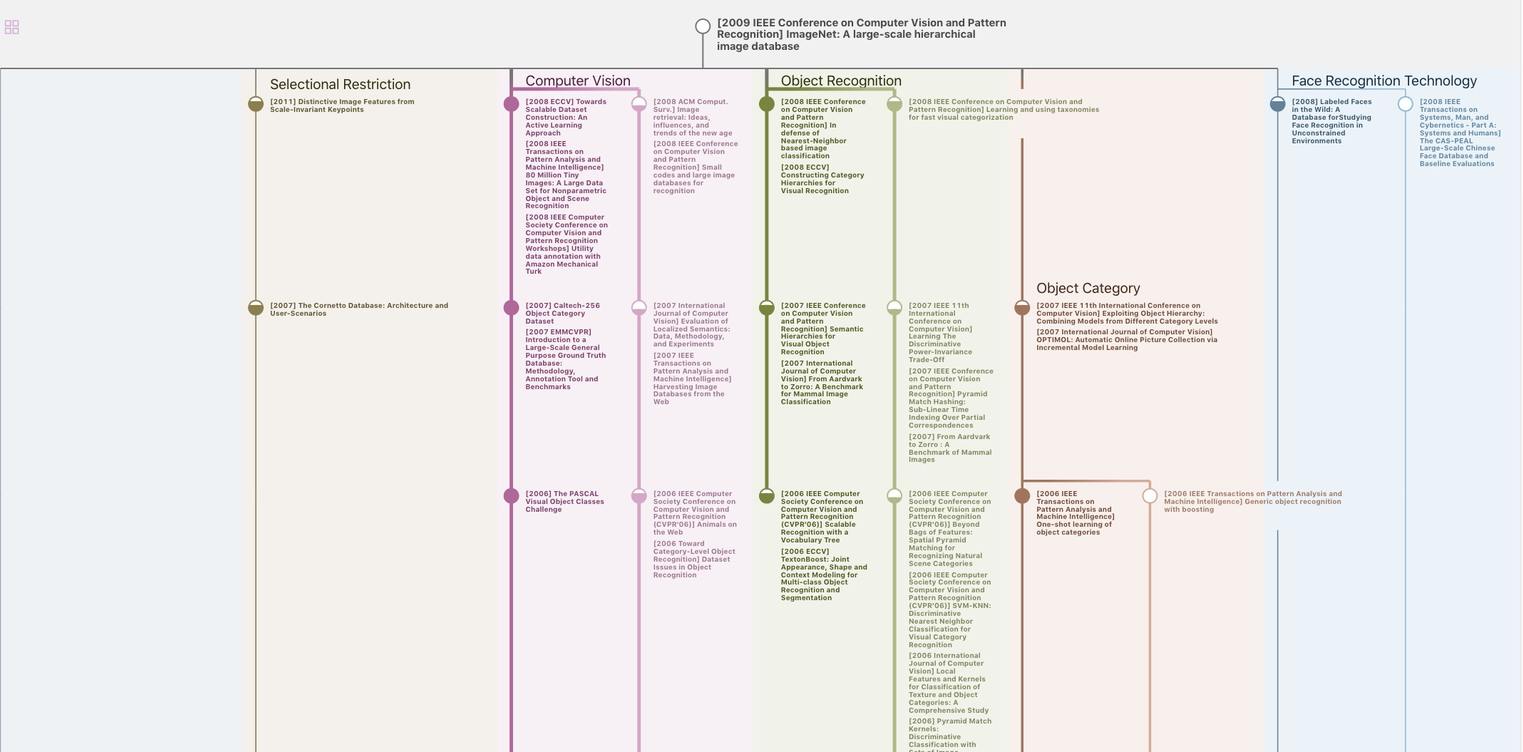
生成溯源树,研究论文发展脉络
Chat Paper
正在生成论文摘要