Fiber Optic Sensor System for Defect Classification using Novel Physics based Modelling and Data Driven Approach
2022 IEEE Aerospace Conference (AERO)(2022)
摘要
This paper discusses a new framework for Machine Health Diagnosis in the field of Structural Health Monitoring. A physics-based approach has been combined with a data-driven methodology to classify structural defects using vibration data from Fiber Bragg Grating (FBG) sensors. Three system architectures were developed comprising physics-based modeling, feature extraction via different data processing techniques and end-to-end defect classification algorithms for an in-house built bearing test-rig. A test rig consisting of a bearing-motor-shaft assembly was developed in-house for data generation and for real-time implementation of the defect detection and classification algorithms. In order to place the sensors in the right locations, a simplified Finite Element (FE) Model of the test rig was developed. Using the data from this FE model, the FBG sensors were installed on the test rig. Data processing algorithms were developed in time as well as frequency domains to extract features from the vibration data collected using these sensors. This includes developing Wavelet algorithms to generate two-dimensional spectral energy data. These features were then used with a developed Convolutional Neural Network (CNN) model which outputs the classification labels corresponding to the different types of bearing defects and defect intensities. Based on the algorithm evaluation, accuracies of 98% and 98.9% were achieved for bearing defect detection and defect intensity, respectively. As a next step, these algorithms will be implemented in an additive manufacturing environment to detect and classify part defects as they are being built.
更多查看译文
关键词
defect classification,sensor
AI 理解论文
溯源树
样例
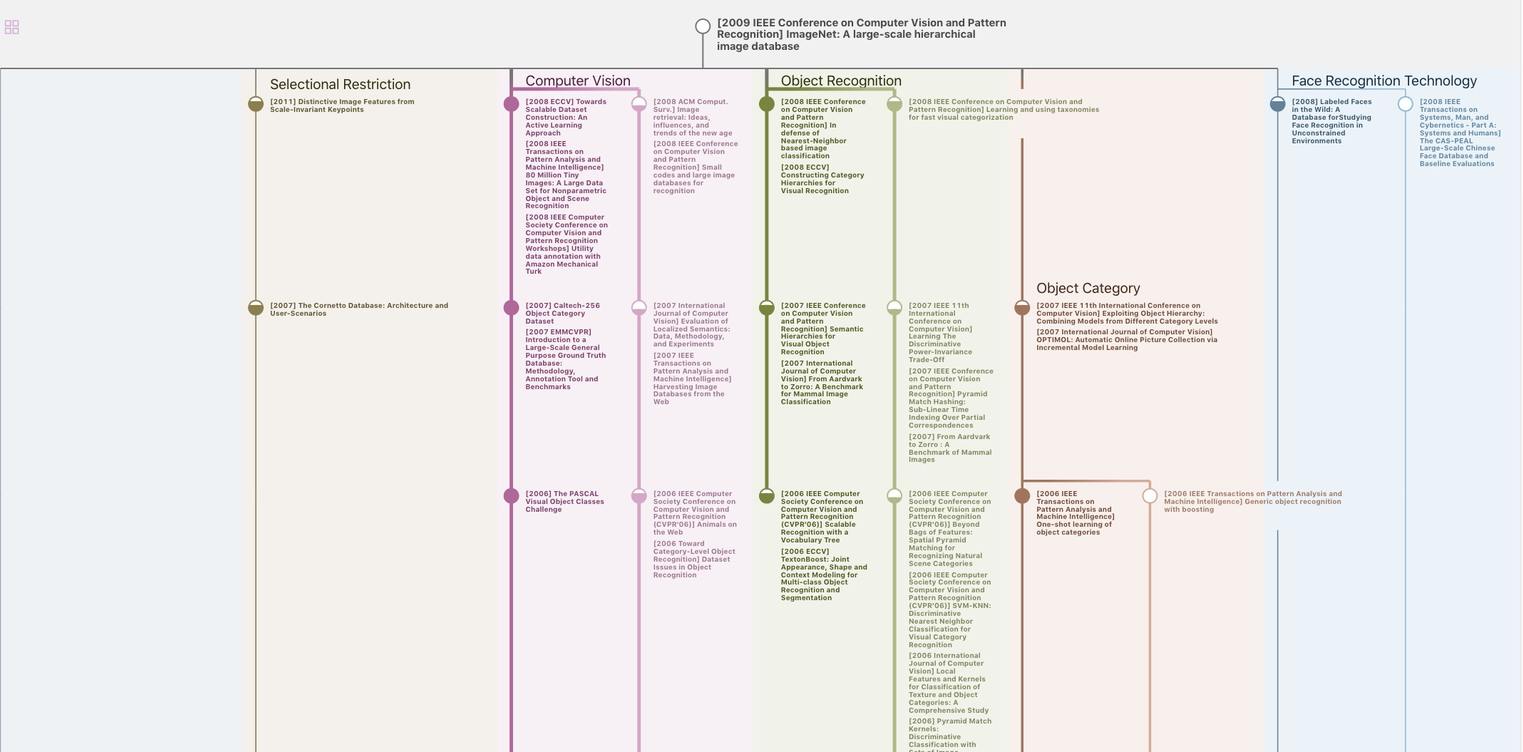
生成溯源树,研究论文发展脉络
Chat Paper
正在生成论文摘要