A Sublinear Adversarial Training Algorithm
CoRR(2022)
摘要
Adversarial training is a widely used strategy for making neural networks resistant to adversarial perturbations. For a neural network of width $m$, $n$ input training data in $d$ dimension, it takes $\\Omega(mnd)$ time cost per training iteration for the forward and backward computation. In this paper we analyze the convergence guarantee of adversarial training procedure on a two-layer neural network with shifted ReLU activation, and shows that only $o(m)$ neurons will be activated for each input data per iteration. Furthermore, we develop an algorithm for adversarial training with time cost $o(m n d)$ per iteration by applying half-space reporting data structure.
更多查看译文
关键词
training,algorithm
AI 理解论文
溯源树
样例
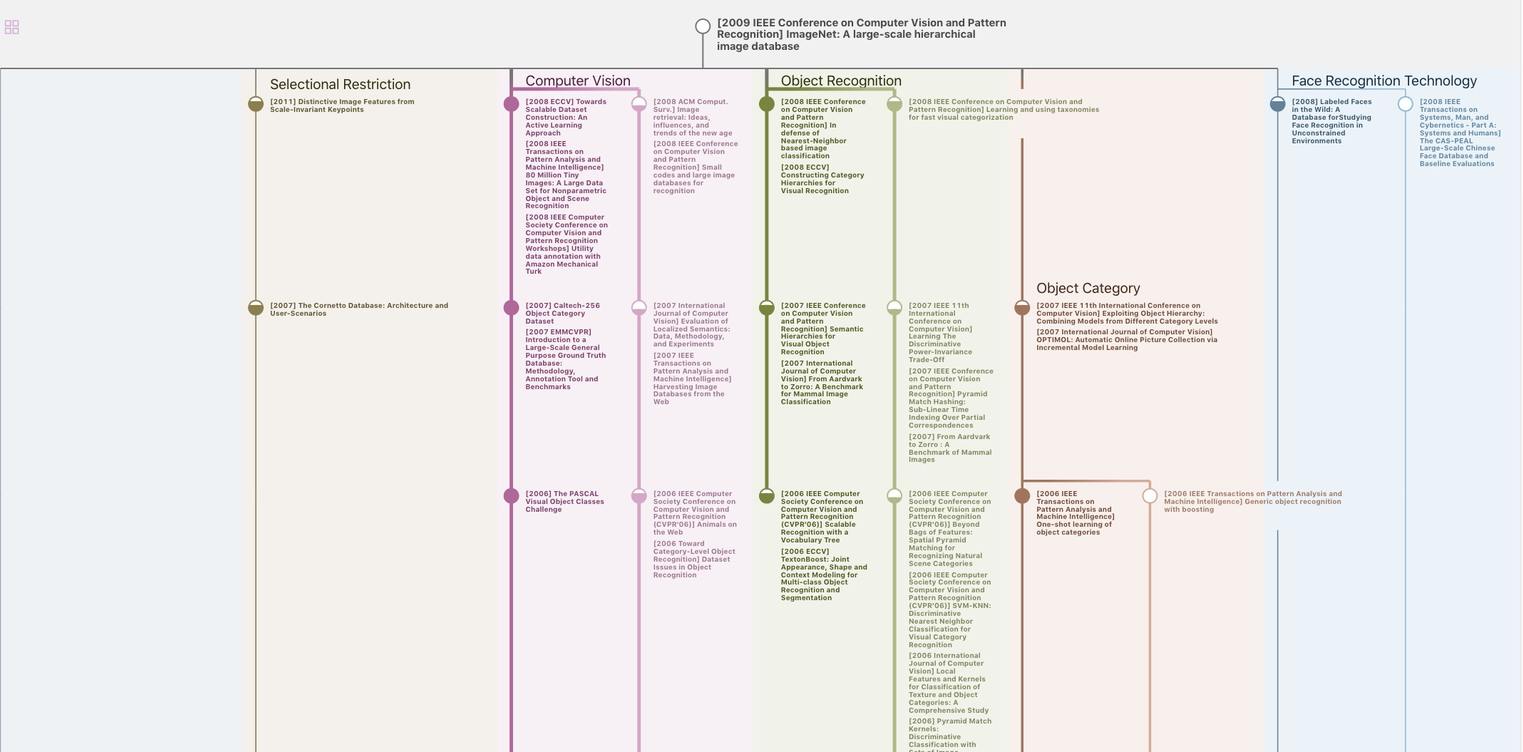
生成溯源树,研究论文发展脉络
Chat Paper
正在生成论文摘要