PEPPER: Empowering User-Centric Recommender Systems over Gossip Learning
Proceedings of the ACM on Interactive, Mobile, Wearable and Ubiquitous Technologies(2022)
摘要
ecommender systems are proving to be an invaluable tool for extracting user-relevant content helping users in their daily activities (e.g., finding relevant places to visit, content to consume, items to purchase). However, to be effective, these systems need to collect and analyze large volumes of personal data (e.g., location check-ins, movie ratings, click rates .. etc.), which exposes users to numerous privacy threats. In this context, recommender systems based on Federated Learning (FL) appear to be a promising solution for enforcing privacy as they compute accurate recommendations while keeping personal data on the users' devices. However, FL, and therefore FL-based recommender systems, rely on a central server that can experience scalability issues besides being vulnerable to attacks. To remedy this, we propose PEPPER, a decentralized recommender system based on gossip learning principles. In PEPPER, users gossip model updates and aggregate them asynchronously. At the heart of PEPPER reside two key components: a personalized peer-sampling protocol that keeps in the neighborhood of each node, a proportion of nodes that have similar interests to the former and a simple yet effective model aggregation function that builds a model that is better suited to each user. Through experiments on three real datasets implementing two use cases: a location check-in recommendation and a movie recommendation, we demonstrate that our solution converges up to 42% faster than with other decentralized solutions providing up to 9% improvement on average performance metric such as hit ratio and up to 21% improvement on long tail performance compared to decentralized competitors.
更多查看译文
关键词
Decentralized Federated Learning,Gossip Learning,model aggregation,Point-of-Interest recommendation,recommender systems
AI 理解论文
溯源树
样例
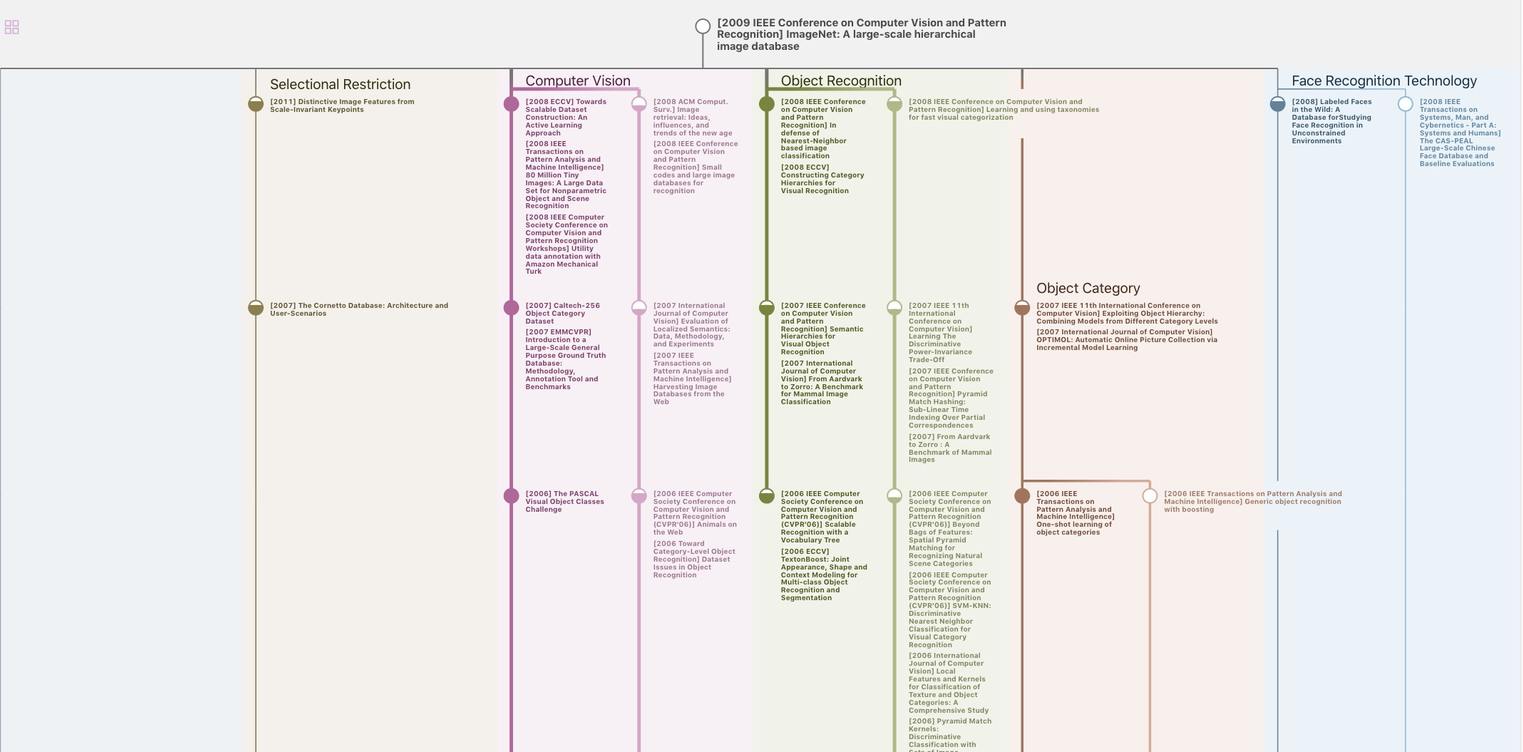
生成溯源树,研究论文发展脉络
Chat Paper
正在生成论文摘要