Exploring Point-BEV Fusion for 3D Point Cloud Object Tracking with Transformer.
IEEE transactions on pattern analysis and machine intelligence(2024)
摘要
With the prevalent use of LiDAR sensors in autonomous driving, 3D point cloud object tracking has received increasing attention. In a point cloud sequence, 3D object tracking aims to predict the location and orientation of an object in consecutive frames. Motivated by the success of transformers, we propose Point Tracking TRansformer (PTTR), which efficiently predicts high-quality 3D tracking results in a coarse-to-fine manner with the help of transformer operations. PTTR consists of three novel designs. 1) Instead of random sampling, we design Relation-Aware Sampling to preserve relevant points to the given template during subsampling. 2) We propose a Point Relation Transformer for effective feature aggregation and feature matching between the template and search region. 3) Based on the coarse tracking results, we employ a novel Prediction Refinement Module to obtain the final refined prediction through local feature pooling. In addition, motivated by the favorable properties of the Bird's-Eye View (BEV) of point clouds in capturing object motion, we further design a more advanced framework named PTTR++, which incorporates both the point-wise view and BEV representation to exploit their complementary effect in generating high-quality tracking results. PTTR++ substantially boosts the tracking performance on top of PTTR with low computational overhead. Extensive experiments over multiple datasets show that our proposed approaches achieve superior 3D tracking accuracy and efficiency. Code will be available at https://github.com/Jasonkks/PTTR.
更多查看译文
关键词
3d point-bev cloud object,tracking,fusion
AI 理解论文
溯源树
样例
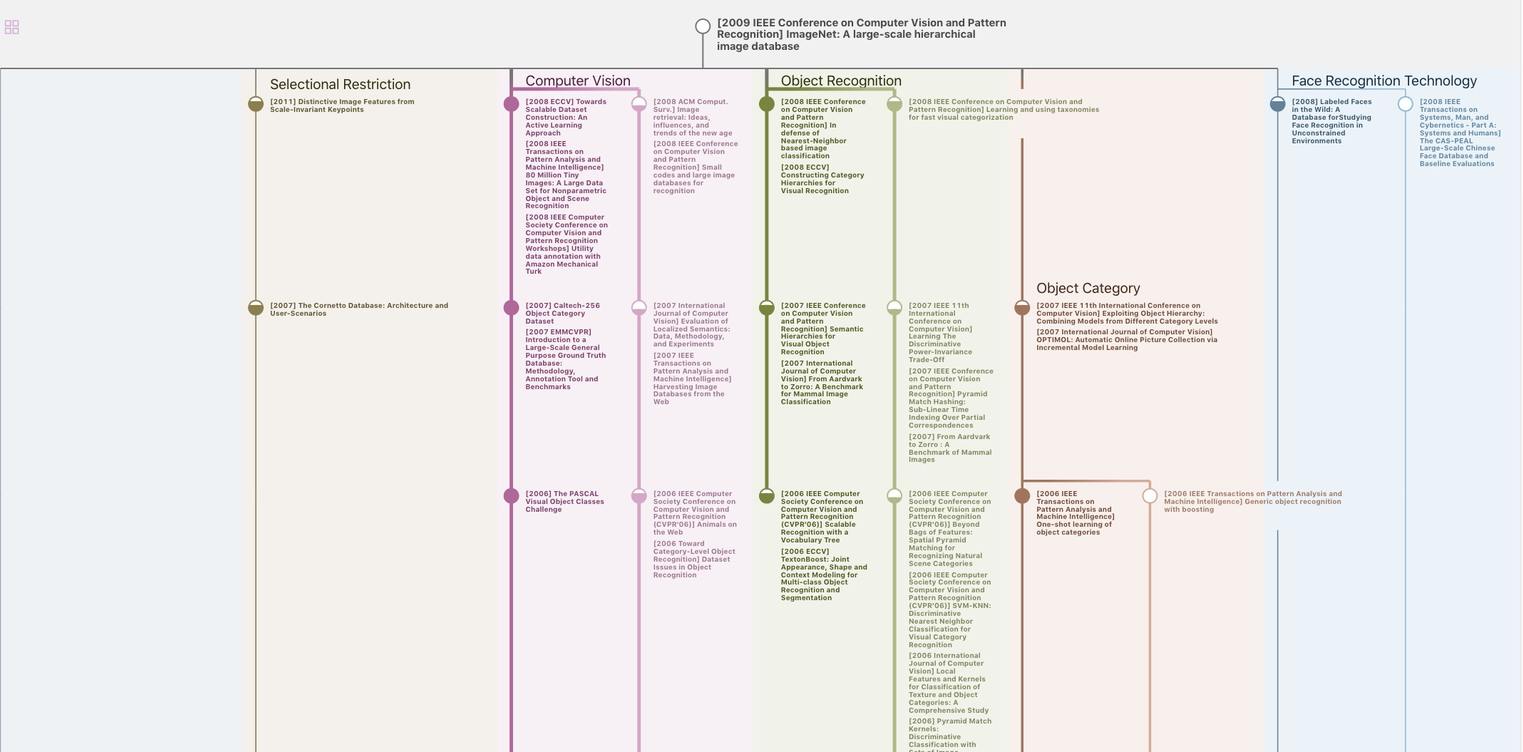
生成溯源树,研究论文发展脉络
Chat Paper
正在生成论文摘要