Digital computing through randomness and order in neural networks
PROCEEDINGS OF THE NATIONAL ACADEMY OF SCIENCES OF THE UNITED STATES OF AMERICA(2022)
摘要
We propose that coding and decoding in the brain are achieved through digital computation using three principles: relative ordinal coding of inputs, random connections between neurons, and belief voting. Due to randomization and despite the coarseness of the relative codes, we show that these principles are sufficient for coding and decoding sequences with error-free reconstruction. In particular, the number of neurons needed grows linearly with the size of the input repertoire growing exponentially. We illustrate our model by reconstructing sequences with repertoires on the order of a billion items. From this, we derive the Shannon equations for the capacity limit to learn and transfer information in the neural population, which is then generalized to any type of neural network. Following the maximum entropy principle of efficient coding, we show that random connections serve to decorrelate redundant information in incoming signals, creating more compact codes for neurons and therefore, conveying a larger amount of information. Henceforth, despite the unreliability of the relative codes, few neurons become necessary to discriminate the original signal without error. Finally, we discuss the significance of this digital computation model regarding neurobiological findings in the brain and more generally with artificial intelligence algorithms, with a view toward a neural information theory and the design of digital neural networks.
更多查看译文
关键词
sparse coding,digital computing,maximum entropy,continual learning,catastrophic forgetting
AI 理解论文
溯源树
样例
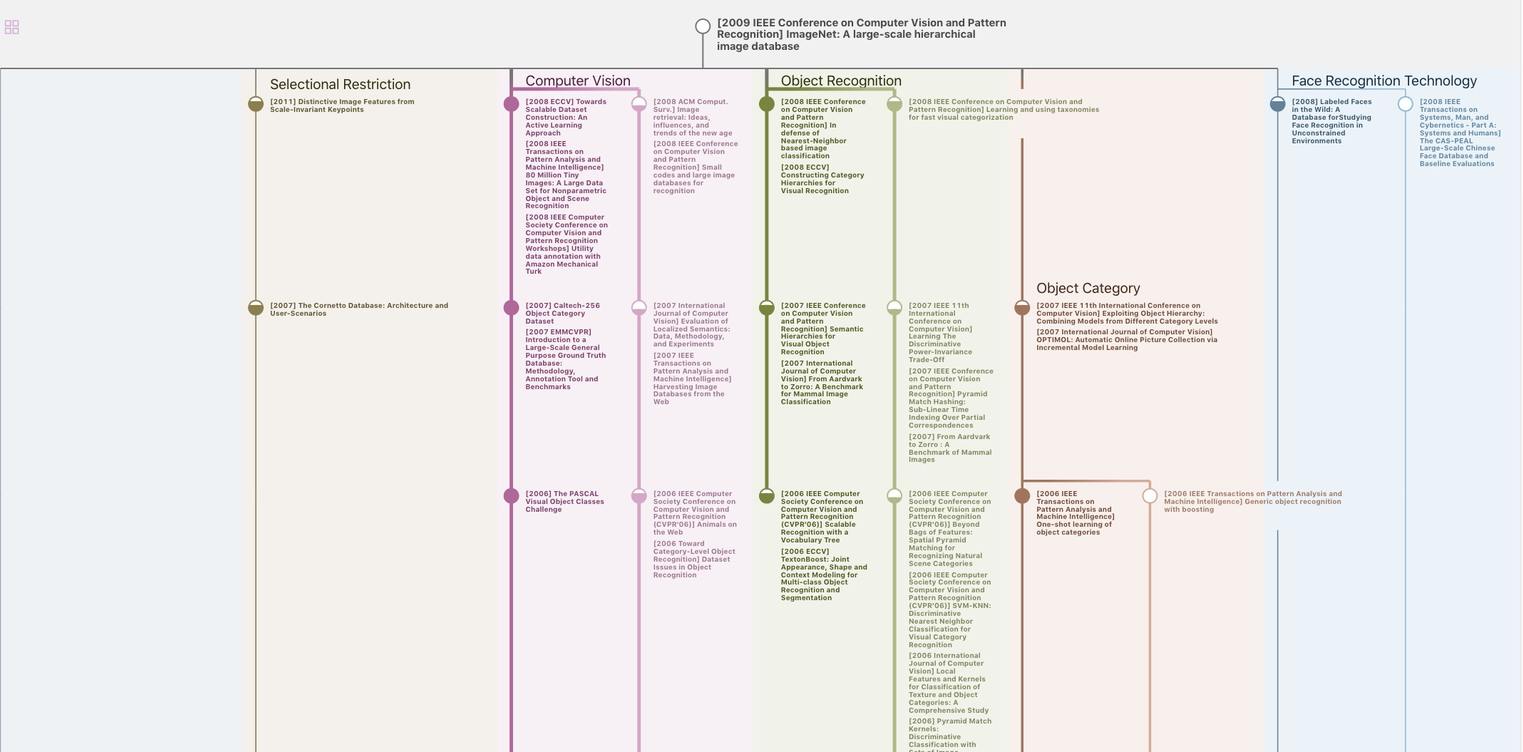
生成溯源树,研究论文发展脉络
Chat Paper
正在生成论文摘要