Developing and validating a chronic obstructive pulmonary disease quick screening questionnaire using statistical learning models
CHRONIC RESPIRATORY DISEASE(2022)
摘要
Background Active targeted case-finding is a cost-effective way to identify individuals with high-risk for early diagnosis and interventions of chronic obstructive pulmonary disease (COPD). A precise and practical COPD screening instrument is needed in health care settings. Methods We created four statistical learning models to predict the risk of COPD using a multi-center randomized cross-sectional survey database (n = 5281). The minimal set of predictors and the best statistical learning model in identifying individuals with airway obstruction were selected to construct a new case-finding questionnaire. We validated its performance in a prospective cohort (n = 958) and compared it with three previously reported case-finding instruments. Results A set of seven predictors was selected from 643 variables, including age, morning productive cough, wheeze, years of smoking cessation, gender, job, and pack-year of smoking. In four statistical learning models, generalized additive model model had the highest area under curve (AUC) value both on the developing cross-sectional data set (AUC = 0.813) and the prospective validation data set (AUC = 0.880). Our questionnaire outperforms the other three tools on the cross-sectional validation data set. Conclusions We developed a COPD case-finding questionnaire, which is an efficient and cost-effective tool for identifying high-risk population of COPD.
更多查看译文
关键词
chronic obstructive pulmonary disease, machine learning, generalized additive model, screening, smoking
AI 理解论文
溯源树
样例
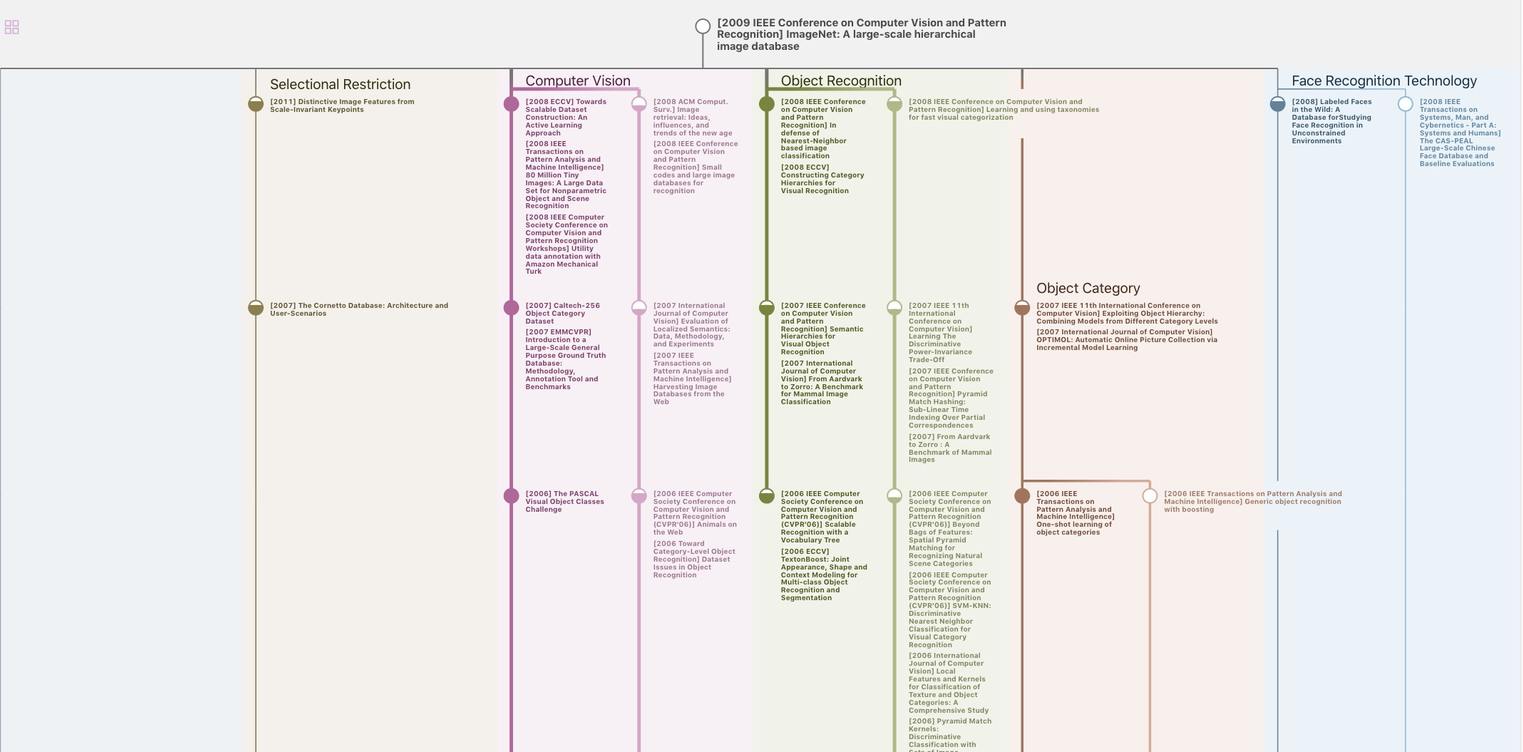
生成溯源树,研究论文发展脉络
Chat Paper
正在生成论文摘要