Weighted Fuzzy Clustering for Time Series With Trend-Based Information Granulation
IEEE TRANSACTIONS ON CYBERNETICS(2024)
摘要
The highly dimensional characteristic of time series brings many challenges on direct mining time series, such as high cost in time and space. Granular computing provides a potential strategy for representing and dealing with time series at a higher level of abstraction. In this study, we propose an information granulation-based weighted fuzzy C-means (wFCM) method to realize time-series clustering, which could avoid high dimensionality processing and provide a concise and visible granular prototype for each cluster. In this method, each time series is first transformed into a series of information granules with trend following the principle of justifiable granularity. The formed granular time series can well capture the main features lying in the original time series and help realize dimensionality reduction. Then, the wFCM method is developed to complete time-series clustering in the granular space. Here, the dynamic time warping (DTW) is extended to capture the similarity for trend-based granular time series. Furthermore, the weighted DTW barycenter averaging is introduced to derive prototypes presented in a granular format, capturing the level, the fluctuation, and the changing trend, which are meaningful and understandable clustering results. The experiments conducted on real-world datasets coming from the UCR time-series database and Chinese stocks are presented to illustrate the effectiveness and practicality of the designed time-series clustering model.
更多查看译文
关键词
Time series analysis,Market research,Prototypes,Clustering algorithms,Time measurement,Optimization,Dimensionality reduction,Dynamic time warping (DTW),granular prototype,time series,trend-based information granulation (TIG),weighted fuzzy clustering
AI 理解论文
溯源树
样例
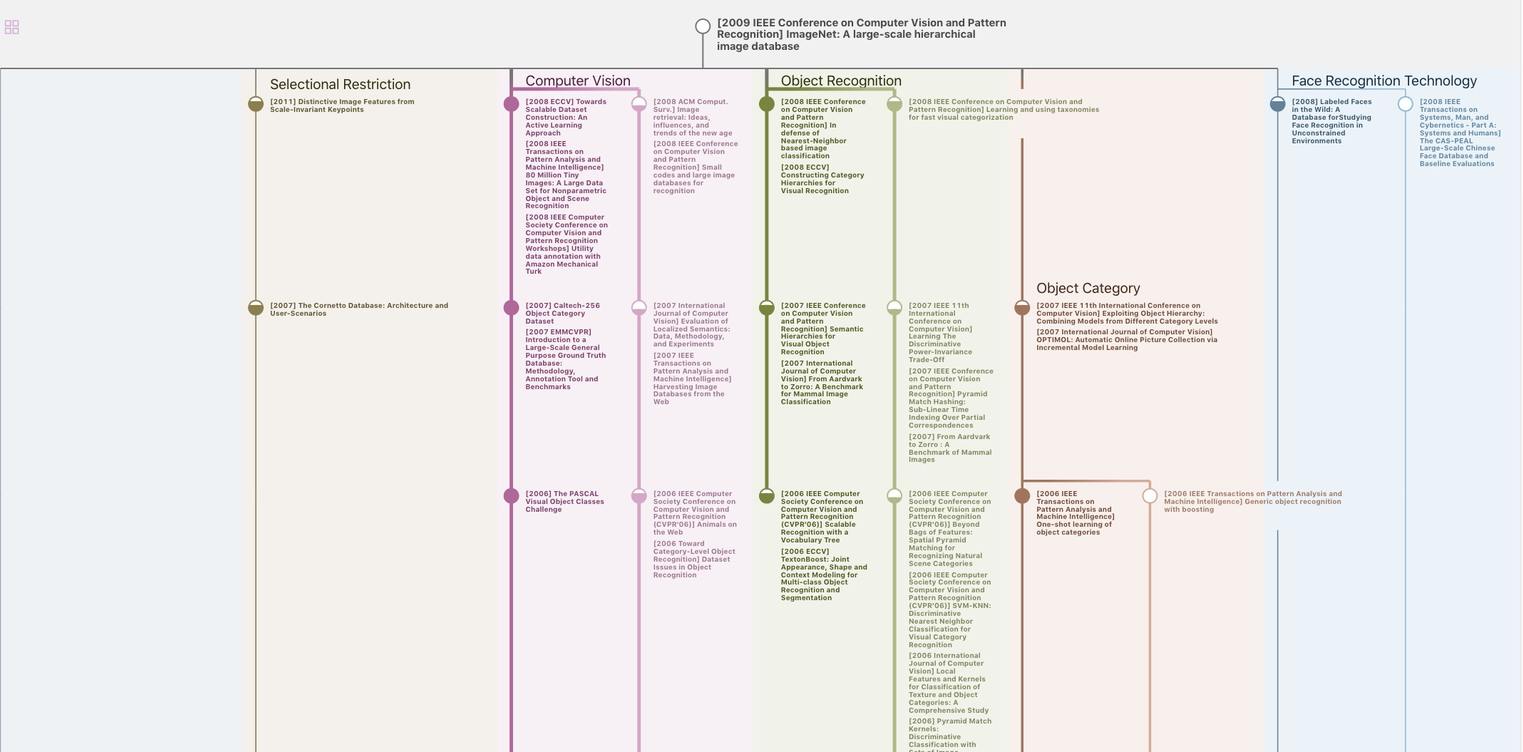
生成溯源树,研究论文发展脉络
Chat Paper
正在生成论文摘要