Machine learning classification of multiple sclerosis in children using optical coherence tomography
MULTIPLE SCLEROSIS JOURNAL(2022)
摘要
Background: In children, multiple sclerosis (MS) is the ultimate diagnosis in only 1/5 to 1/3 of cases after a first episode of central nervous system (CNS) demyelination. As the visual pathway is frequently affected in MS and other CNS demyelinating disorders (DDs), structural retinal imaging such as optical coherence tomography (OCT) can be used to differentiate MS. Objective: This study aimed to investigate the utility of machine learning (ML) based on OCT features to identify distinct structural retinal features in children with DDs. Methods: This study included 512 eyes from 187 (n(eyes) = 374) children with demyelinating diseases and 69 (n(eyes) = 138) controls. Input features of the analysis comprised of 24 auto-segmented OCT features. Results: Random Forest classifier with recursive feature elimination yielded the highest predictive values and identified DDs with 75% and MS with 80% accuracy, while multiclass distinction between MS and monophasic DD was performed with 64% accuracy. A set of eight retinal features were identified as the most important features in this classification. Conclusion: This study demonstrates that ML based on OCT features can be used to support a diagnosis of MS in children.
更多查看译文
关键词
Multiple sclerosis,pediatric,optical coherence tomography,supervised learning,retinal nerve fiber layer thickness
AI 理解论文
溯源树
样例
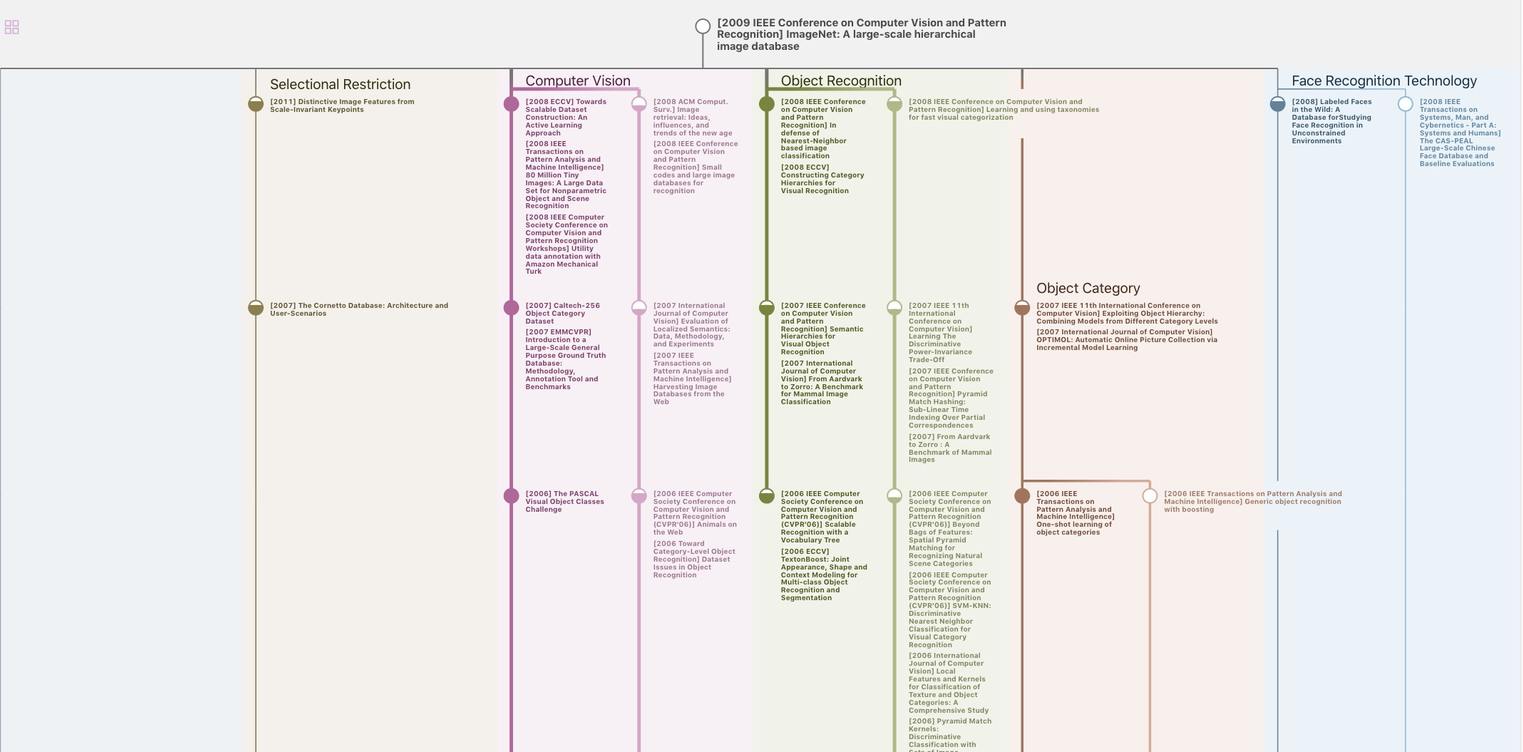
生成溯源树,研究论文发展脉络
Chat Paper
正在生成论文摘要