A Relevant, Hard and Diverse Triplet Sampling Method for Multi-Label Remote Sensing Image Retrieval
2022 IEEE Mediterranean and Middle-East Geoscience and Remote Sensing Symposium (M2GARSS)(2022)
摘要
Deep metric learning based methods that characterize the semantic similarity of images on an embedding (i.e., metric) space have attracted great attention for content-based image retrieval (CBIR) in remote sensing (RS). One of the common strategies to learn a metric space is based on the selection of triplets of similar (positive) and dissimilar (negative) images for a given anchor image. The accurate selection of image triplets is a difficult task. This is more evident when each training image is annotated by multiple class labels (i.e., multi-labels). To address this problem, in this paper, we propose a novel deep learning based triplet sampling method to select a small set of the most representative and informative triplets for multi-label RS CBIR problems. The proposed method consists of two main steps. In the first step, the proposed method selects a small set of anchors (which are diverse to each other in the embedding space) from each training mini-batch by an iterative algorithm. In the second step, the sets of positive and negative images are selected for each anchor based on the relevancy, hardness and diversity of the images among each other by a novel strategy. Experimental results show the effectiveness of the proposed method on CBIR problems for multi-label RS images.
更多查看译文
关键词
Multi-label image retrieval,triplet selection,remote sensing
AI 理解论文
溯源树
样例
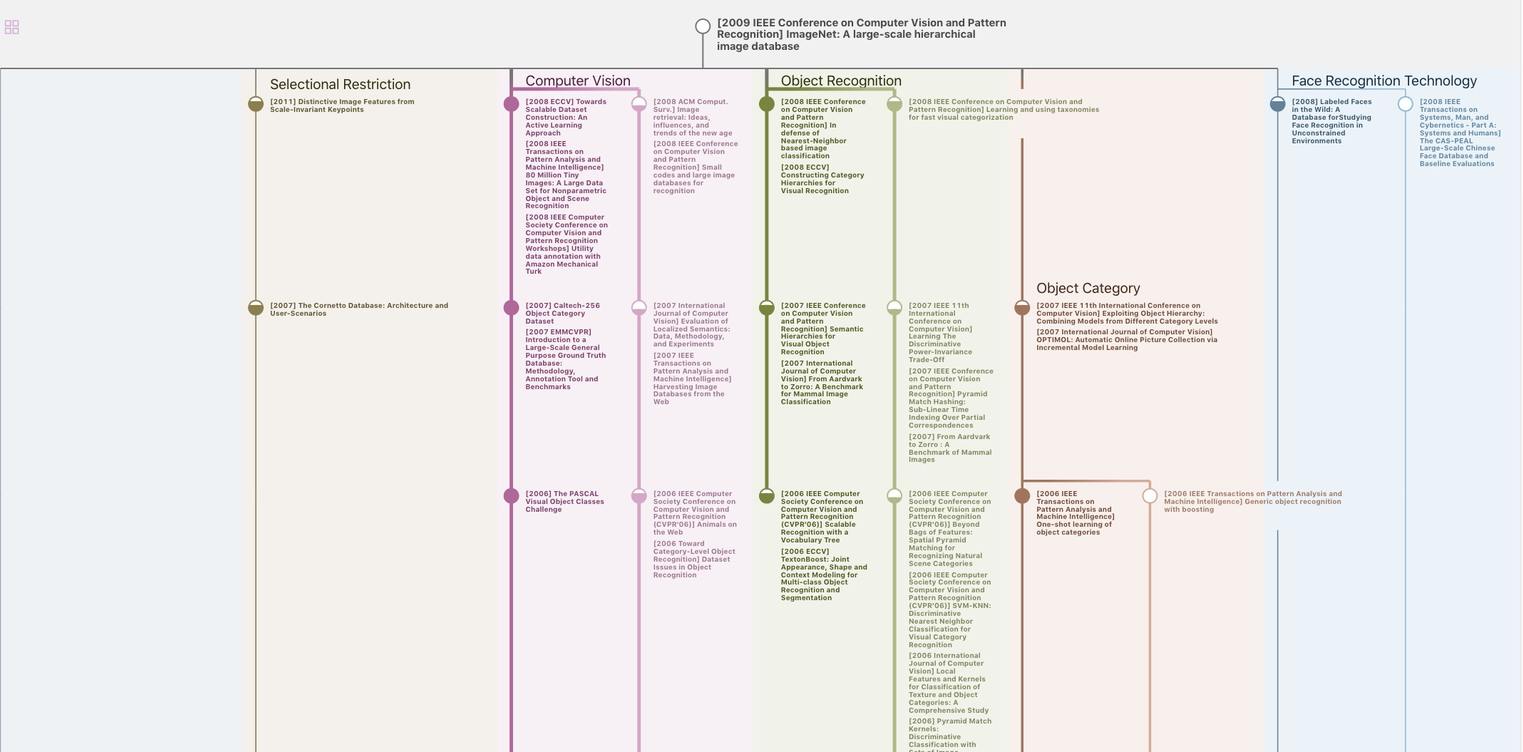
生成溯源树,研究论文发展脉络
Chat Paper
正在生成论文摘要