QSAM-Net: Rain Streak Removal by Quaternion Neural Network With Self-Attention Module
IEEE TRANSACTIONS ON MULTIMEDIA(2024)
摘要
Real-world images captured in remote sensing, image or video retrieval, and outdoor surveillance are often degraded due to poor weather conditions, such as rain and mist. These conditions introduce artifacts that make visual analysis challenging and limit the performance of high-level computer vision methods. In time-critical applications, it is vital to develop algorithms that automatically remove rain without compromising the quality of the image contents. This article proposes a novel approach called QSAM-Net, a quaternion multi-stage multiscale neural network with a self-attention module. The algorithm requires significantly fewer parameters by a factor of 3.98 than the real-valued counterpart and state-of-the-art methods while improving the visual quality of the images. The extensive evaluation and benchmarking on synthetic and real-world rainy images demonstrate the effectiveness of QSAM-Net. This feature makes the network suitable for edge devices and applications requiring near real-time performance. Furthermore, the experiments show that the improved visual quality of images also leads to better object detection accuracy and training speed.
更多查看译文
关键词
Deep learning,object detection,quaternion image processing,quaternion neural networks,rain removal
AI 理解论文
溯源树
样例
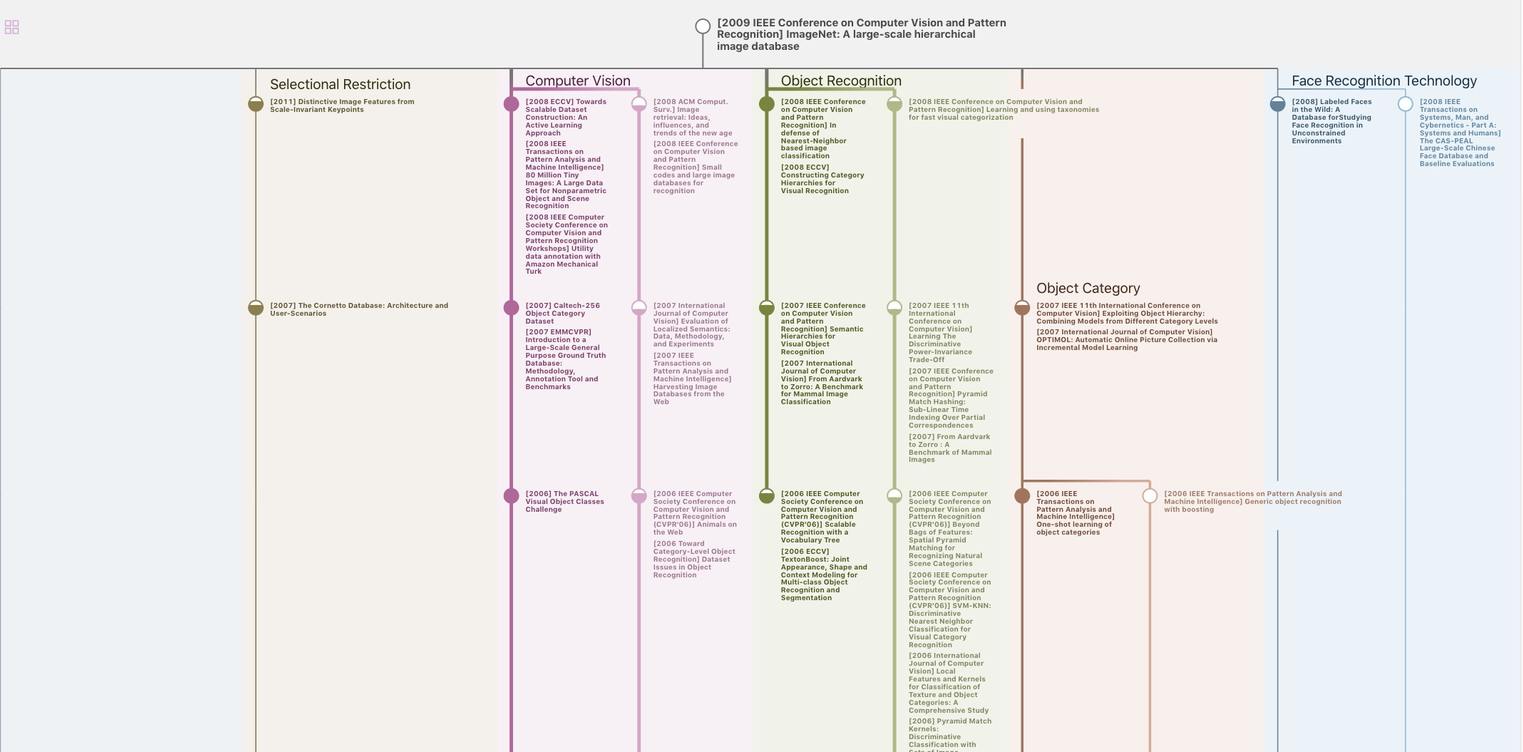
生成溯源树,研究论文发展脉络
Chat Paper
正在生成论文摘要