Association Between Neighborhood Factors and Adult Obesity in Shelby County, Tennessee: Geospatial Machine Learning
JMIR public health and surveillance(2022)
摘要
Background: Obesity is a global epidemic causing at least 2.8 million deaths per year. This complex disease is associated with significant socioeconomic burden, reduced work productivity, unemployment, and other social determinants of health (SDOH) disparities. Objective: The objective of this study was to investigate the effects of SDOH on obesity prevalence among adults in Shelby County, Tennessee, the United States, using a geospatial machine learning approach. Methods: Obesity prevalence was obtained from the publicly available 500 Cities database of Centers for Disease Control and Prevention, and SDOH indicators were extracted from the US census and the US Department of Agriculture. We examined the geographic distributions of obesity prevalence patterns, using Getis-Ord Gi* statistics and calibrated multiple models to study the association between SDOH and adult obesity. Unsupervised machine learning was used to conduct grouping analysis to investigate the distribution of obesity prevalence and associated SDOH indicators. Results: Results depicted a high percentage of neighborhoods experiencing high adult obesity prevalence within Shelby County. In the census tract, the median household income, as well as the percentage of individuals who were Black, home renters, living below the poverty level, 55 years or older, unmarried, and uninsured, had a significant association with adult obesity prevalence. The grouping analysis revealed disparities in obesity prevalence among disadvantaged neighborhoods. Conclusions: More research is needed to examine links between geographical location, SDOH, and chronic diseases. The findings of this study, which depict a significantly higher prevalence of obesity within disadvantaged neighborhoods, and other geospatial information can be leveraged to offer valuable insights, informing health decision-making and interventions that mitigate risk factors of increasing obesity prevalence.
更多查看译文
关键词
obesity,obesity surveillance,disease surveillance,machine learning,geographic information systems,social determinants of health,SDOH,disparities
AI 理解论文
溯源树
样例
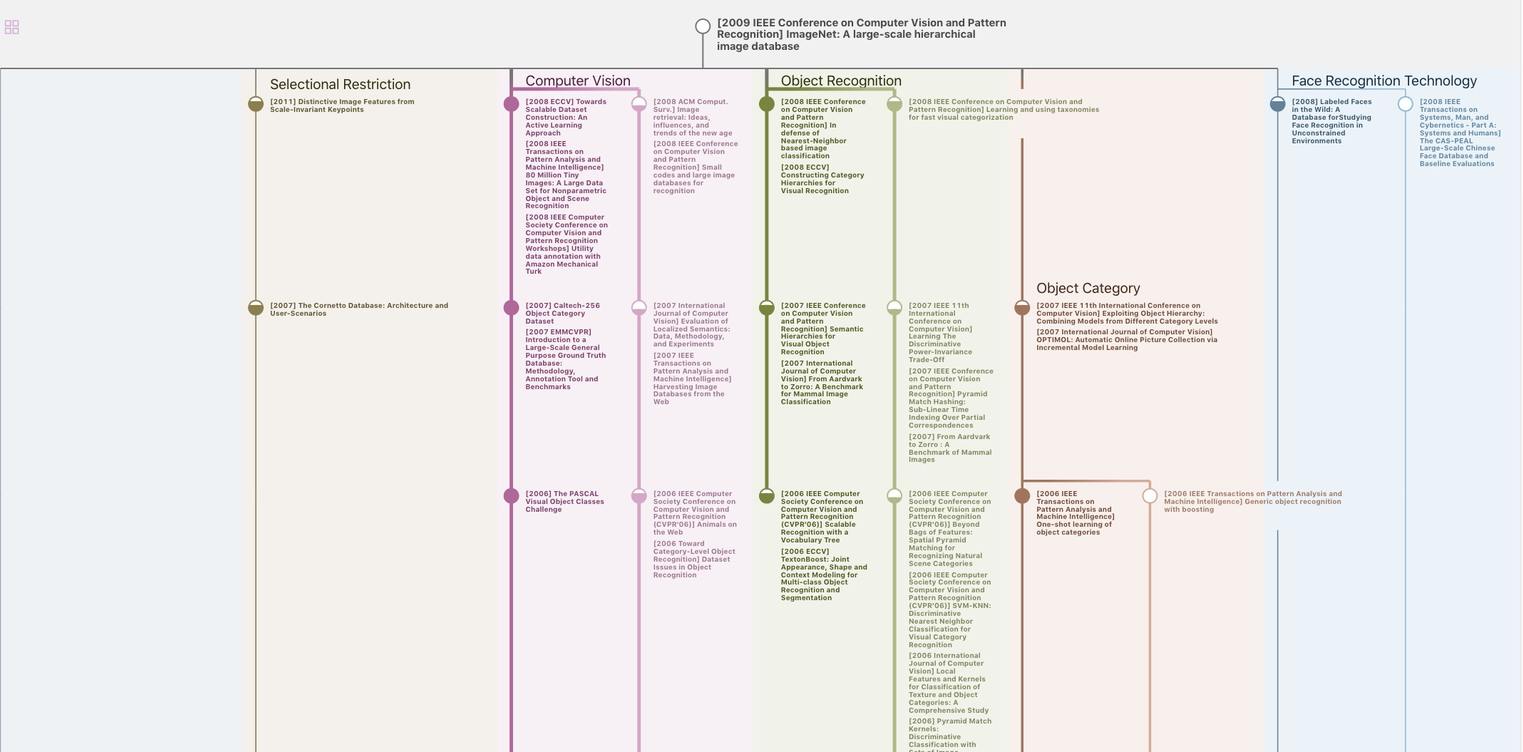
生成溯源树,研究论文发展脉络
Chat Paper
正在生成论文摘要