A Hybrid Incentive Mechanism for Decentralized Federated Learning
Distributed Ledger Technologies: Research and Practice(2022)
摘要
Federated Learning (FL) presents a privacy-compliant approach by sharing model parameters instead of raw data. However, how to motivate data owners to participate in and stay within an FL ecosystem by continuously contributing their data to the FL model remains a challenge. In this article, we propose a hybrid incentive mechanism based on blockchain to address the above challenge. The proposed mechanism comprises two primary smart contract-based modules, namely the reputation module and the reverse auction module. The former is used to dynamically calculate the reputation score of each FL participant. It employs a trust-jointed reputation scheme to balance the weights between trust values of parameters and bid prices. The latter is responsible for initiating FL auction tasks, calculating price rankings, and assigning corresponding token rewards. Experiments are conducted to evaluate the feasibility and performance of the proposed mechanism against the three typical threats. Experimental results indicate that our mechanism can successfully reduce incentive costs while preventing participants from colluding and over-bidding in the data sharing auction.
更多查看译文
关键词
hybrid incentive mechanism,learning
AI 理解论文
溯源树
样例
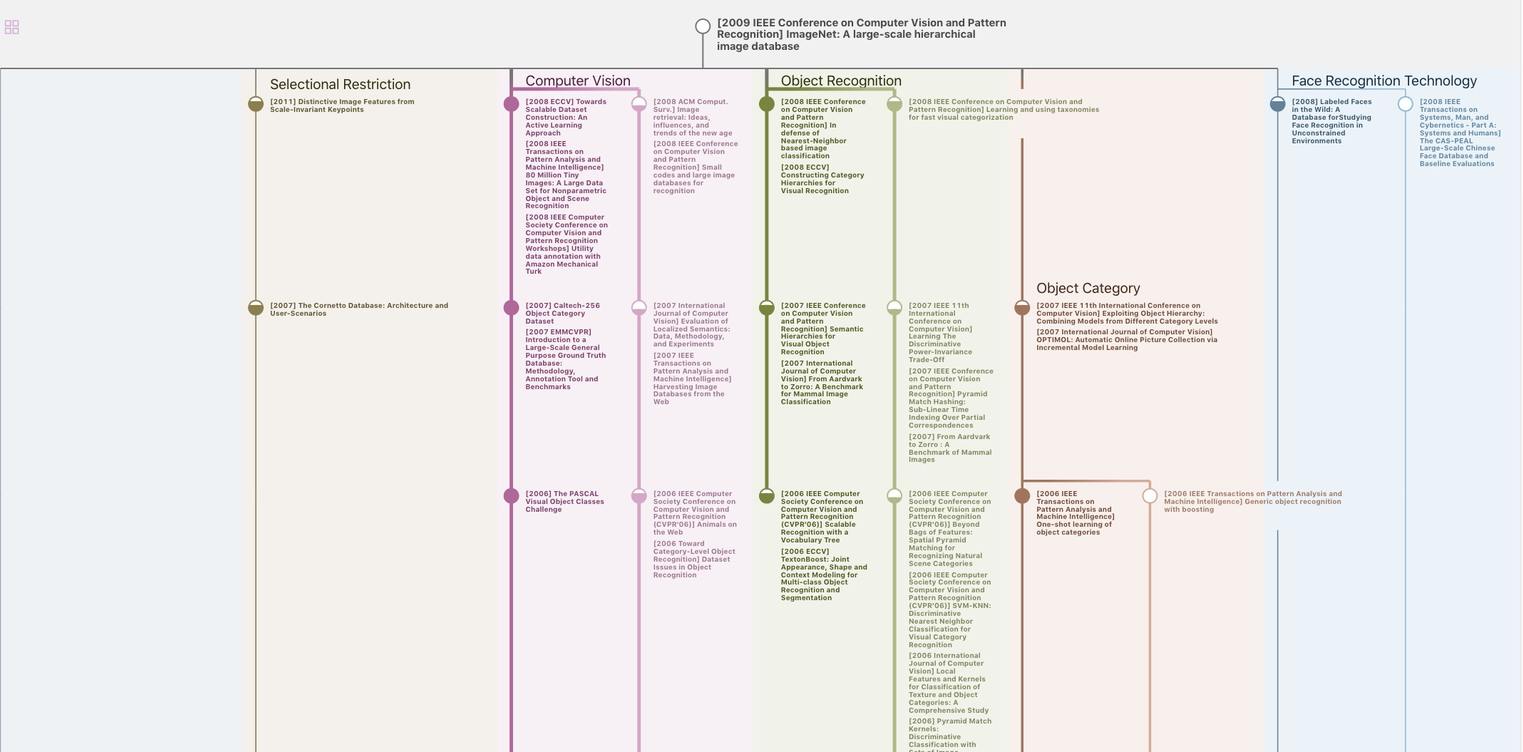
生成溯源树,研究论文发展脉络
Chat Paper
正在生成论文摘要