A Deep Reinforcement Learning based Intrusion Detection Strategy for Smart Vehicular Networks
IEEE INFOCOM 2022 - IEEE CONFERENCE ON COMPUTER COMMUNICATIONS WORKSHOPS (INFOCOM WKSHPS)(2022)
摘要
Smart vehicular network (SVN) intellectualizes traditional transportation network, significantly enhancing traffic convenience and safety. However, high connectivity and massive devices bring more vulnerabilities, which severely compromise the security, privacy, and trust of the facilities and data. To address the ever-increasing security threats in SVN, we introduce an intrusion detection system to distinguish the abnormal traffic or behavior. A Deep Reinforcement Learning (DRL)-based intrusion detection strategy is proposed in this paper to optimize the detection performance. We exploit a modified Dueling DQN (Deep Q Learning) model, in which interaction between agent and environment is transformed into a supervised machine learning task. Through action taking and reward feedback, the Dueling DQN model can be trained to learn the intrinsic features of traffic data. Finally, simulation result on benchmark intrusion detection dataset also verifies the feasibility and effectiveness of the proposed strategy.
更多查看译文
关键词
deep reinforcement learning, intrusion detection, deep q network, network security
AI 理解论文
溯源树
样例
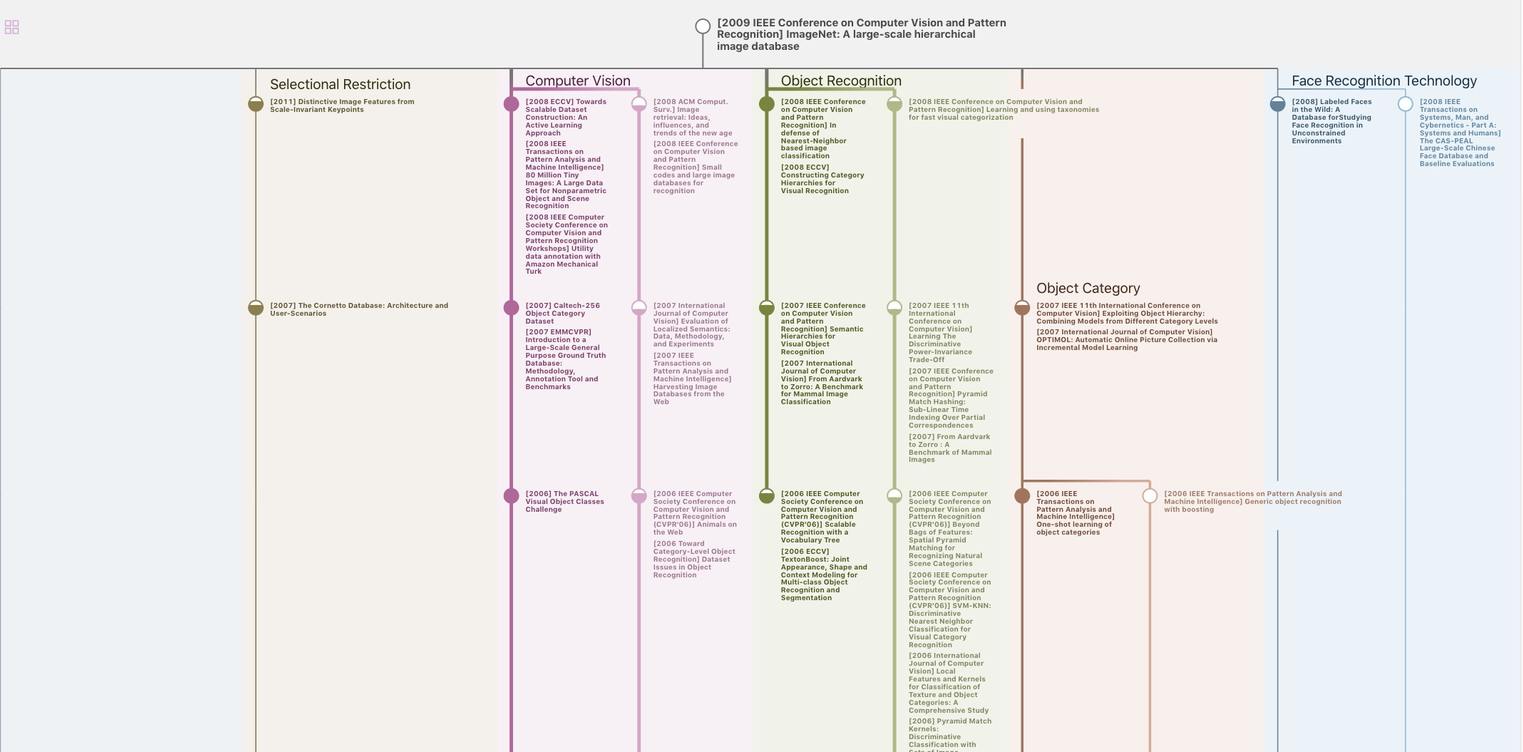
生成溯源树,研究论文发展脉络
Chat Paper
正在生成论文摘要