Mitigating Malicious Adversaries Evasion Attacks in Industrial Internet of Things
IEEE Transactions on Industrial Informatics(2023)
摘要
With advanced 5G/6G networks, data-driven interconnected devices will increase exponentially. As a result, the Industrial Internet of Things (IIoT) requires data secure information extraction to apply digital services, medical diagnoses, and financial forecasting. This introduction of high-speed network mobile applications will also adapt. As a consequence, the scale and complexity of Android malware are rising. Detection of malware classification is vulnerable to attacks. A fabricated feature can force misclassification to produce the desired output. This article proposes a subset feature selection method to evade fabricated attacks in the IIoT environment. The method extracts application-aware features from a single android application to train an independent classification model. Ensemble-based learning is then used to train the distinct classification models. Finally, the collaborative ML classifier makes independent decisions to fight against adversarial evasion attacks. We compare and evaluate the benchmark Android malware dataset. The proposed method achieved 91% accuracy with 14 fabricated input features.
更多查看译文
关键词
Adversarial attacks,Android,Industrial Internet of Things (IIoT),malware
AI 理解论文
溯源树
样例
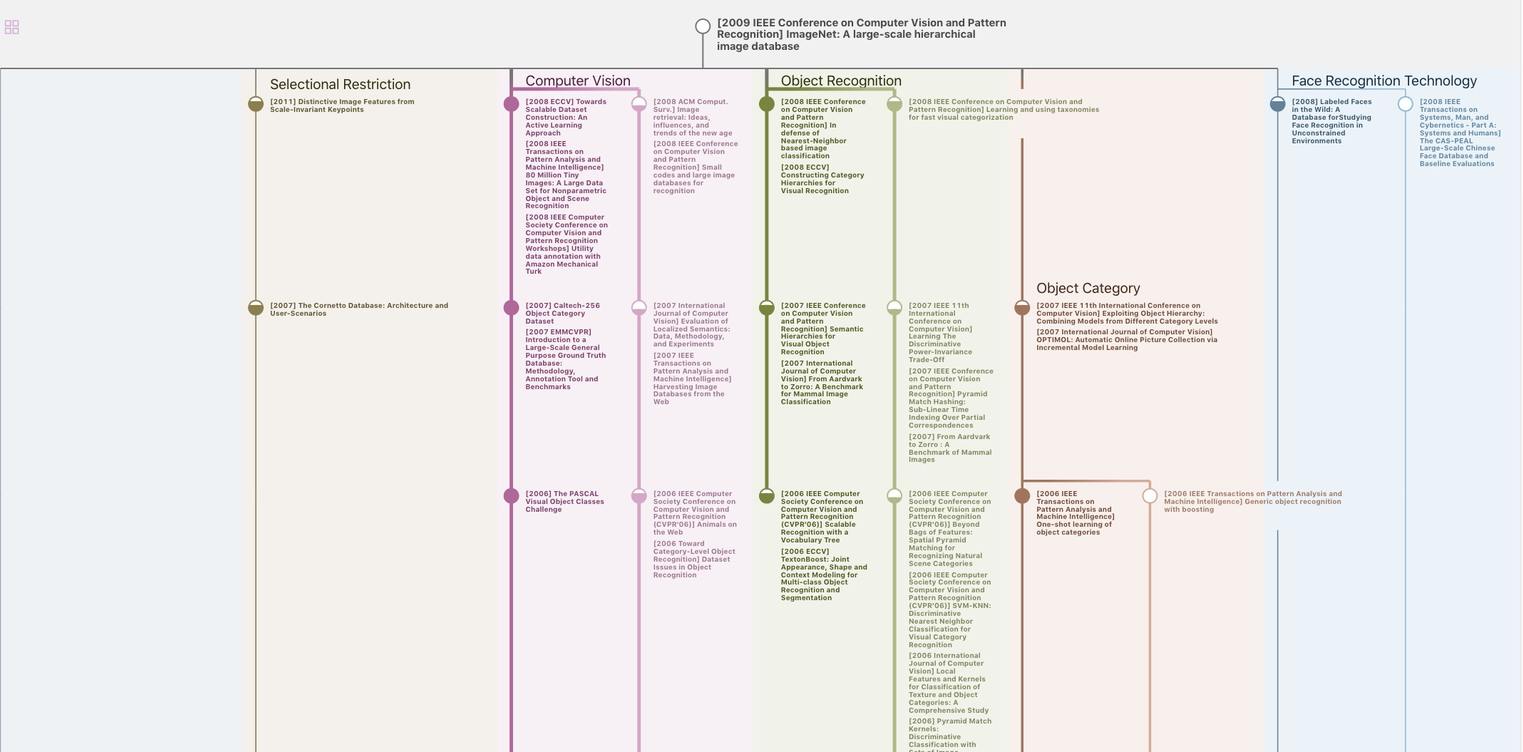
生成溯源树,研究论文发展脉络
Chat Paper
正在生成论文摘要