Neural Network Modeling Attacks on Arbiter-PUF-Based Designs
IEEE TRANSACTIONS ON INFORMATION FORENSICS AND SECURITY(2022)
摘要
By revisiting, improving, and extending recent neural-network based modeling attacks on XOR Arbiter PUFs from the literature, we show that XOR Arbiter PUFs, (XOR) Feed-Forward Arbiter PUFs, and Interpose PUFs can be attacked faster, up to larger security parameters, and with an order of magnitude fewer challenge-response pairs than previously known both in simulation and in silicon data. To support our claim, we discuss the differences and similarities of recently proposed modeling attacks and offer a fair comparison of the performance of these attacks by implementing all of them using the popular machine learning framework Keras and comparing their performance against the well-studied Logistic Regression attack. Our findings show that neural-network-based modeling attacks have the potential to outperform traditional modeling attacks on PUFs and must hence become part of the standard toolbox for PUF security analysis; the code and discussion in this paper can serve as a basis for the extension of our results to PUF designs beyond the scope of this work.
更多查看译文
关键词
Security, Data models, Analytical models, Cryptography, Neural networks, Machine learning, Predictive models, Physical unclonable function, strong PUFs, machine learning, modeling attacks, arbiter PUF
AI 理解论文
溯源树
样例
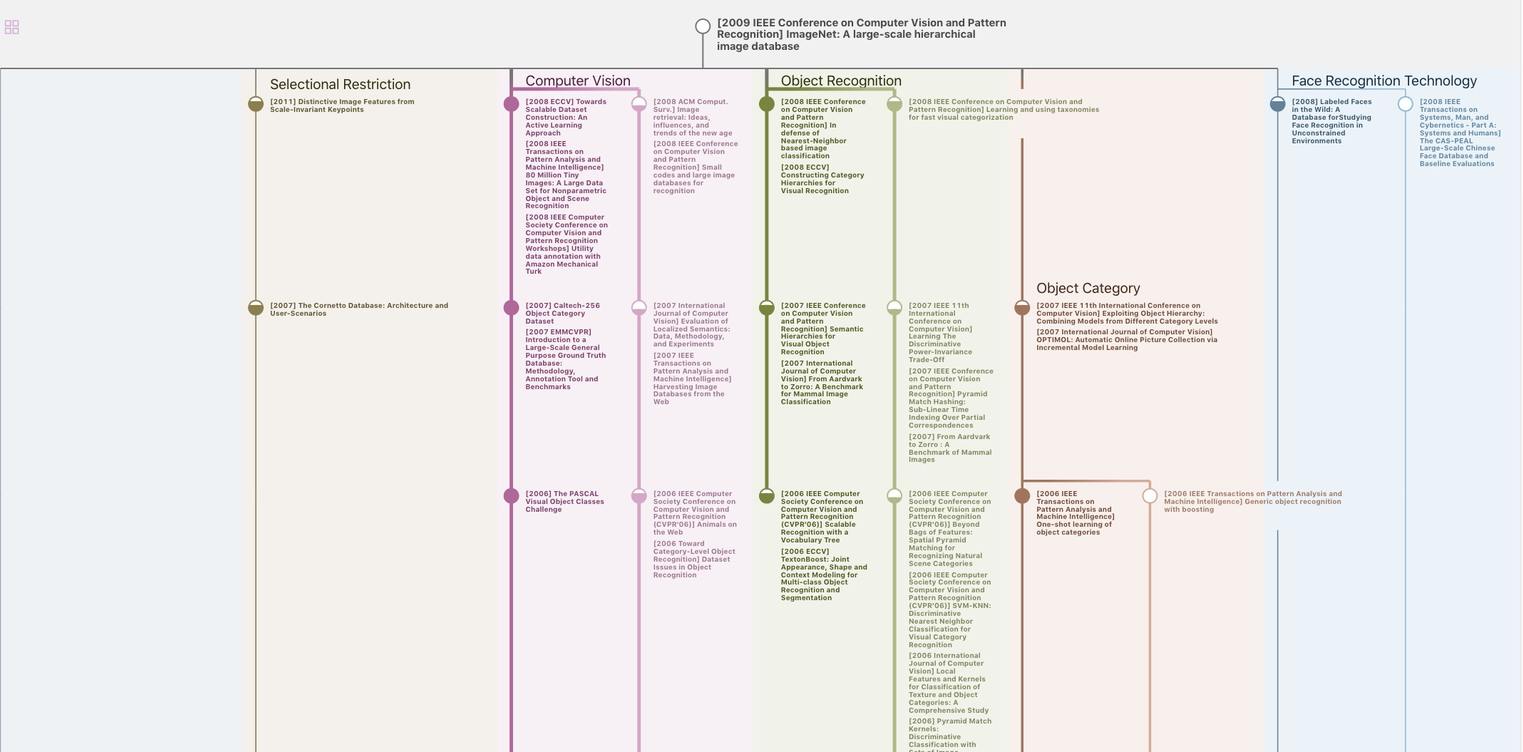
生成溯源树,研究论文发展脉络
Chat Paper
正在生成论文摘要