Efficient Constraint Learning for Data-Driven Active Distribution Network Operation
IEEE TRANSACTIONS ON POWER SYSTEMS(2024)
摘要
Scheduling flexible sources to promote the integration of renewable generation is one fundamental problem for operating active distribution networks (ADNs). However, existing works are usually based on power flow models, which require network parameters (e.g., topology and line impedance) that may be unavailable in practice. To address this issue, we propose an efficient constraint learning method to operate ADNs. This method first trains multilayer perceptrons (MLPs) based on historical data to learn the mappings from decisions to constraint violations and power loss. Then, power flow constraints can be replicated by these MLPs without network parameters. We further prove that MLPs learn constraints by formulating a union of disjoint polytopes to approximate the corresponding feasible region. Thus, the proposed method can be interpreted as a piecewise linearization method, which also explains its desirable ability to replicate complex constraints. Finally, a novel pruning method is developed to remove the useless binary variable solutions in advance, which can enhance the solution's reliability and reduce the computational complexity. Numerical experiments based on the IEEE 33- and 123-bus test systems validate that the proposed method can achieve desirable optimality, feasibility, and computational efficiency simultaneously.
更多查看译文
关键词
Active distribution networks,deep learning,renewable generation,optimal power flow,flexible sources
AI 理解论文
溯源树
样例
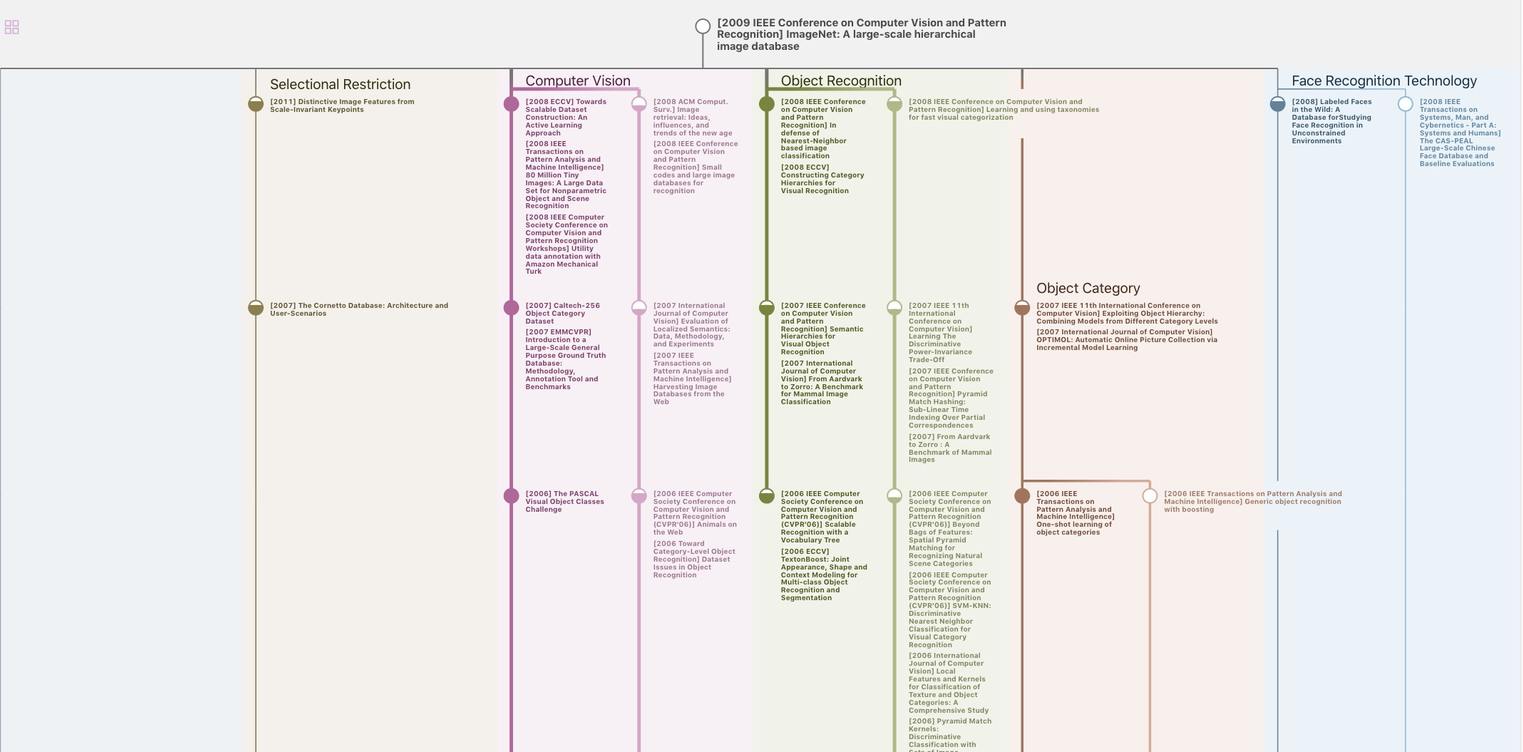
生成溯源树,研究论文发展脉络
Chat Paper
正在生成论文摘要