HaloAE: An HaloNet based Local Transformer Auto-Encoder for Anomaly Detection and Localization
arxiv(2022)
摘要
Unsupervised anomaly detection and localization is a crucial task as it is impossible to collect and label all possible anomalies. Many studies have emphasized the importance of integrating local and global information to achieve accurate segmentation of anomalies. To this end, there has been a growing interest in Transformer, which allows modeling long-range content interactions. However, global interactions through self attention are generally too expensive for most image scales. In this study, we introduce HaloAE, the first auto-encoder based on a local 2D version of Transformer with HaloNet. With HaloAE, we have created a hybrid model that combines convolution and local 2D block-wise self-attention layers and jointly performs anomaly detection and segmentation through a single model. We achieved competitive results on the MVTec dataset, suggesting that vision models incorporating Transformer could benefit from a local computation of the self-attention operation, and pave the way for other applications.
更多查看译文
关键词
halonet,haloae,transformer,auto-encoder
AI 理解论文
溯源树
样例
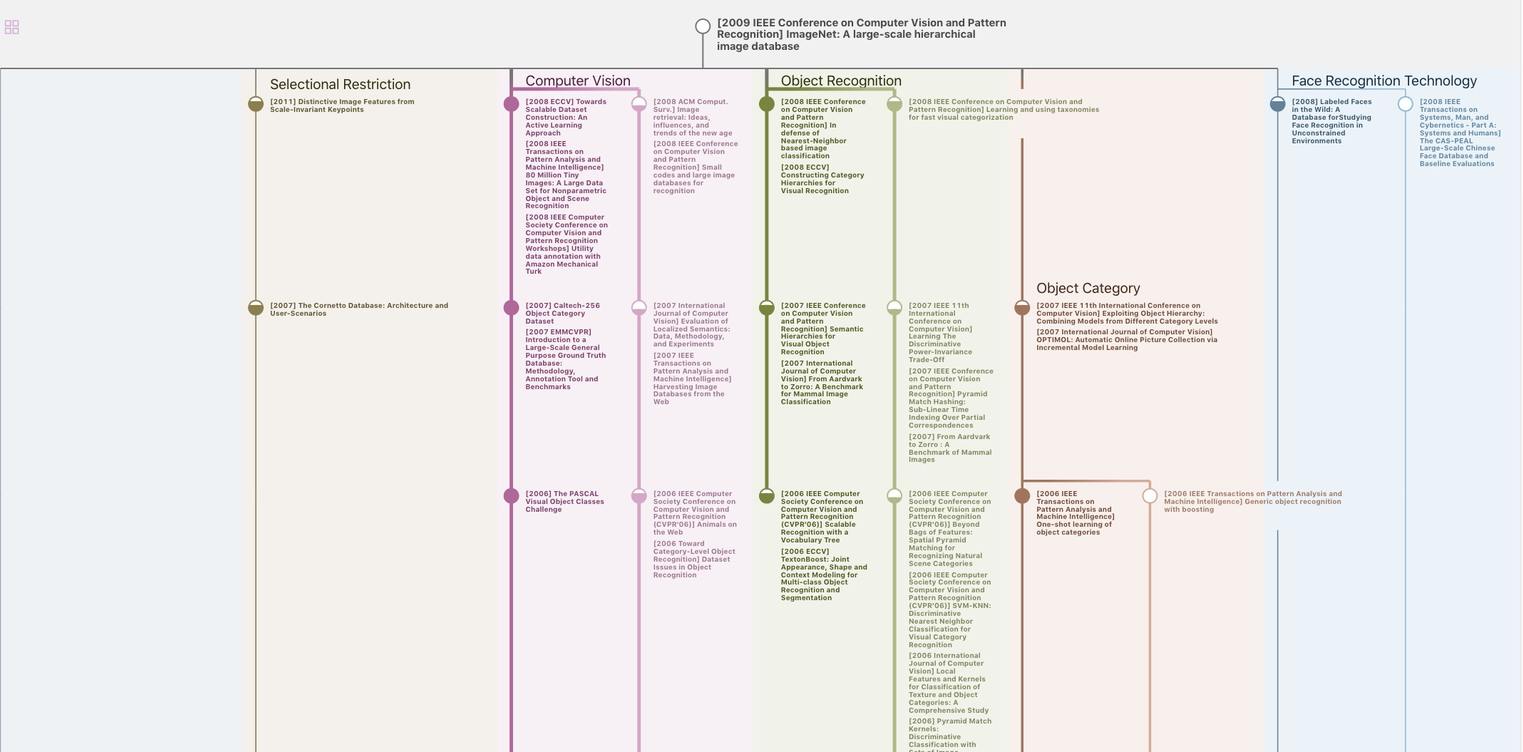
生成溯源树,研究论文发展脉络
Chat Paper
正在生成论文摘要