Diversity Learning: Introducing the Space-time Scheme to Ensemble Learning
2022 IEEE WIRELESS COMMUNICATIONS AND NETWORKING CONFERENCE (WCNC)(2022)
摘要
Inspired by diversity technology, we rethink the model enhancement from the view of wireless communication and propose a space-time framework for ensemble learning, called diversity learning. Such framework provides a new perspective that links the multi-model learning with the multi-channel communication. In this paper, 2x1 diversity learning is mainly studied whose efficiency is guaranteed theoretically. We also evaluate the proposed scheme on two popular image classification tasks, MNIST and CIFAR-10. The results elucidate that the diversity learning reaps superiority on model enhancement, convergence, complexity and robustness compared to single models as well as weighting ensemble approach. Furthermore, the diversity schemes can be deployed in several emerging distributed learning systems, especially the mobile scenarios such as edge computing and cooperative learning where the resources for computation and communication are restricted.
更多查看译文
关键词
distributed learning, diversity scheme, space-time framework, model enhancement, ensemble learning
AI 理解论文
溯源树
样例
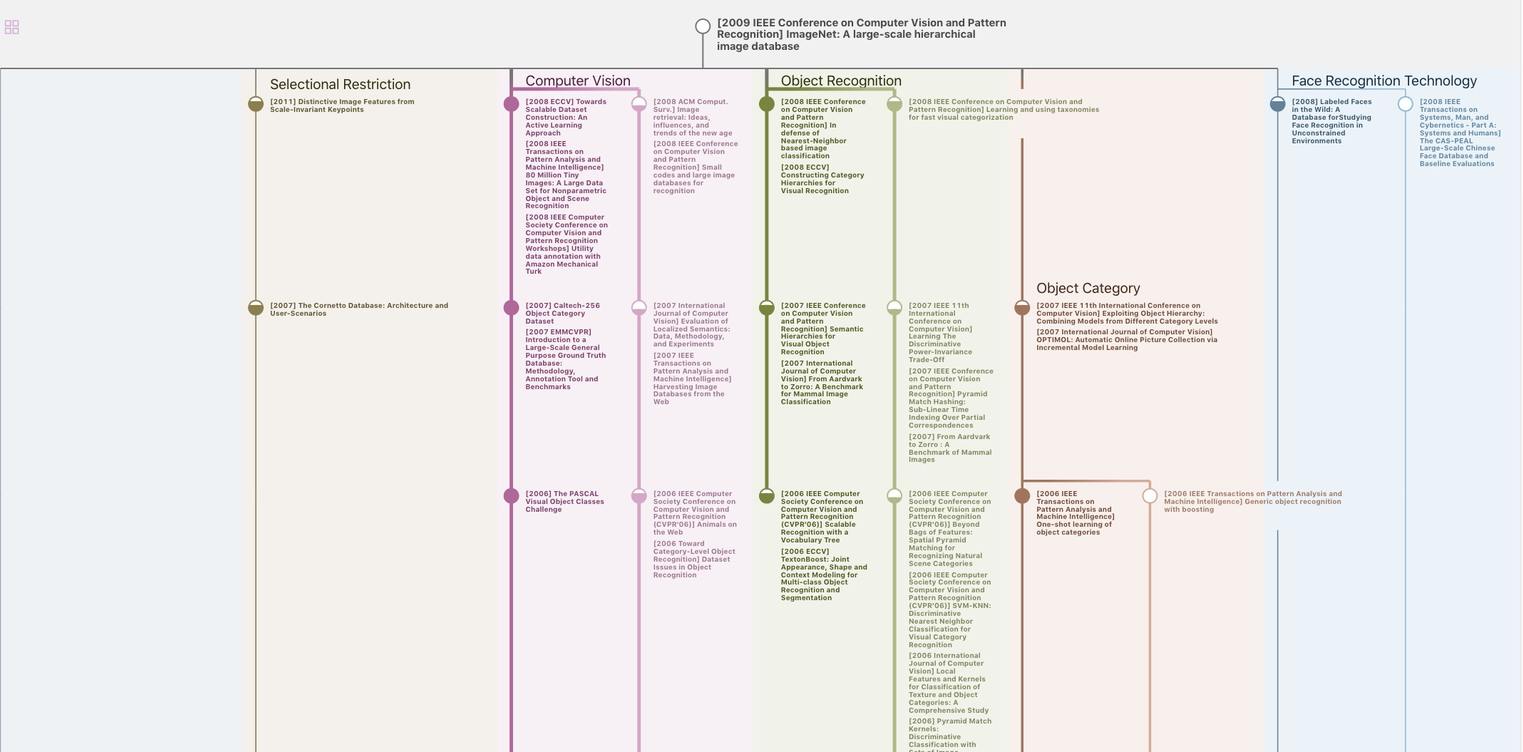
生成溯源树,研究论文发展脉络
Chat Paper
正在生成论文摘要