AccEar: Accelerometer Acoustic Eavesdropping with Unconstrained Vocabulary
IEEE Symposium on Security and Privacy (S&P)(2022)
摘要
With the increasing popularity of voice-based applications, acoustic eavesdropping has become a serious threat to users' privacy. While on smartphones the access to microphones needs an explicit user permission, acoustic eavesdropping attacks can rely on motion sensors (such as accelerometer and gyroscope), which access is unrestricted. However, previous instances of such attacks can only recognize a limited set of pre-trained words or phrases. In this paper, we present AccEar, an accelerometerbased acoustic eavesdropping attack that can reconstruct any audio played on the smartphone's loudspeaker with unconstrained vocabulary. We show that an attacker can employ a conditional Generative Adversarial Network (cGAN) to reconstruct highfidelity audio from low-frequency accelerometer signals. The presented cGAN model learns to recreate high-frequency components of the user's voice from low-frequency accelerometer signals through spectrogram enhancement. We assess the feasibility and effectiveness of AccEar attack in a thorough set of experiments using audio from 16 public personalities. As shown by the results in both objective and subjective evaluations, AccEar successfully reconstructs user speeches from accelerometer signals in different scenarios including varying sampling rate, audio volume, device model, etc.
更多查看译文
关键词
cGAN model,AccEar attack,high-frequency components,low-frequency accelerometer signals,conditional generative adversarial network,high-fidelity audio,accelerometer-based acoustic eavesdropping attack,gyroscope,motion sensors,acoustic eavesdropping attacks,voice-based applications,unconstrained vocabulary
AI 理解论文
溯源树
样例
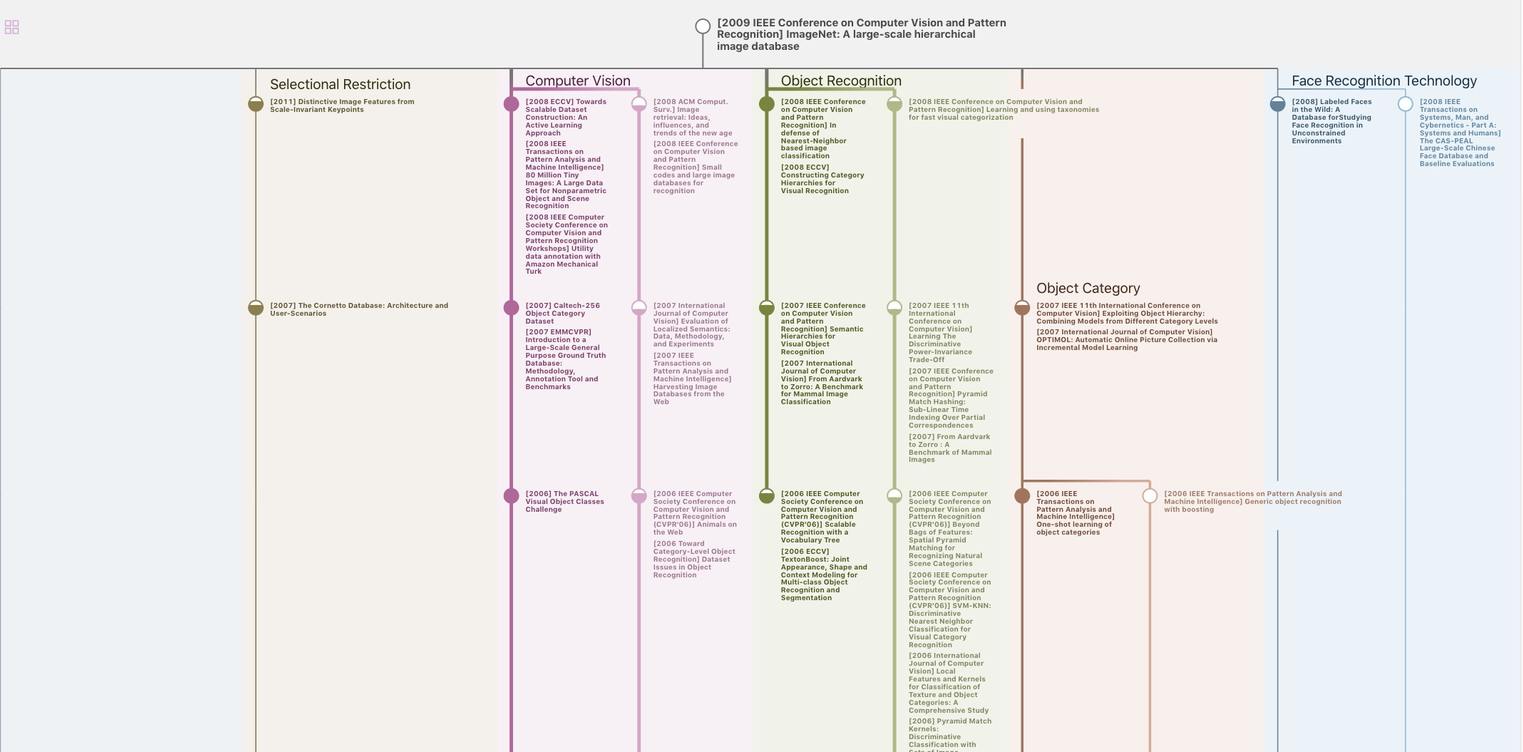
生成溯源树,研究论文发展脉络
Chat Paper
正在生成论文摘要