Why do networks have inhibitory/negative connections?
arxiv(2023)
摘要
Why do brains have inhibitory connections? Why do deep networks have negative weights? We believe representing functions is the primary role of both (i) the brain in natural intelligence, and (ii) deep networks in artificial intelligence. Our answer to why there are inhibitory/negative weights is: to learn more functions. We prove that, in the absence of negative weights, neural networks with non-decreasing activation functions are not universal approximators. While this may be an intuitive result to some, to the best of our knowledge, there is no formal theory, in either machine learning or neuroscience, that demonstrates why negative weights are crucial in the context of representation capacity. Further, we provide insights on the geometric properties of the representation space that non-negative deep networks cannot represent. We expect these insights will yield a deeper understanding of more sophisticated inductive priors imposed on the distribution of weights that lead to more efficient biological and machine learning.
更多查看译文
关键词
inhibitory/negative connections,networks
AI 理解论文
溯源树
样例
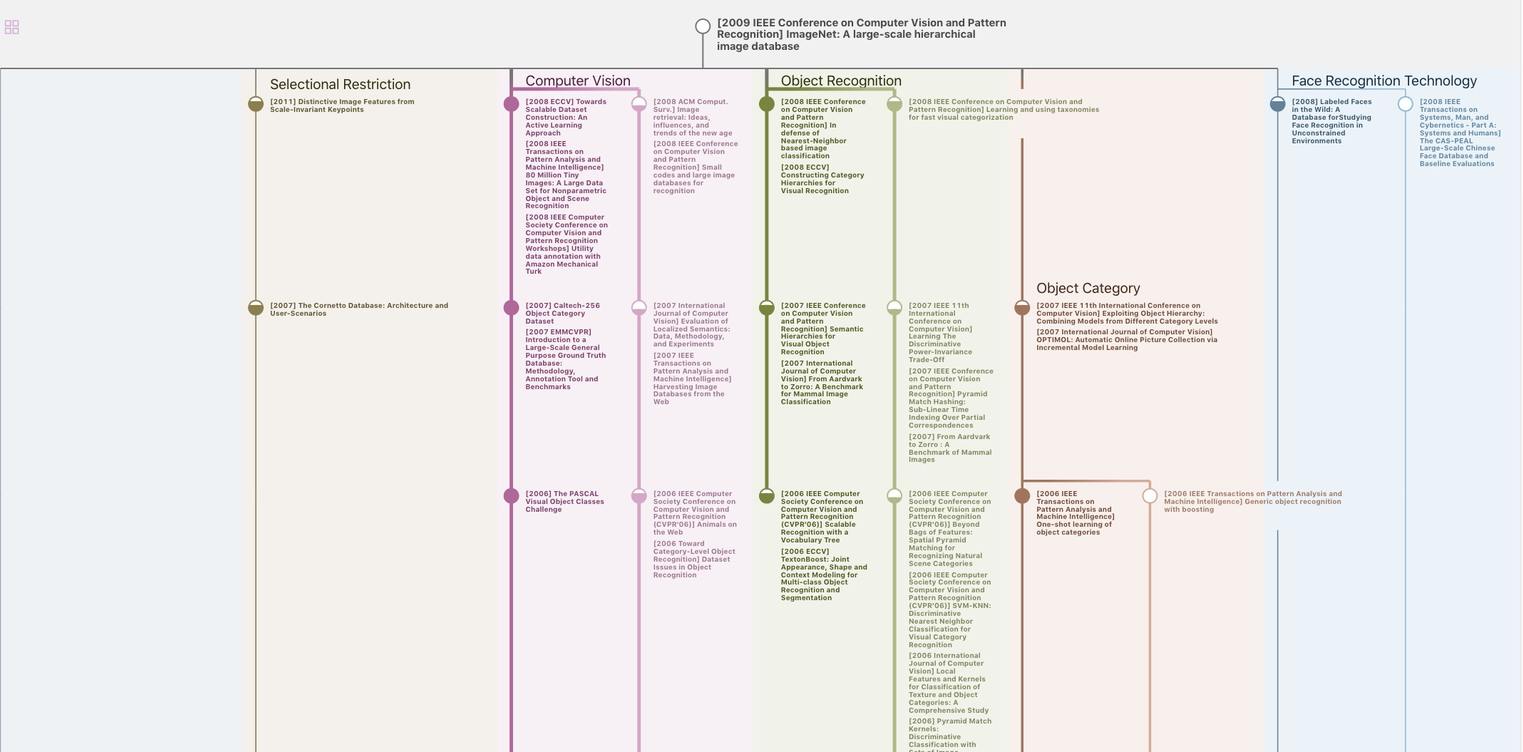
生成溯源树,研究论文发展脉络
Chat Paper
正在生成论文摘要