Grasp Stability Prediction with Sim-to-Real Transfer from Tactile Sensing.
IEEE/RJS International Conference on Intelligent RObots and Systems (IROS)(2022)
摘要
Robot simulation has been an essential tool for data-driven manipulation tasks. However, most existing simulation frameworks lack either efficient and accurate models of physical interactions with tactile sensors or realistic tactile simulation. This makes the sim-to-real transfer for tactile-based manipulation tasks still challenging. In this work, we integrate simulation of robot dynamics and vision-based tactile sensors by modeling the physics of contact. This contact model uses simulated contact forces at the robot's end-effector to inform the generation of realistic tactile outputs. To eliminate the sim-to-real transfer gap, we calibrate our physics simulator of robot dynamics, contact model, and tactile optical simulator with real-world data, and then we demonstrate the effectiveness of our system on a zero-shot sim-to-real grasp stability prediction task where we achieve an average accuracy of 90.7% on various objects. Experiments reveal the potential of applying our simulation framework to more complicated manipulation tasks. We open-source our simulation framework at https://github.com/CMURoboTouch/Taxim/tree/taxim-robot.
更多查看译文
关键词
complicated manipulation tasks,contact forces,contact model,data-driven manipulation tasks,grasp stability prediction,physical interactions,real-world data,realistic tactile outputs,realistic tactile simulation,robot dynamics,robot simulation,sim-to-real transfer gap,tactile optical simulator,tactile sensing,tactile-based manipulation tasks,vision-based tactile sensors,zero-shot sim-to-real grasp
AI 理解论文
溯源树
样例
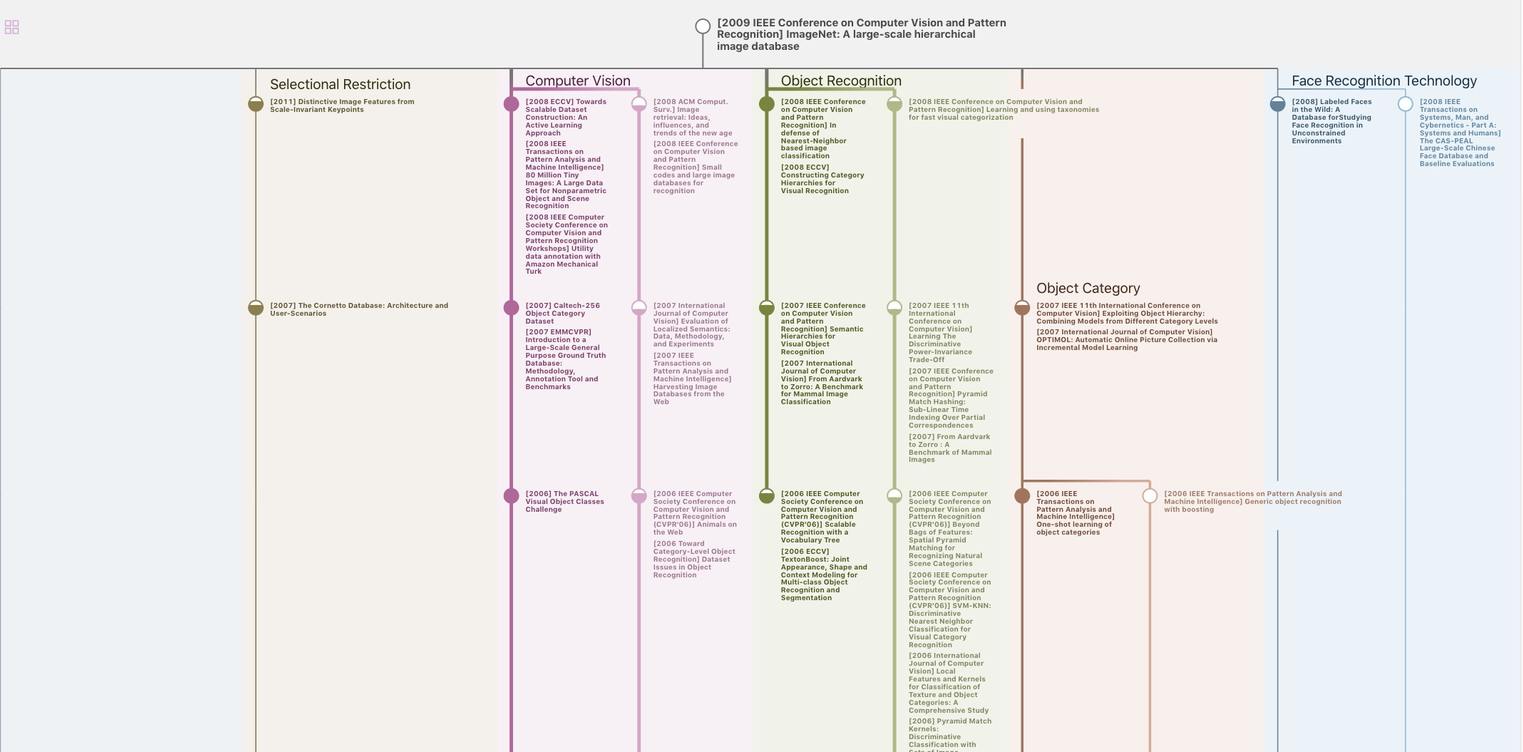
生成溯源树,研究论文发展脉络
Chat Paper
正在生成论文摘要