Discriminative Projection Learning with Adaptive Reversed Graph Embedding for Supervised and Semi-supervised Dimensionality Reduction
IEEE Transactions on Circuits and Systems for Video Technology(2022)
摘要
The graph embedding based dimensionality reduction (DR) methods have been widely used to overcome the curse of dimensionality problem in high-dimensional data. Especially in pattern recognition and data analysis, they are frequently used to extract features. However, there are two problems in the graph embedding based supervised DR methods: 1) the original data will inevitably be corrupted by noise or outliers, which makes the fixed graph constructed from these data unreliable; and 2) determining how to model the projection for enhancing the discrimination of projected samples. To overcome the above two challenges, this paper proposes a new supervised DR method called discriminative projection learning with adaptive reversed graph embedding (DP-ARGE). Specifically, DP-ARGE learns the graph dynamically in a low-dimensional subspace by using the low-dimensional embedding rather than projected samples for improving its robustness. In addition, a novel discriminative regularization term is designed to enhance the discrimination of the projection samples by using a few pairwise marginal heterogeneous samples rather than the all. To tackle the problem of estimating the neighborhood size parameter of the graph, a simple yet effective automatic parameter estimation strategy based on the density and similarity of data set is proposed. Furthermore, for better handling the data set with a few labels, DP-ARGE is extend to a semi-supervised method (SDP-ARGE) by a neighborhood preserving regularization term. SDP-ARGE can be used to preserve the local manifold structure of the data in the semi-supervised scenario. Comprehensive experiments show the superiority of the proposed methods in several real-world data sets.
更多查看译文
关键词
Dimensionality reduction,reversed graph embedding,adaptive,supervised learning,semi-supervised learning
AI 理解论文
溯源树
样例
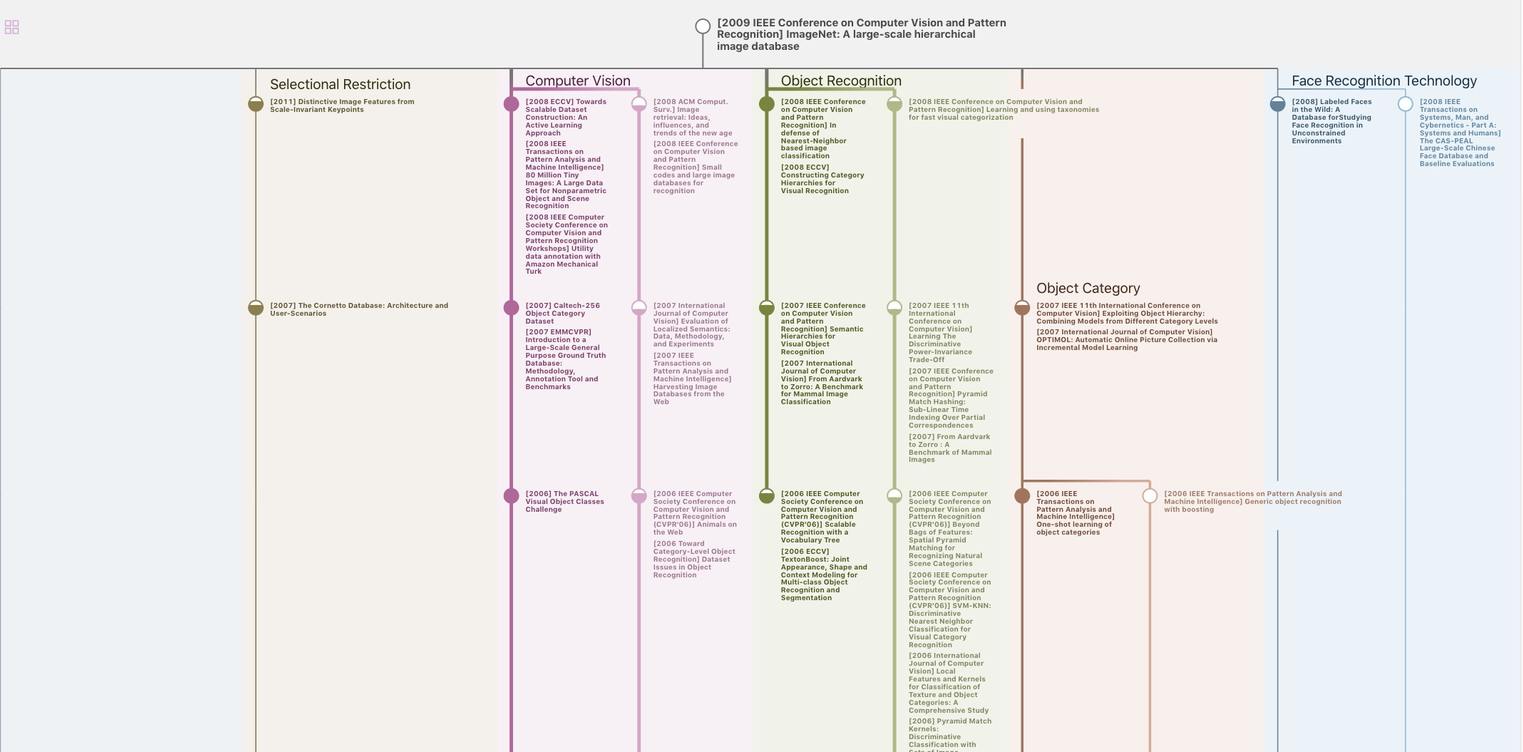
生成溯源树,研究论文发展脉络
Chat Paper
正在生成论文摘要