Deep Learning Approach of Drilling Decision for Subhorizontal Drain Geosteering Based on APC-LSTM Model
SPE Drilling & Completion(2022)
摘要
Summary Steering drilling is used for exploring oil, natural gas, and other liquid and gaseous minerals. Steering drilling consists of high-efficiency drill bits, steering power drilling tools, and logging while drilling (LWD) and is used in petroleum drilling engineering. This paper mainly discusses subhorizontal drain geosteering, one of the methods of guided subhorizontal drilling. We use the currently popular deep learning method to conduct intelligent guided drilling. Geosteering is a sequential drilling decision process under uncertain stratum environment. However, the current geosteering drilling process relies heavily on manual work and has no use of temporal context. This paper aims to solve decision-making of geosteering in deep well (between 4500 and 6000 km) or ultradeep well (between 6000 and 9000 km). To this end, we make three contributions: (1) a wide-angle eye mechanism to obtain more geological information; (2) an asymmetric peephole convolutional long short-term memory (APC-LSTM) approach for geosteering drilling decision, whose input data were assembled with the wide-angle eye mechanism; and (3) use of the deep convolution generative adversarial networks (DCGAN) model to generate simulated logging data and conduct experiments in the simulation environment to verify our proposed method. APC-LSTM can capture the spatial-temporal correlation better between different strata for decision-making. Meanwhile, the APC-LSTM drilling decision model achieved better performance than other advanced methods in two drilling data sets. Tested in a simulative drilling environment, our proposed model achieves excellent application effect. Moreover, our method has been applied to the wells of oil field in practice.
更多查看译文
关键词
drilling decision,subhorizontal drain geosteering,deep learning,apc-lstm
AI 理解论文
溯源树
样例
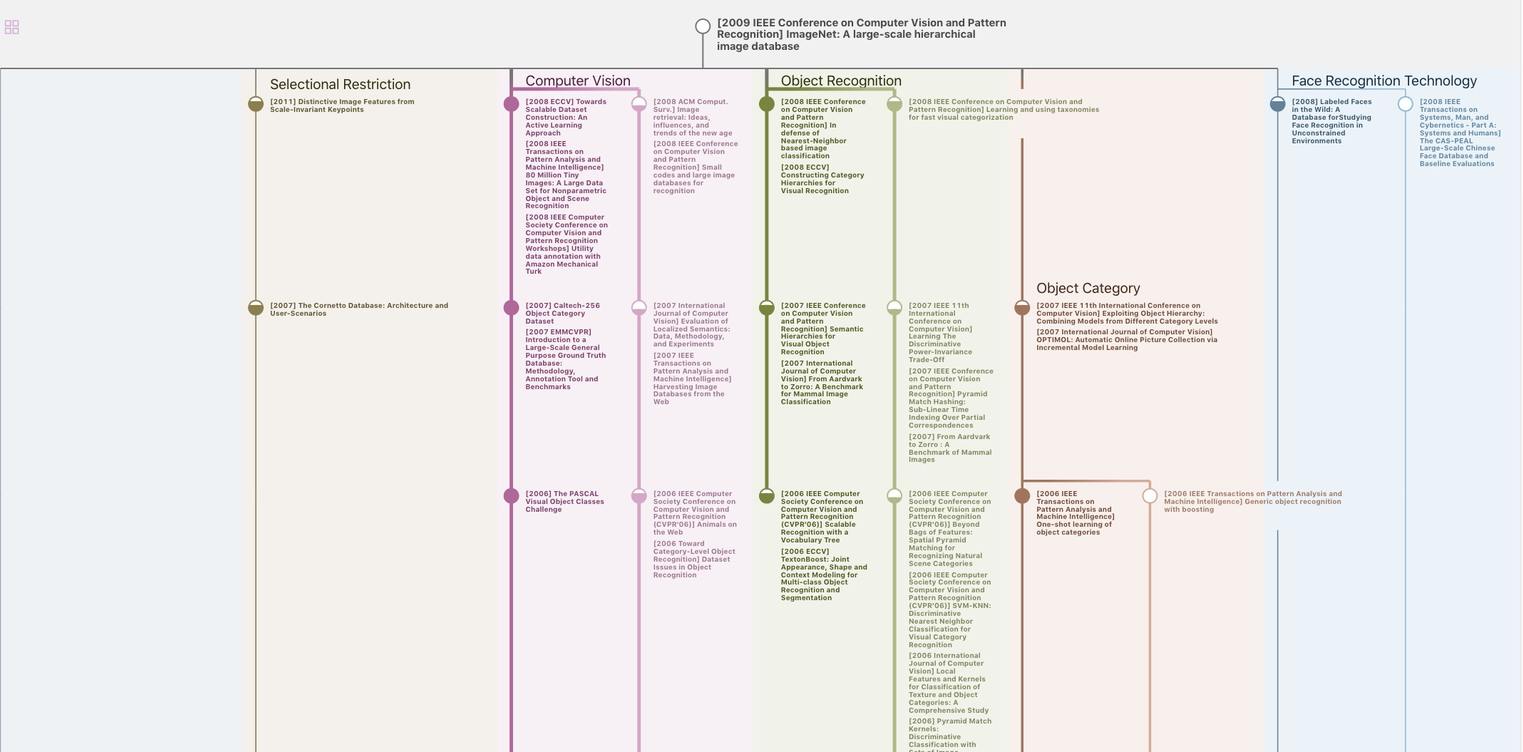
生成溯源树,研究论文发展脉络
Chat Paper
正在生成论文摘要