Hybrid connected attentional lightweight network for gangue intelligent segmentation in top-coal caving face
Journal of Intelligent & Fuzzy Systems(2023)
摘要
The estimation of gangue content is the main basis for intelligent top coal caving mining by computer vision, and the automatic segmentation of gangue is crucial to computer vision analysis. However, it is still a great challenge due to the degradation of images and the limitation of computing resources. In this paper, a hybrid connected attentional lightweight network (HALNet) with high speed, few parameters and high accuracy is proposed for gangue intelligent segmentation on the conveyor in the top-coal caving face. Firstly, we propose a deep separable dilation convolution block (DSDC) combining deep separable convolution and dilation convolution, which can provide a larger receptive field to learn more information and reduce the size and computational cost of the model. Secondly, a bridging residual learning framework is designed as the basic unit of encoder and decoder to minimize the loss of semantic information in the process of feature extraction. An attention fusion block (AFB) with skip pathway is introduced to capture more representative and distinctive features through the fusion of high-level and low-level features. Finally, the proposed network is trained through the expanded dataset, and the gangue image segmentation results are obtained by pixel-by-pixel classification method. The experimental results show that the proposed HALNet reduces about 57 percentage parameters compared with U-Net, and achieves state-of-the art performance on dataset.
更多查看译文
关键词
Gangue intelligent segmentation, the top-coal caving face, depthwise separable dilation convolution, attention mechanism
AI 理解论文
溯源树
样例
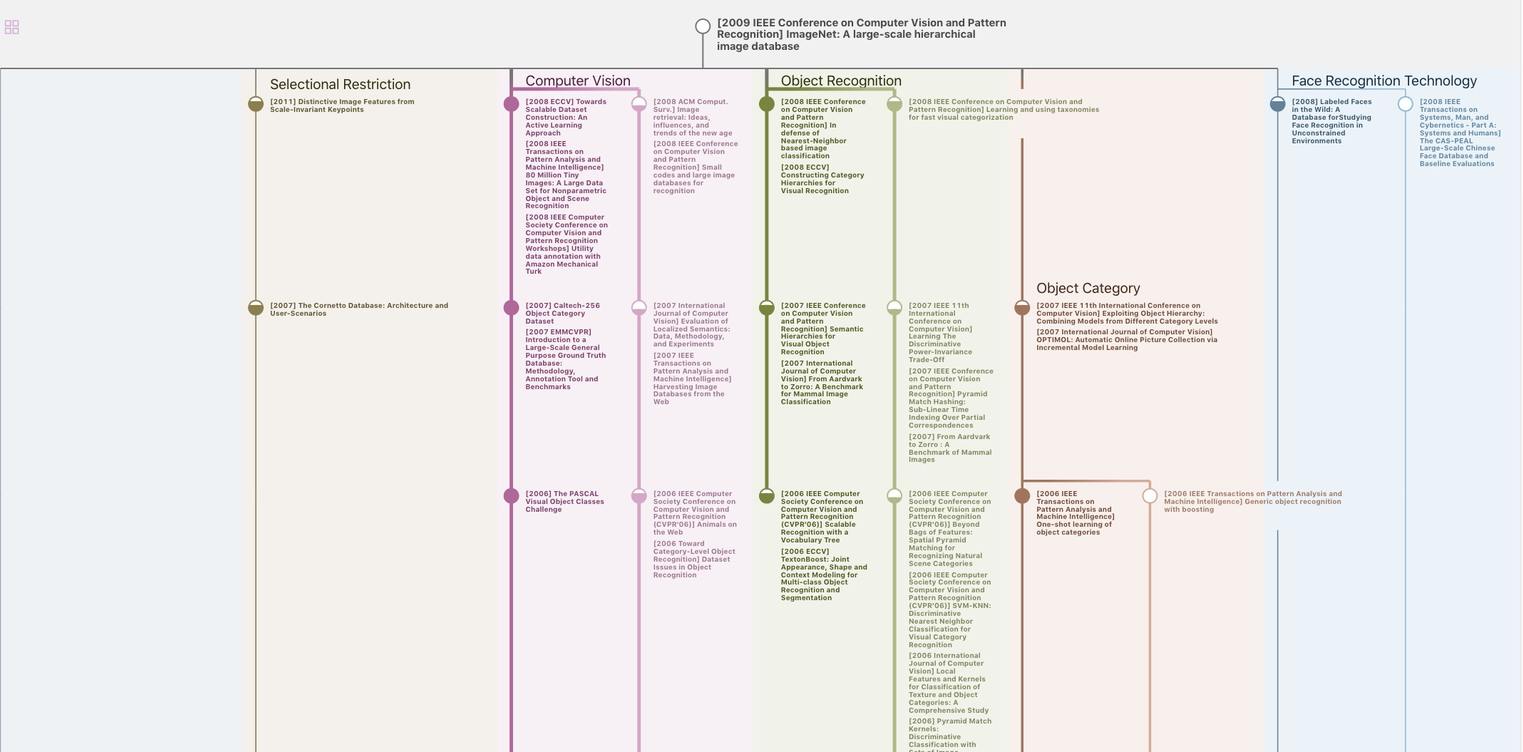
生成溯源树,研究论文发展脉络
Chat Paper
正在生成论文摘要