When do Models Generalize? A Perspective from Data-Algorithm Compatibility
arxiv(2022)
摘要
One of the major open problems in machine learning is to characterize generalization in the overparameterized regime, where most traditional generalization bounds become inconsistent (Nagarajan and Kolter, 2019). In many scenarios, their failure can be attributed to obscuring the crucial interplay between the training algorithm and the underlying data distribution. To address this issue, we propose a concept named compatibility, which quantitatively characterizes generalization in a both data-relevant and algorithm-relevant manner. By considering the entire training trajectory and focusing on early-stopping iterates, compatibility exploits the data and the algorithm information and is therefore a more suitable notion for generalization. We validate this by theoretically studying compatibility under the setting of solving overparameterized linear regression with gradient descent. Specifically, we perform a data-dependent trajectory analysis and derive a sufficient condition for compatibility in such a setting. Our theoretical results demonstrate that in the sense of compatibility, generalization holds with significantly weaker restrictions on the problem instance than the previous last iterate analysis.
更多查看译文
关键词
generalization,data-algorithm compatibility,early stopping,overparameterized linear regression
AI 理解论文
溯源树
样例
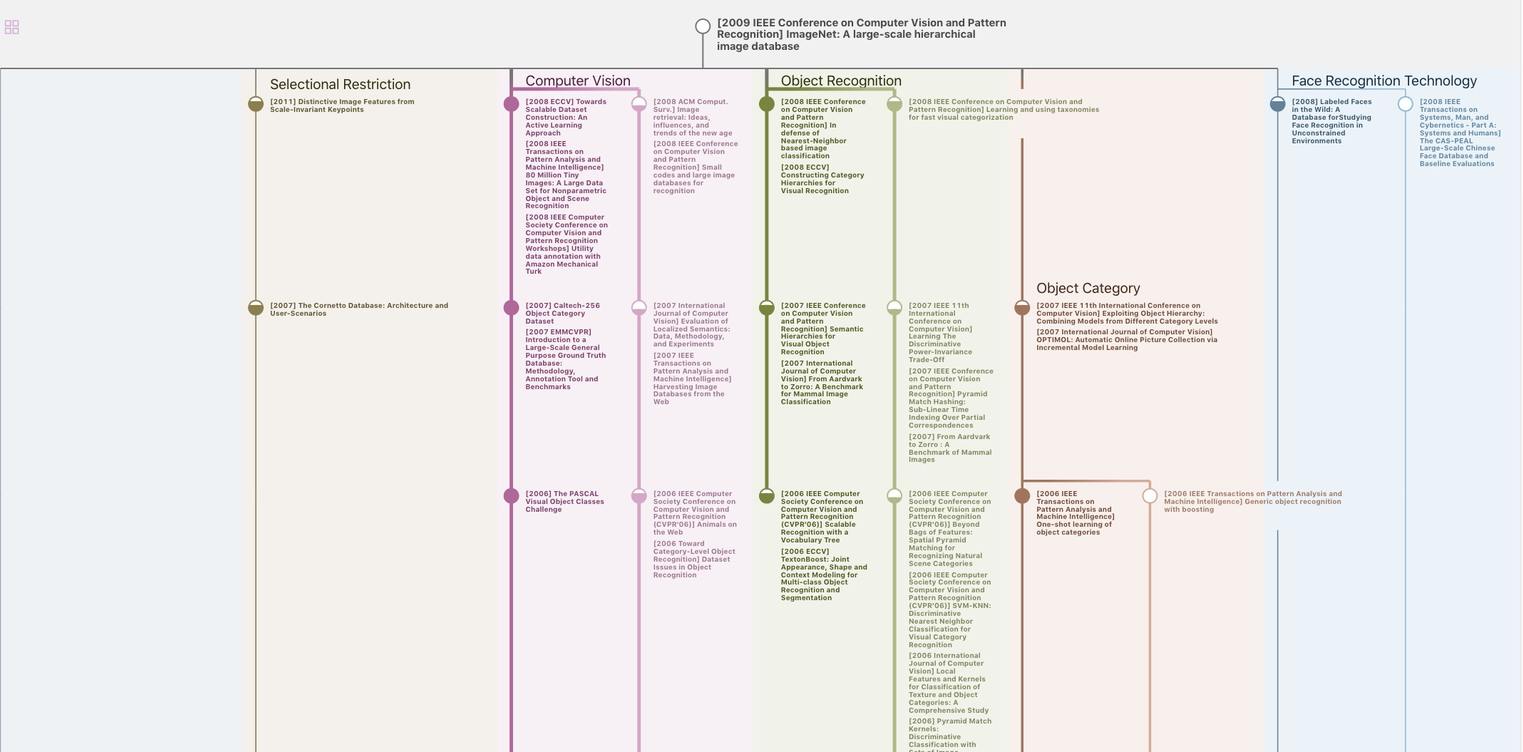
生成溯源树,研究论文发展脉络
Chat Paper
正在生成论文摘要