Task relevance driven adversarial learning for simultaneous detection, size grading, and quantification of hepatocellular carcinoma via integrating multi-modality MRI.
Medical image analysis(2022)
摘要
Hepatocellular Carcinoma (HCC) detection, size grading, and quantification (i.e. the center point coordinates, max-diameter, and area) by using multi-modality magnetic resonance imaging (MRI) are clinically significant tasks for HCC assessment and treatment. However, delivering the three tasks simultaneously is extremely challenging due to: (1) the lack of effective an mechanism to capture the relevance among multi-modality MRI information for multi-modality feature fusion and selection; (2) the lack of effective mechanism and constraint strategy to achieve mutual promotion of multi-task. In this paper, we proposed a task relevance driven adversarial learning framework (TrdAL) for simultaneous HCC detection, size grading, and multi-index quantification using multi-modality MRI (i.e. in-phase, out-phase, T2FS, and DWI). The TrdAL first obtains expressive feature of dimension reduction via using a CNN-based encoder. Secondly, the proposed modality-aware Transformer is utilized for multi-modality MRI features fusion and selection, which solves the challenge of multi-modality information diversity via capturing the relevance among multi-modality MRI. Then, the innovative task relevance driven and radiomics guided discriminator (Trd-Rg-D) is used for united adversarial learning. The Trd-Rg-D captures the internal high-order relationships to refine the performance of multi-task simultaneously. Moreover, adding the radiomics feature as the prior knowledge into Trd-Rg-D enhances the detailed feature extraction. Lastly, a novel task interaction loss function is used for constraining the TrdAL, which enforces the higher-order consistency among multi-task labels to enhance mutual promotion. The TrdAL is validated on a corresponding multi-modality MRI of 135 subjects. The experiments demonstrate that TrdAL achieves high accuracy of (1) HCC detection: specificity of 93.71%, sensitivity of 93.15%, accuracy of 93.33%, and IoU of 82.93%; (2) size grading: accuracy of large size, medium size, small size, tiny size, and healthy subject are 90.38%, 87.74%, 80.68%, 77.78%, and 96.87%; (3) multi-index quantification: the mean absolute error of center point, max-diameter, and area are 2.74mm, 3.17mm, and 144.51mm2. All of these results indicate that the proposed TrdAL provides an efficient, accurate, and reliable tool for HCC diagnosis in clinical.
更多查看译文
AI 理解论文
溯源树
样例
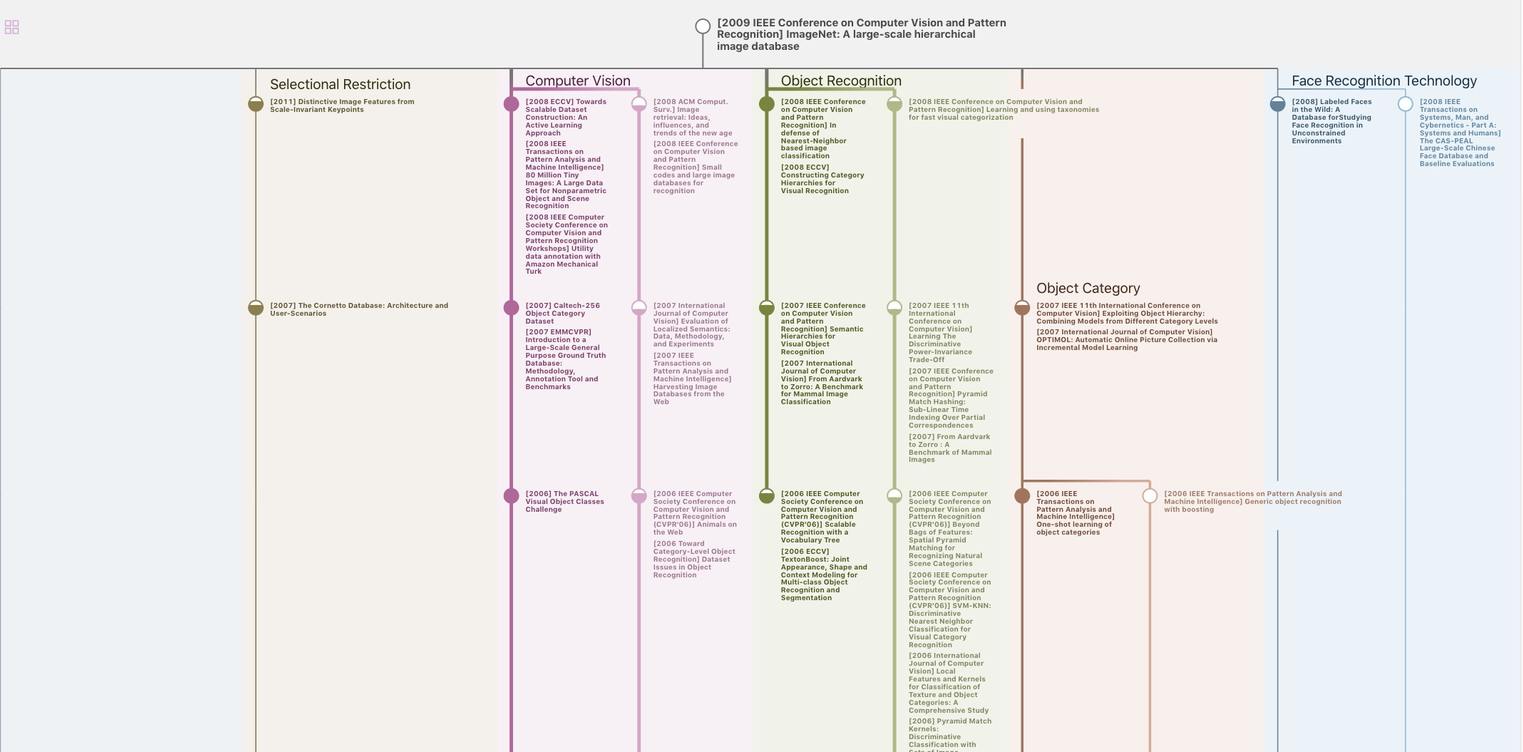
生成溯源树,研究论文发展脉络
Chat Paper
正在生成论文摘要