Elastic temporal alignment for few‐shot action recognition
IET Computer Vision(2022)
摘要
Few-shot action recognition aims to learn a classification model with good generalisation ability when trained with only a few labelled videos. However, it is difficult to learn discriminative feature representations for videos in such a setting. The Elastic Temporal Alignment (ETA) for few-shot action recognition is proposed. First, a convolutional neural network is employed to extract feature representations of video frames sparsely sampled from videos. In order to obtain the similarity of two videos, a temporal alignment estimation function is utilised to estimate the matching score between each pair of frames from the two videos through an elastic alignment mechanism. The analysis shows that when we judge whether two frames from respective videos are matched, multiple adjacent frames in the videos should be considered, so as to embody the temporal information. Thus, before feeding per-frame feature vectors of videos into the temporal alignment estimation function, a temporal message passing function is leveraged to propagate the information of per-frame features in the temporal domain. The method has been evaluated on four action recognition datasets, including Kinetics, Something-Something V2, HMDB51, and UCF101. The experimental results verify the effectiveness of ETA and show its superiority over state-of-the-art methods.
更多查看译文
AI 理解论文
溯源树
样例
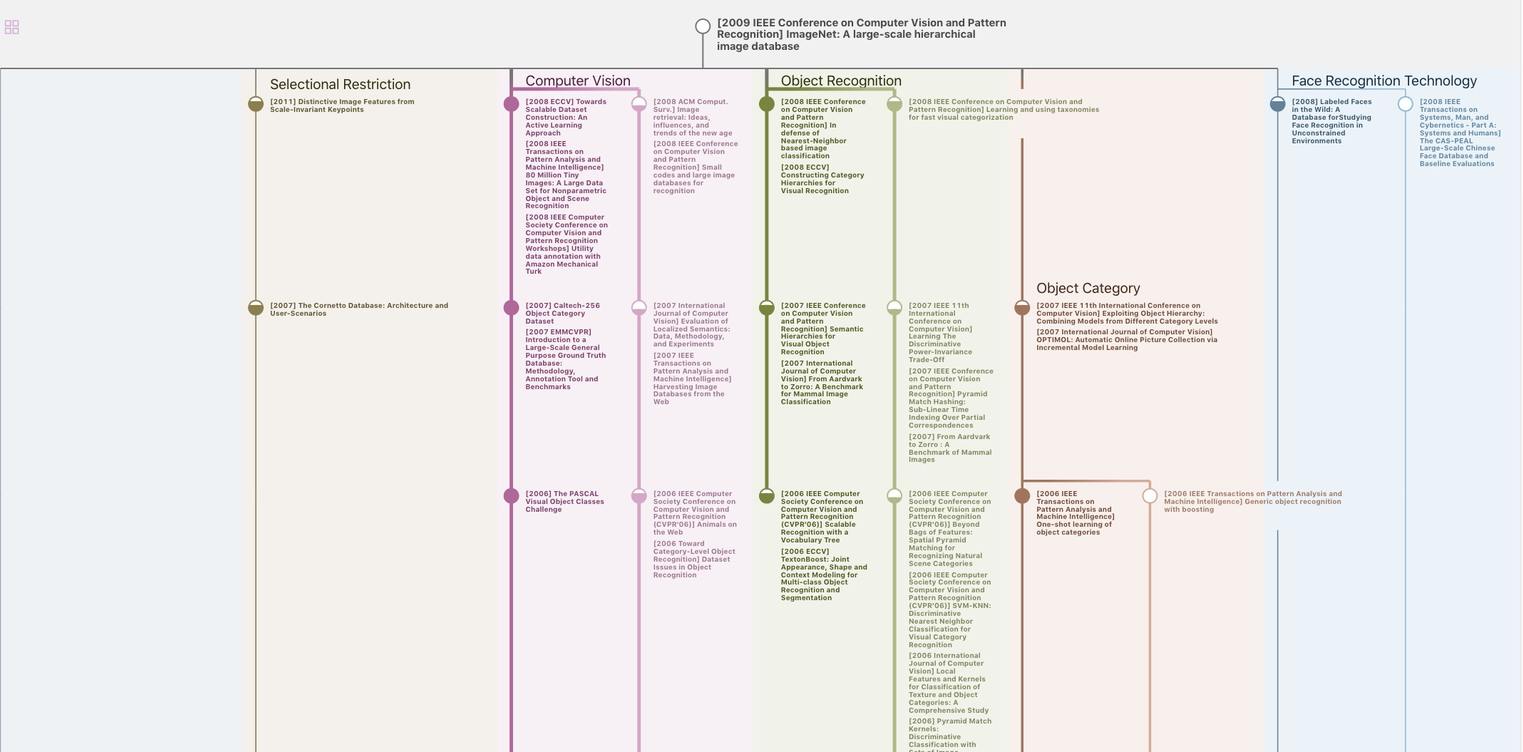
生成溯源树,研究论文发展脉络
Chat Paper
正在生成论文摘要