Adaptive Blending of Probabilistic Precipitation Forecasts with Emphasis on Calibration and Temporal Forecast Consistency
Artificial Intelligence for the Earth Systems(2022)
摘要
Abstract A wealth of forecasting models is available for operational weather forecasting. Their strengths often depend on the lead time considered, which generates the need for a seamless combination of different forecast methods. The combined and continuous products are made in order to retain or even enhance the forecast quality of the individual forecasts and to extend the lead time to potentially hazardous weather events. In this study, we further improve an artificial neural network based combination model that was recently proposed in a previous paper. This model combines two initial precipitation ensemble forecasts and produces exceedance probabilities for a set of thresholds for hourly precipitation amounts. Both initial forecasts perform differently well for different lead times, whereas the combined forecast is calibrated and outperforms both initial forecasts with respect to various validation scores and for all considered lead times (+1h to +6h). Moreover, the robustness of the combination model is tested by applying it to a new dataset and by evaluating the spatial and temporal consistency of its forecasts. The changes proposed further improve the forecast quality and make it more useful for practical applications. Temporal consistency of the combined product is evaluated using a flip-flop index. It is shown that the combination provides a higher persistence with decreasing lead times compared to both input systems.
更多查看译文
AI 理解论文
溯源树
样例
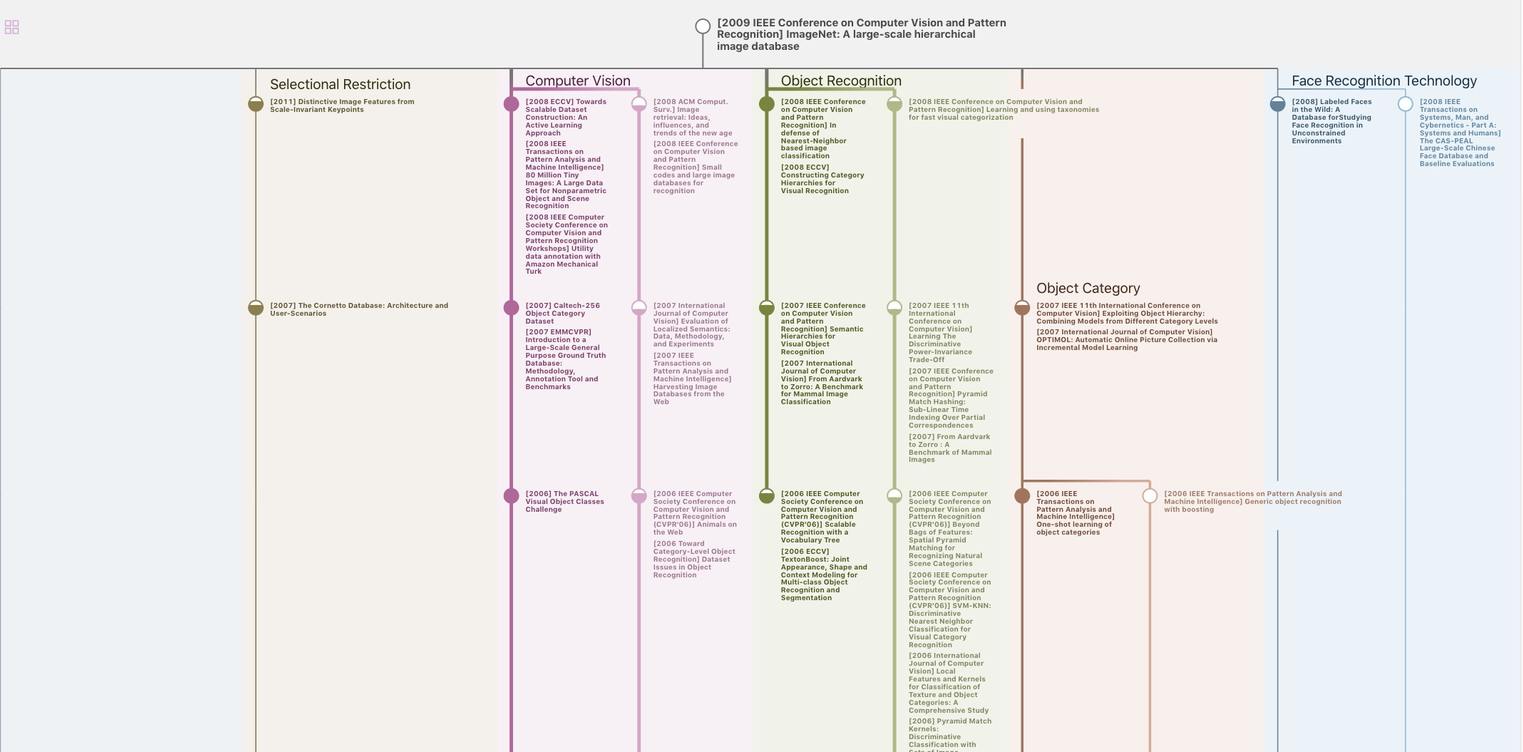
生成溯源树,研究论文发展脉络
Chat Paper
正在生成论文摘要