Diversity embedding deep matrix factorization for multi-view clustering
Information Sciences(2022)
摘要
Multi-view clustering has attracted increasing attention by reason of its ability to leverage the complementarity of multi-view data. Existing multi-view clustering methods have explored nonnegative matrix factorization to decompose a matrix into multiple matrices for feature representations from multi-view data, which are not discriminative enough to deal with the natural data containing complex information. Moreover, most of multi-view clustering methods prioritize the consensus information among multi-view data, leaving a large amount of information redundant and the clustering performance deteriorated. To address these issues, this paper proposes a multi-view clustering framework that adopts a diversity loss for deep matrix factorization and reduces feature redundancy while obtaining more discriminative features. We then bridge the relation between deep auto-encoder and deep matrix factorization to optimize the objective function. This method avoids the challenges in the optimization process. Extensive experiments demonstrate that the proposed method is superior to state-of-the-art methods.
更多查看译文
关键词
Multi-view clustering,Deep matrix factorization,Deep learning,Diversity embedding
AI 理解论文
溯源树
样例
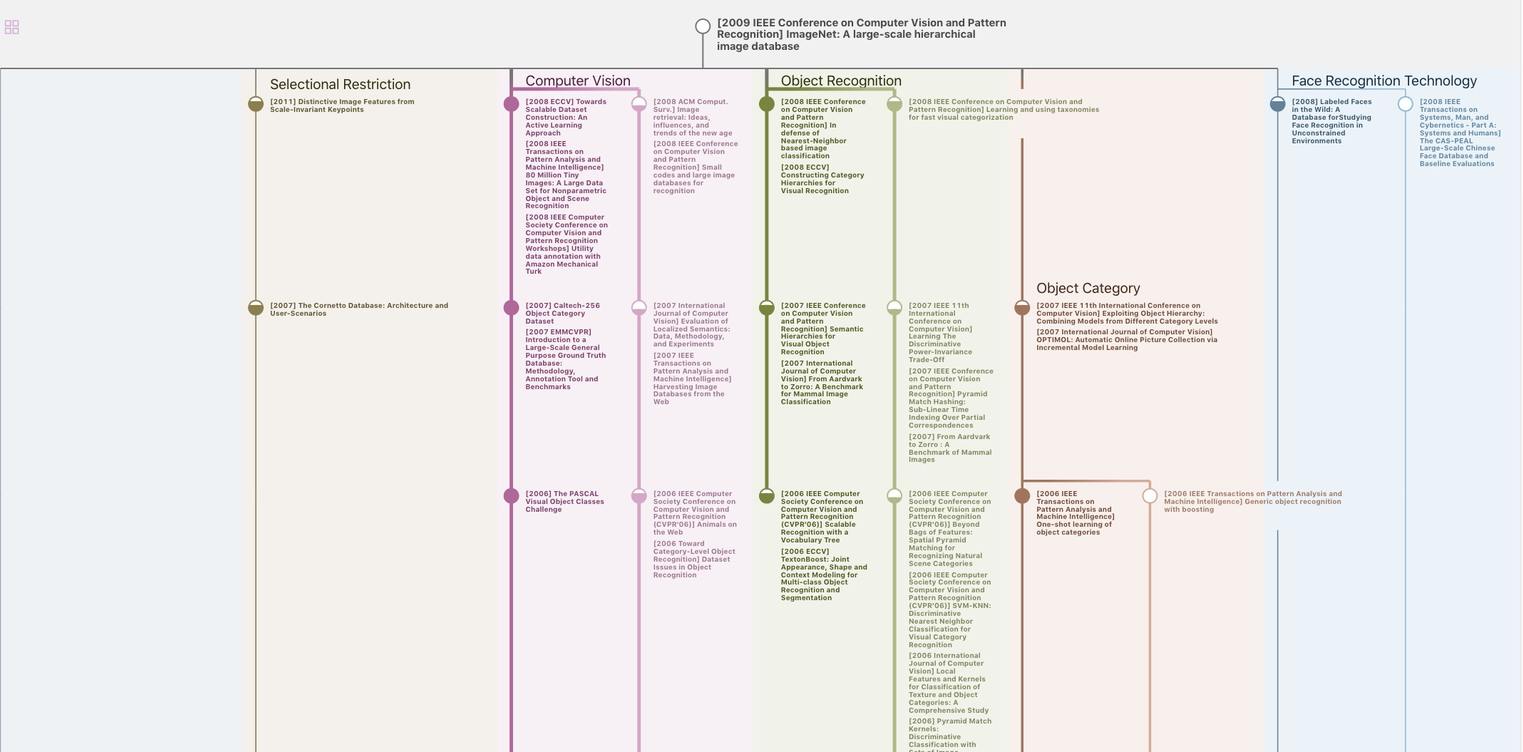
生成溯源树,研究论文发展脉络
Chat Paper
正在生成论文摘要