Neural network accelerator for quantum control
2022 IEEE/ACM Third International Workshop on Quantum Computing Software (QCS)(2022)
摘要
Efficient quantum control is necessary for practical quantum computing implementations with current technologies. Conventional algorithms for determining optimal control parameters are computationally expensive, largely excluding them from use outside of the simulation. Existing hardware solutions structured as lookup tables are imprecise and costly. By designing a machine learning model to approximate the results of traditional tools, a more efficient method can be produced. Such a model can then be synthesized into a hardware accelerator for use in quantum systems. In this study, we demonstrate a machine learning algorithm for predicting optimal pulse parameters. This algorithm is lightweight enough to fit on a low-resource FPGA and perform inference with a latency of 175 ns and pipeline interval of 5 ns with > 0.99 gate fidelity. In the long term, such an accelerator could be used near quantum computing hardware where traditional computers cannot operate, enabling quantum control at a reasonable cost at low latencies without incurring large data bandwidths outside of the cryogenic environment.
更多查看译文
关键词
hls4ml,machine learning,neural networks,quantum control,FPGA,low-power,low-latency
AI 理解论文
溯源树
样例
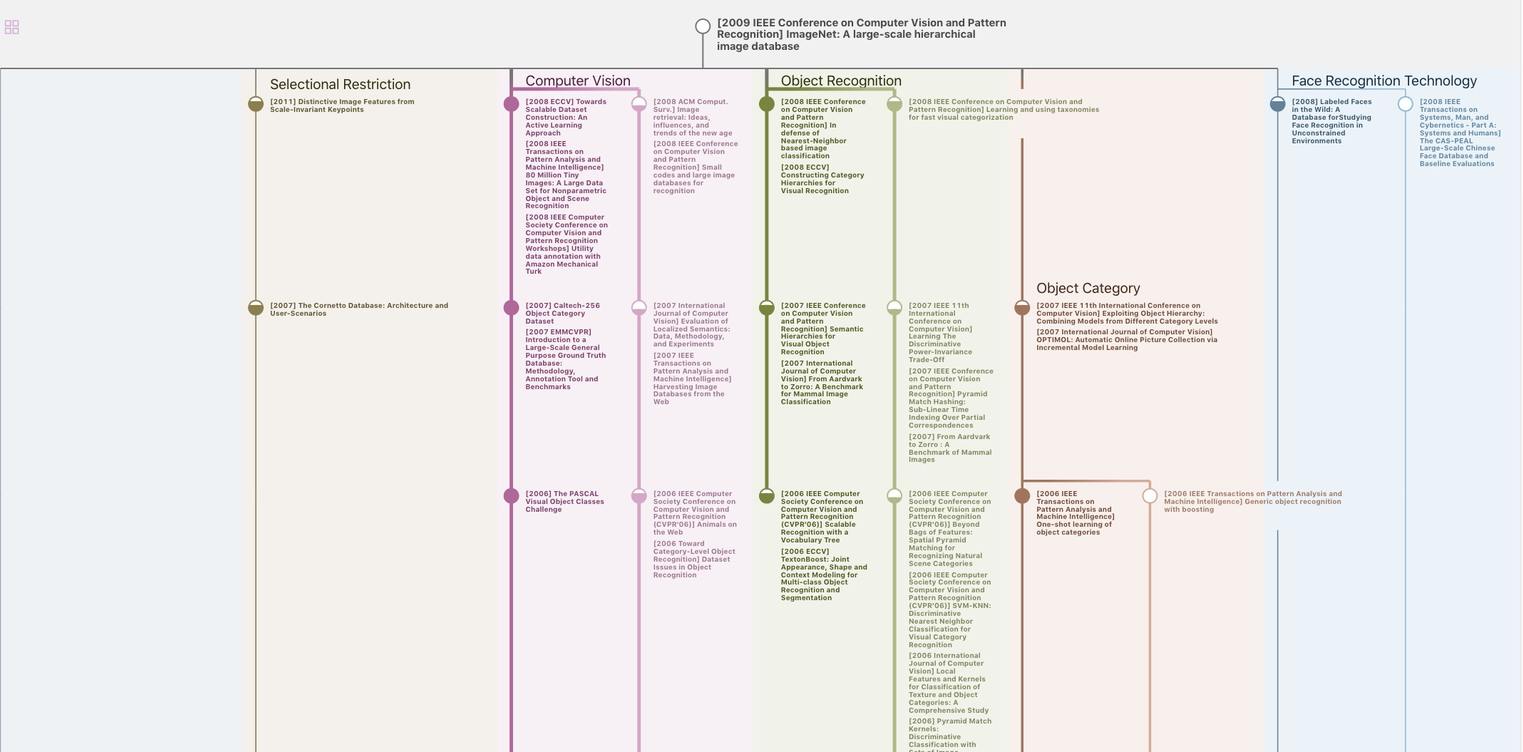
生成溯源树,研究论文发展脉络
Chat Paper
正在生成论文摘要