Prevalence estimation and optimal classification methods to account for time dependence in antibody levels
Journal of Theoretical Biology(2023)
摘要
Serology testing can identify past infection by quantifying the immune response of an infected individual providing important public health guidance. Individual immune responses are time-dependent, which is reflected in antibody measurements. Moreover, the probability of obtaining a particular measurement from a random sample changes due to changing prevalence (i.e., seroprevalence, or fraction of individuals exhibiting an immune response) of the disease in the population. Taking into account these personal and population-level effects, we develop a mathematical model that suggests a natural adaptive scheme for estimating prevalence as a function of time. We then combine the estimated prevalence with optimal decision theory to develop a time-dependent probabilistic classification scheme that minimizes the error associated with classifying a value as positive (history of infection) or negative (no such history) on a given day since the start of the pandemic. We validate this analysis by using a combination of real-world and synthetic SARS-CoV-2 data and discuss the type of longitudinal studies needed to execute this scheme in real-world settings.
更多查看译文
关键词
Time-dependent classification,SARS-CoV-2,Prevalence estimation,Optimization,Antibody testing
AI 理解论文
溯源树
样例
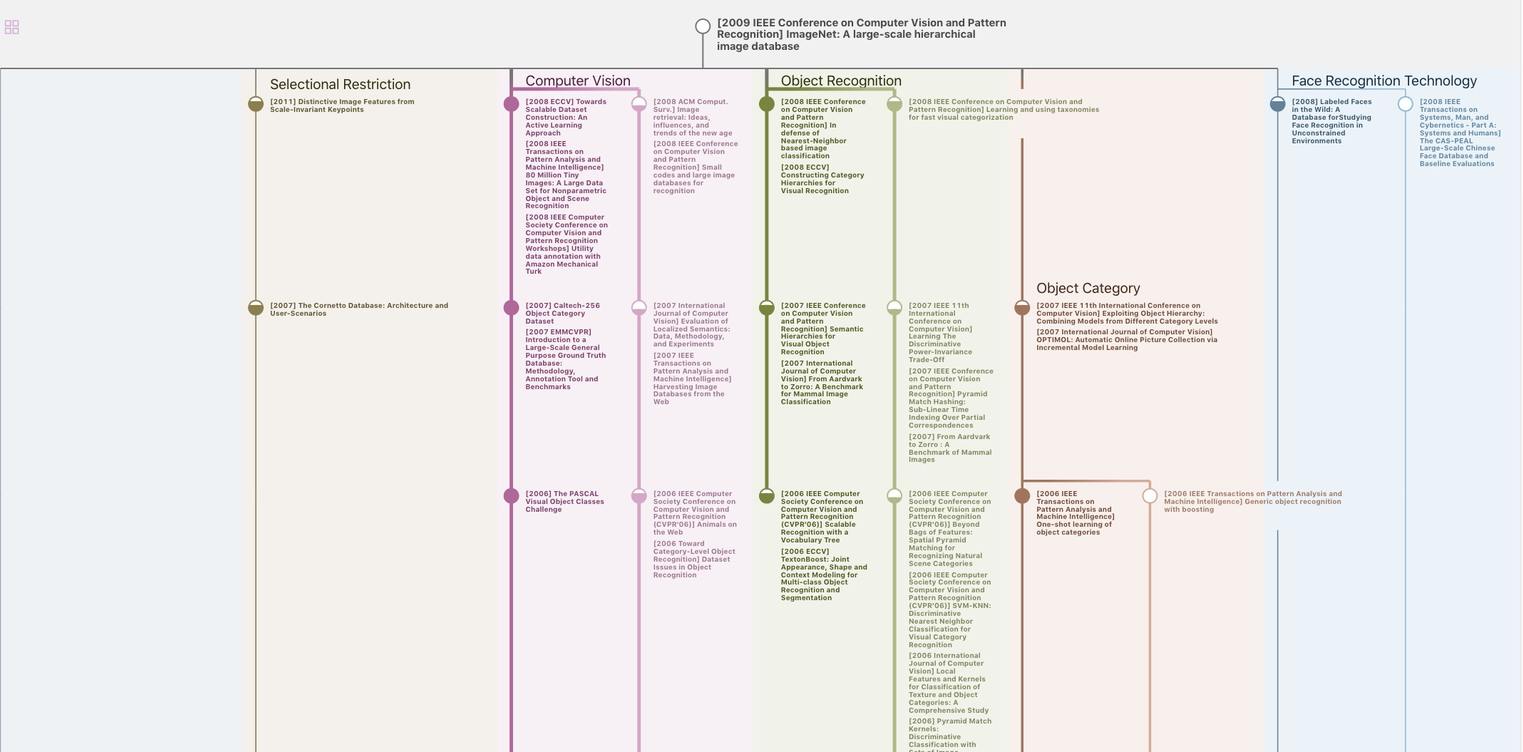
生成溯源树,研究论文发展脉络
Chat Paper
正在生成论文摘要