Lattice Network for Lightweight Image Restoration
IEEE Transactions on Pattern Analysis and Machine Intelligence(2023)
摘要
Deep learning has made unprecedented progress in image restoration (IR), where residual block (RB) is popularly used and has a significant effect on promising performance. However, the massive stacked RBs bring about burdensome memory and computation cost. To tackle this issue, we aim to design an economical structure for adaptively connecting pair-wise RBs, thereby enhancing the model representation. Inspired by the topological structure of lattice filter in signal processing theory, we elaborately propose the lattice block (LB), where couple butterfly-style topological structures are utilized to bridge pair-wise RBs. Specifically, each candidate structure of LB relies on the combination coefficients learned through adaptive channel reweighting. As a basic mapping block, LB can be plugged into various IR models, such as image super-resolution, image denoising, image deraining, etc. It can avail the construction of lightweight IR models accompanying half parameter amount reduced, while keeping the considerable reconstruction accuracy compared with RBs. Moreover, a novel contrastive loss is exploited as a regularization constraint, which can further enhance the model representation without increasing the inference expenses. Experiments on several IR tasks illustrate that our method can achieve more favorable performance than other state-of-the-art models with lower storage and computation.
更多查看译文
关键词
Image Restoration,Lightweight,Lattice Block,Attention,Contrastive Learning
AI 理解论文
溯源树
样例
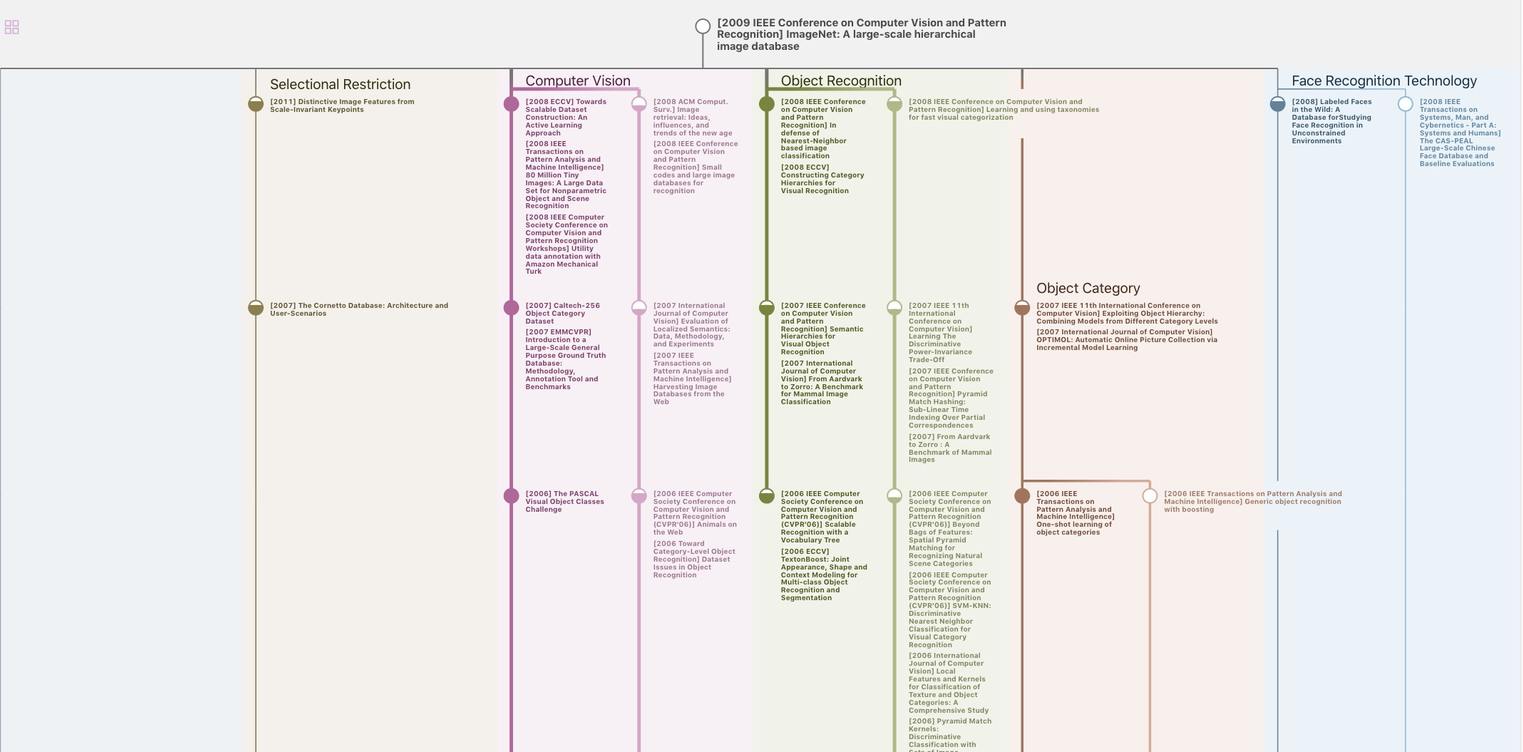
生成溯源树,研究论文发展脉络
Chat Paper
正在生成论文摘要