Examining Humans' Problem-Solving Styles in Technology-Rich Environments Using Log File Data
JOURNAL OF INTELLIGENCE(2022)
摘要
This study investigated how one's problem-solving style impacts his/her problem-solving performance in technology-rich environments. Drawing upon experiential learning theory, we extracted two behavioral indicators (i.e., planning duration for problem solving and human-computer interaction frequency) to model problem-solving styles in technology-rich environments. We employed an existing data set in which 7516 participants responded to 14 technology-based tasks of the Programme for the International Assessment of Adult Competencies (PIAAC) 2012. Clustering analyses revealed three problem-solving styles: Acting indicates a preference for active explorations; Reflecting represents a tendency to observe; and Shirking shows an inclination toward scarce tryouts and few observations. Explanatory item response modeling analyses disclosed that individuals with the Acting style outperformed those with the Reflecting or the Shirking style, and this superiority persisted across tasks with different difficulties.
更多查看译文
关键词
problem-solving style technology-rich environments, experiential learning theory, k-means clustering, explanatory item response modeling, log file data
AI 理解论文
溯源树
样例
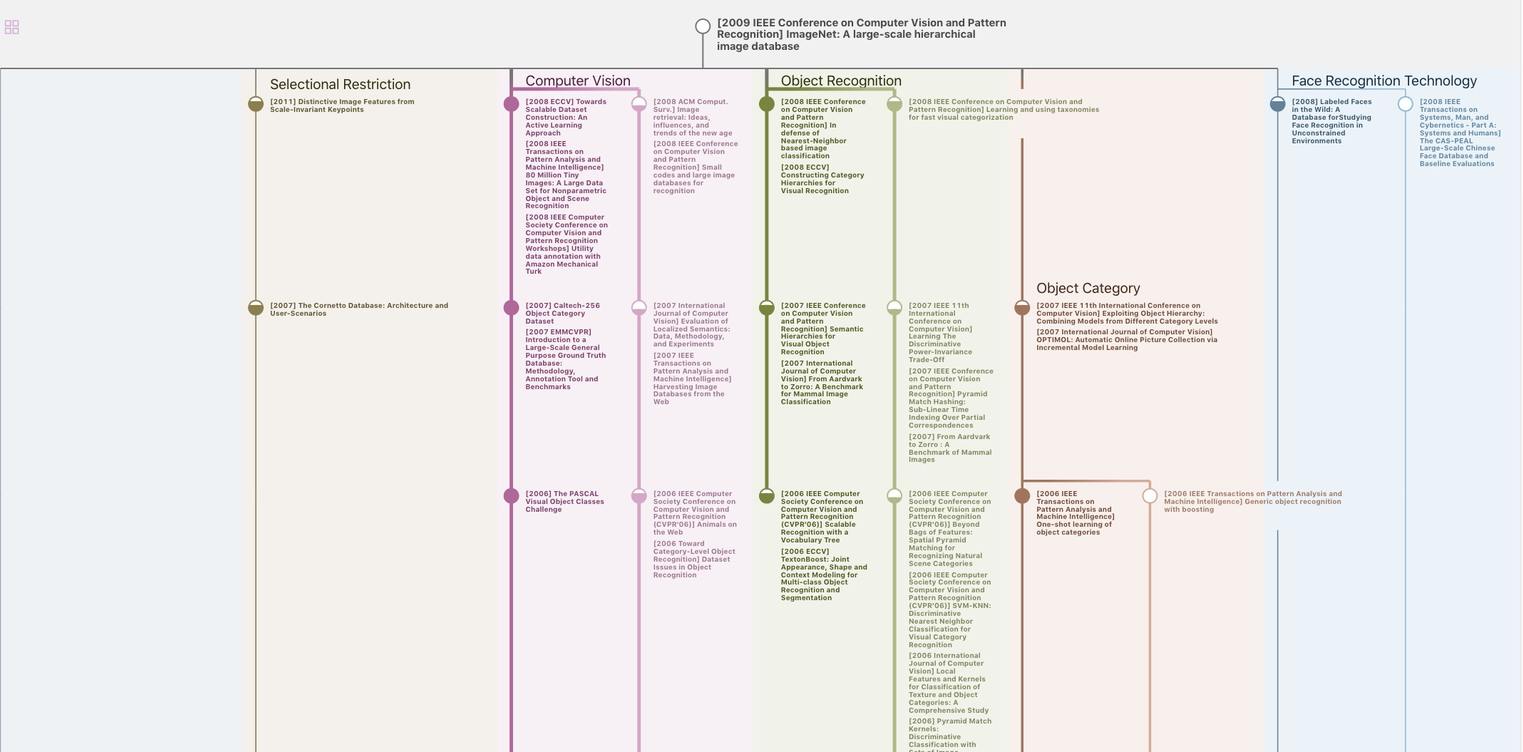
生成溯源树,研究论文发展脉络
Chat Paper
正在生成论文摘要