DeepCrowd: A Deep Model for Large-Scale Citywide Crowd Density and Flow Prediction (Extended abstract)
2022 IEEE 38th International Conference on Data Engineering (ICDE)(2022)
摘要
Predicting the density and flow of the crowd at a citywide level is significant for city management. By meshing a large urban area to a number of fine-grained mesh-grids, citywide crowd and traffic information in a continuous time period can be represented with 4D tensor (Timestep, Height, Width, Channel). Based on this, we revisit the density and in-out flow prediction problem and publish a new aggregated human mobility dataset generated from a real-world smartphone application. Compared with the existing ones, our dataset has larger mesh-grid number, finer-grained mesh size, and higher user sample. Towards such kind of large-scale crowd dataset, we propose a novel deep learning model called DeepCrowd by designing pyramid architectures and high-dimensional attention mechanism based on Convolutional LSTM. Both the datasets and codes are made available at https://github.com/deepkashiwa20/DeepCrowd.
更多查看译文
关键词
Crowd Density,Crowd Flow,Human Mobility,Open Data,Pyramid ConvLSTMs,Deep Learning.
AI 理解论文
溯源树
样例
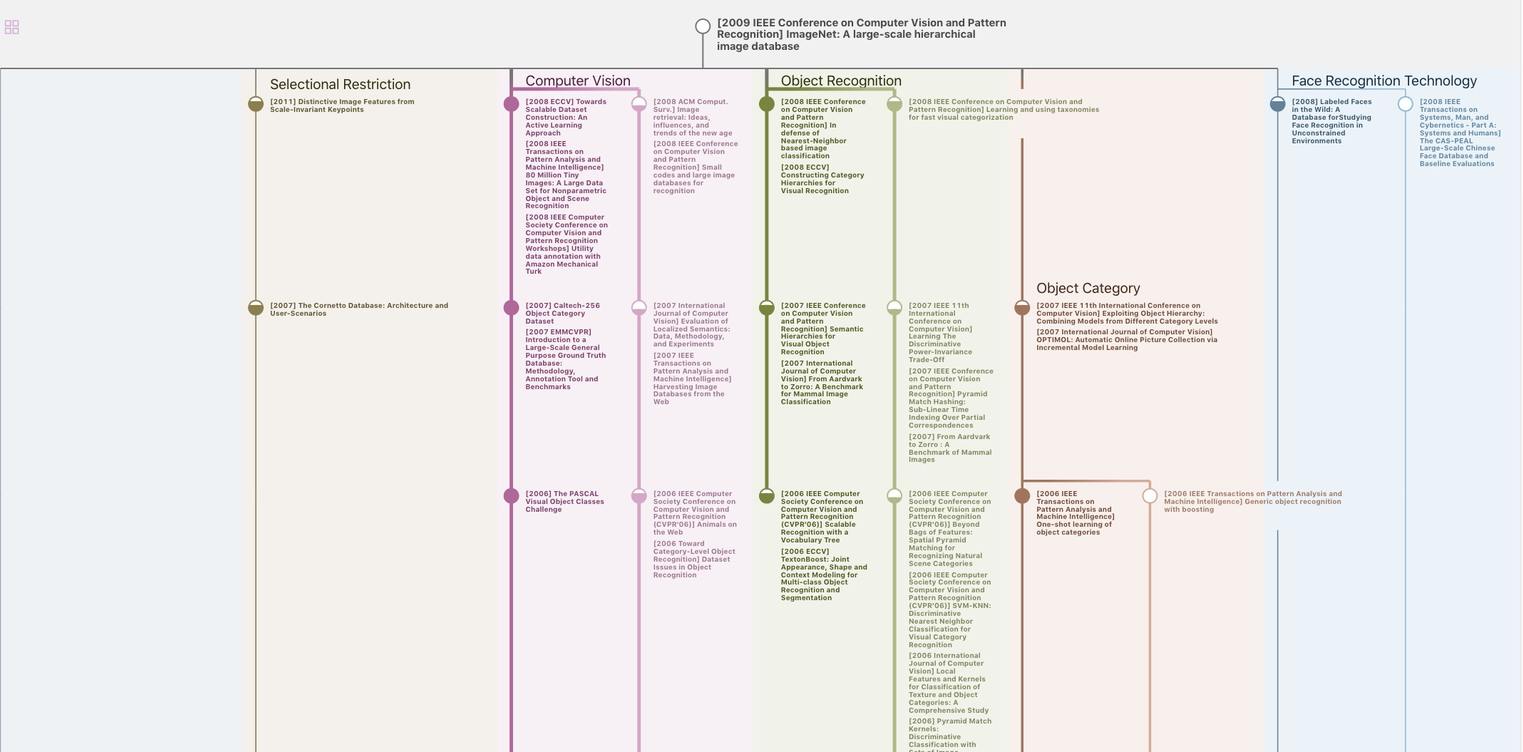
生成溯源树,研究论文发展脉络
Chat Paper
正在生成论文摘要