A Novel Extreme-Learning-Machine Aided Receiver Design for THz-SM with Hardware Imperfections
IEEE Communications Letters(2022)
摘要
Terahertz (THz) communication is promising as it can enable ultra-wide-band and ultra-high-rate for various emerging communication services. In this letter, we propose to exploit the extreme learning machine (ELM) network based regressor for simple and low-complexity joint channel estimation (CE) and signal detection (SD) for THz-band spatial modulation (THz-SM) communications impaired by hardware imperfections. Computer simulations show the performance superiority of the proposed joint CE/SD scheme when compared with the state-of-the-art schemes, and other machine learning-based ones, including the support vector machine (SVM), deep neural network (DNN) and some variants of ELM. Specifically, we show that its bit error rate (BER) performance approaches to that of the recently derived maximal likelihood (ML) SD. In addition, the robustness of the proposed scheme is validated by considering two types of background impulsive noises.
更多查看译文
关键词
Hardware,Support vector machines,Signal detection,Channel estimation,Symbols,Receiving antennas,Indexes,Terahertz (THz) communication,spatial modulation (SM),hardware imperfections,extreme learning machine (ELM),channel estimation (CE),signal detection (SD)
AI 理解论文
溯源树
样例
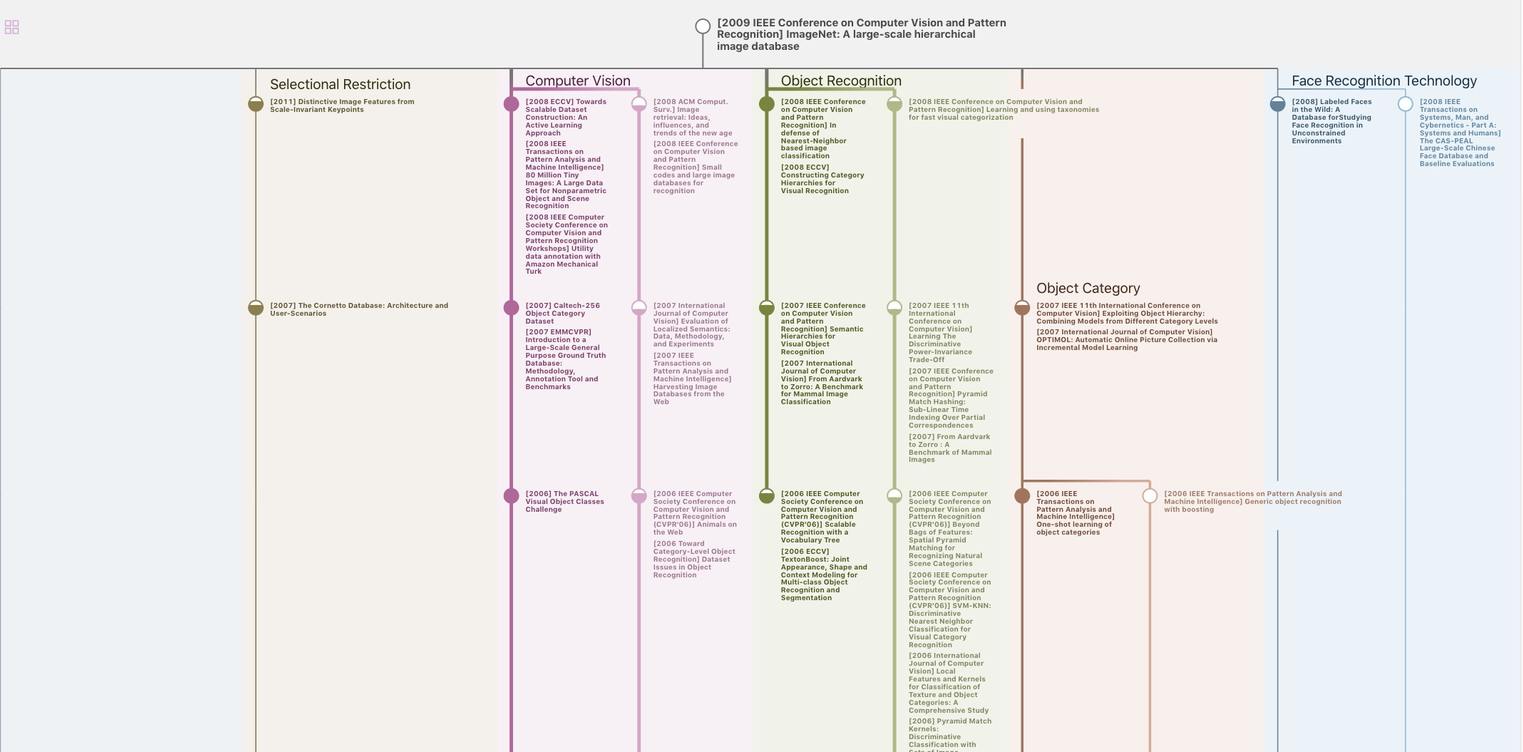
生成溯源树,研究论文发展脉络
Chat Paper
正在生成论文摘要