Meta-Learning Based Classification for Moving Object Trajectories in Mobile IoT
IEEE Transactions on Big Data(2023)
摘要
The proliferation and ubiquity of GPS-enabled mobile Internet of Things (IoT) devices (e.g., drones and unmanned robotic devices) across many disciplines has generated substantial interest in the analysis and mining of trajectory data for enhancing public services such as air pollution monitoring and road safety. Among all trajectory analysis techniques in mobile IoT, classifying moving device trajectories is a fundamental research problem due to its importance for numerous important mobile IoT applications such as travel demand analysis and animal migration patterns. However, existing classification methods for moving object trajectories either are dynamic programming problems (such as similarity-based methods with dynamic time wrapping or Fréchet distance) with a quadratic time complexity in all cases, or need abundant labeled samples to training classification model, thus limit the scalability of these methods for given resource-constrained mobile IoT devices. In this study, we propose a deep learning-based approach for trajectory classification in mobile IoT with linear time complexity by firstly encoding a trajectory as a vector via deep representation learning, then utilizing a meta-learning based approach to learn the ability of classifying trajectory with few annotated samples. Experiments on a massive trajectory dataset show that the proposed approach outperforms state-of-the-art baselines consistently and significantly.
更多查看译文
关键词
Mobile IoT,GPS-enabled devices,trajectory classification,meta-learning
AI 理解论文
溯源树
样例
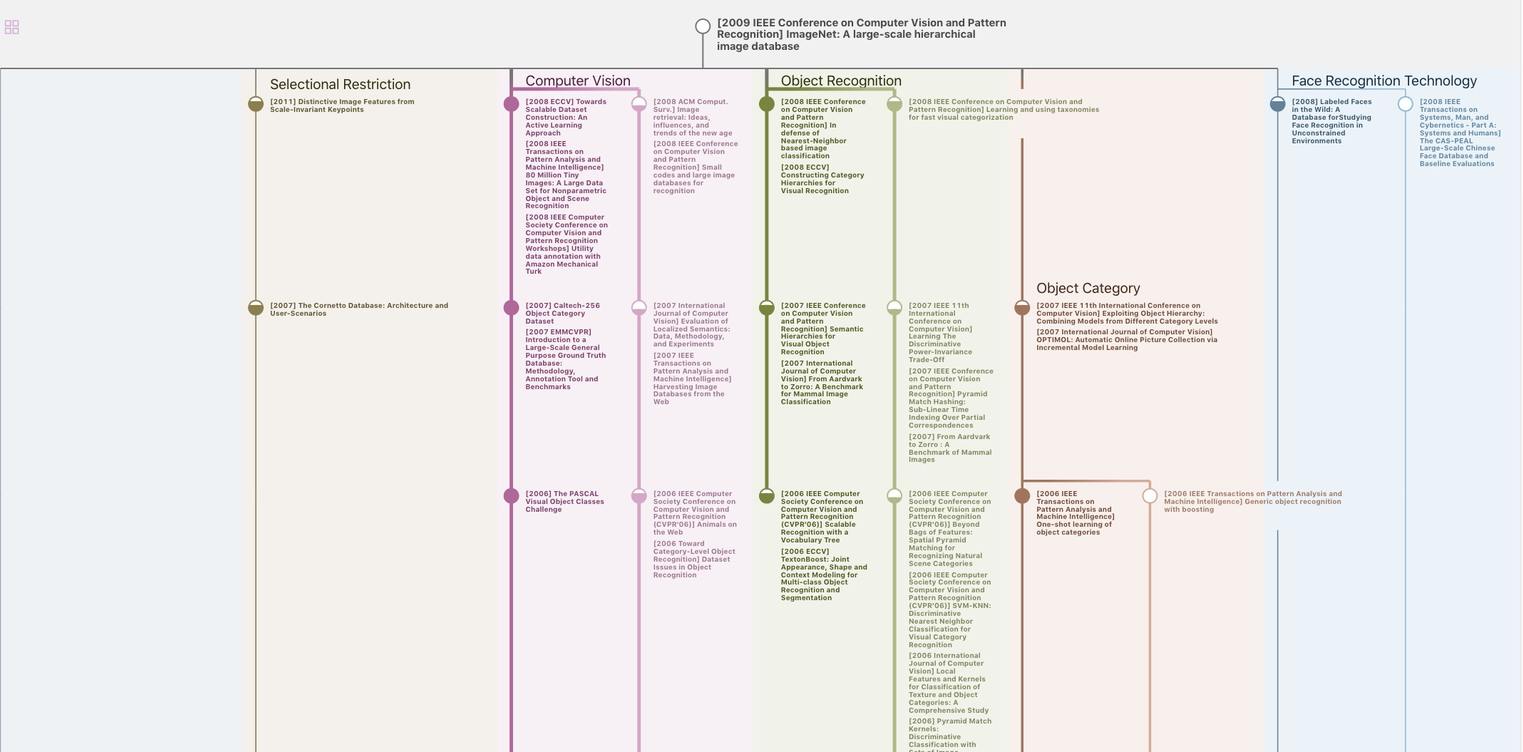
生成溯源树,研究论文发展脉络
Chat Paper
正在生成论文摘要