DPS: Dynamic Pricing and Scheduling for Distributed Machine Learning Jobs in Edge-Cloud Networks
IEEE Transactions on Mobile Computing(2023)
摘要
5G and Internet of Things stimulate smart applications of edge computing, such as autonomous driving and smart city. As edge computing power increases, more and more machine learning (ML) jobs will be trained in the edge-cloud network, adopting the parameter server (PS) architecture. Due to the distinct features of the edge (low-latency and the scarcity of resources), the cloud (high delay and rich computing capacity) and ML jobs (frequent communication between workers and PSs and unfixed runtime), existing cloud job pricing and scheduling algorithms are not applicable. Therefore, how to price, deploy and schedule ML jobs in the edge-cloud network becomes a challenging problem. To solve it, we propose an auction-based online framework DPS. DPS consists of three major parts: job admission control, price function design and scheduling orchestrator. DPS dynamically prices workers and PSs based on historical job information and real-time system status, and decides whether to accept the job according to the deployment cost. DPS then deploys and schedules accepted ML jobs to pursue the maximum social welfare. Through theoretical analysis, we prove that DPS can achieve a good competition ratio and truthfulness in polynomial time. Large-scale simulations and testbed experiments show that DPS can improve social welfare by at least
$95\%$
, compared with benchmark algorithms in today's cloud system.
更多查看译文
关键词
Distributed machine learning, online pricing, online scheduling, parameter server architecture, edge-cloud networks
AI 理解论文
溯源树
样例
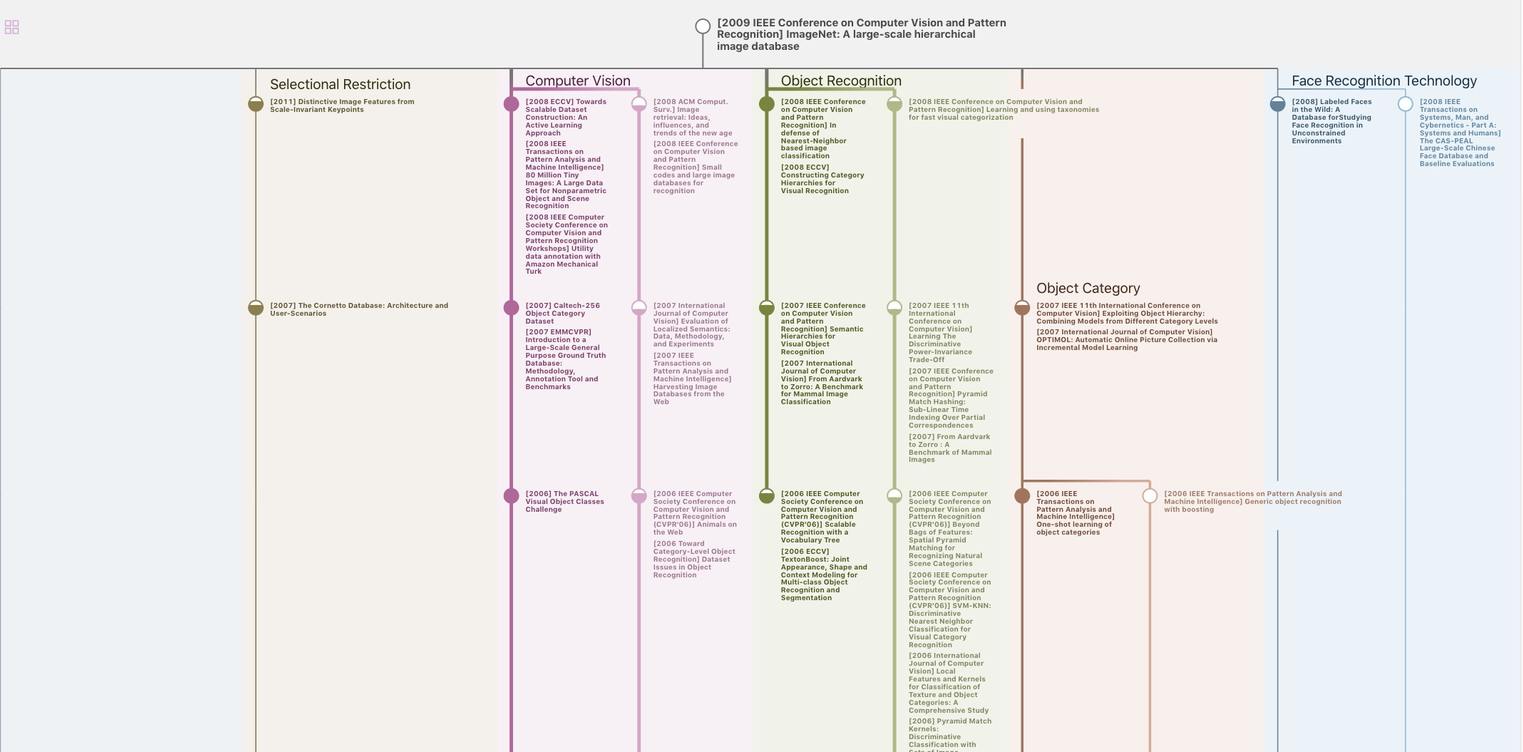
生成溯源树,研究论文发展脉络
Chat Paper
正在生成论文摘要