s-LIME: Reconciling Locality and Fidelity in Linear Explanations
Advances in Intelligent Data Analysis XX(2022)
摘要
The benefit of locality is one of the major premises of LIME, one of the most prominent methods to explain black-box machine learning models. This emphasis relies on the postulate that the more locally we look at the vicinity of an instance, the simpler the black-box model becomes, and the more accurately we can mimic it with a linear surrogate. As logical as this seems, our findings suggest that, with the current design of LIME, the surrogate model may degenerate when the explanation is too local, namely, when the bandwidth parameter
$$\sigma $$
tends to zero. Based on this observation, the contribution of this paper is twofold. Firstly, we study the impact of both the bandwidth and the training vicinity on the fidelity and semantics of LIME explanations. Secondly, and based on our findings, we propose s-LIME, an extension of LIME that reconciles fidelity and locality.
更多查看译文
关键词
Explainable AI, Interpretability
AI 理解论文
溯源树
样例
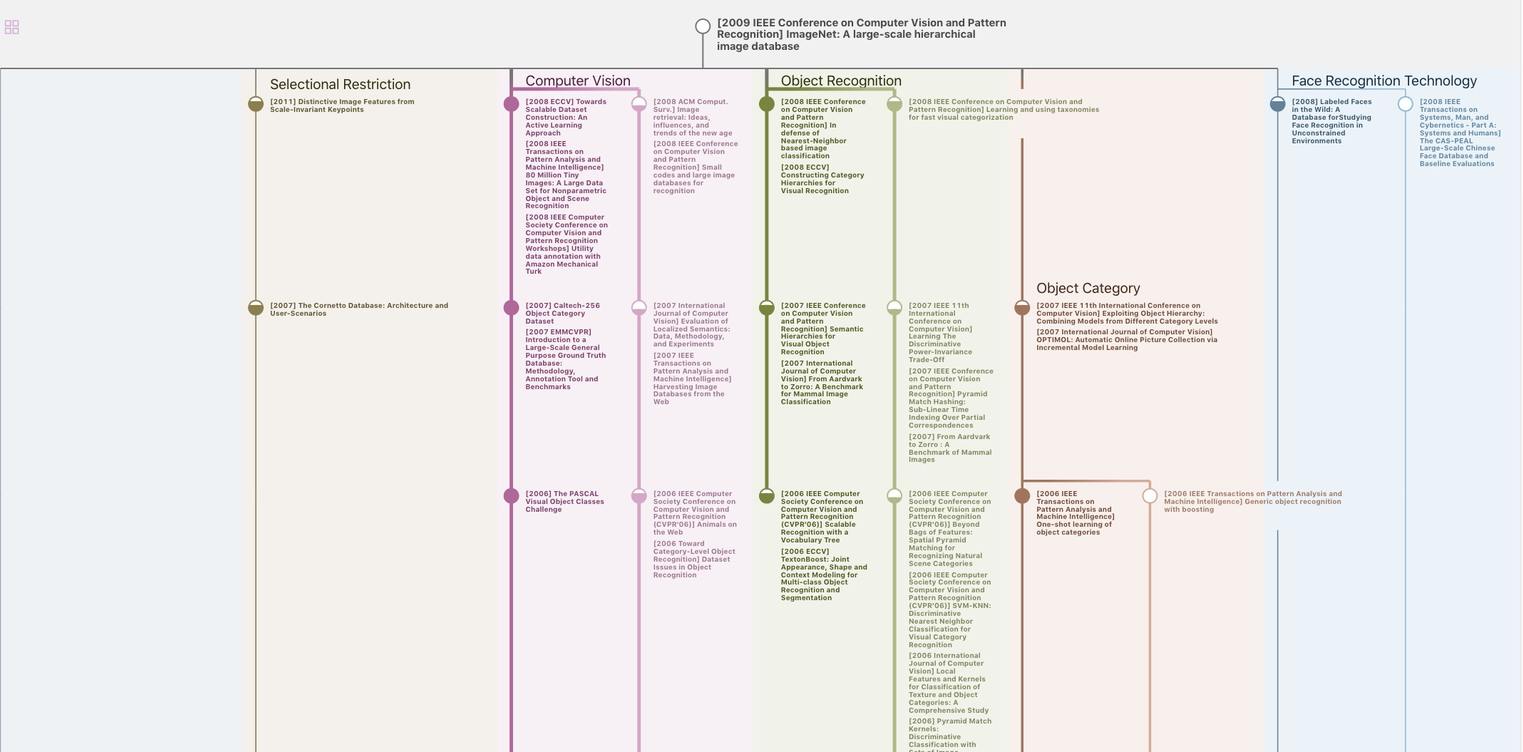
生成溯源树,研究论文发展脉络
Chat Paper
正在生成论文摘要