Joint learning-based causal relation extraction from biomedical literature.
Journal of biomedical informatics(2023)
摘要
Causal relation extraction of biomedical entities is one of the most complex tasks in biomedical text mining, which involves two kinds of information: entity relations and entity functions. One feasible approach is to take relation extraction and function detection as two independent sub-tasks. However, this separate learning method ignores the intrinsic correlation between them and leads to unsatisfactory performance. In this paper, we propose a joint learning model, which combines entity relation extraction and entity function detection to exploit their commonality and capture their inter-relationship, so as to improve the performance of biomedical causal relation extraction. Experimental results on the BioCreative-V Track 4 corpus show that our joint learning model outperforms the separate models in BEL statement extraction, achieving the F1 scores of 57.0% and 37.3% on the test set in Stage 2 and Stage 1 evaluations, respectively. This demonstrates that our joint learning system reaches the state-of-the-art performance in Stage 2 compared with other systems.
更多查看译文
关键词
BEL Statement,Function Detection,Joint Learning,Relation Extraction
AI 理解论文
溯源树
样例
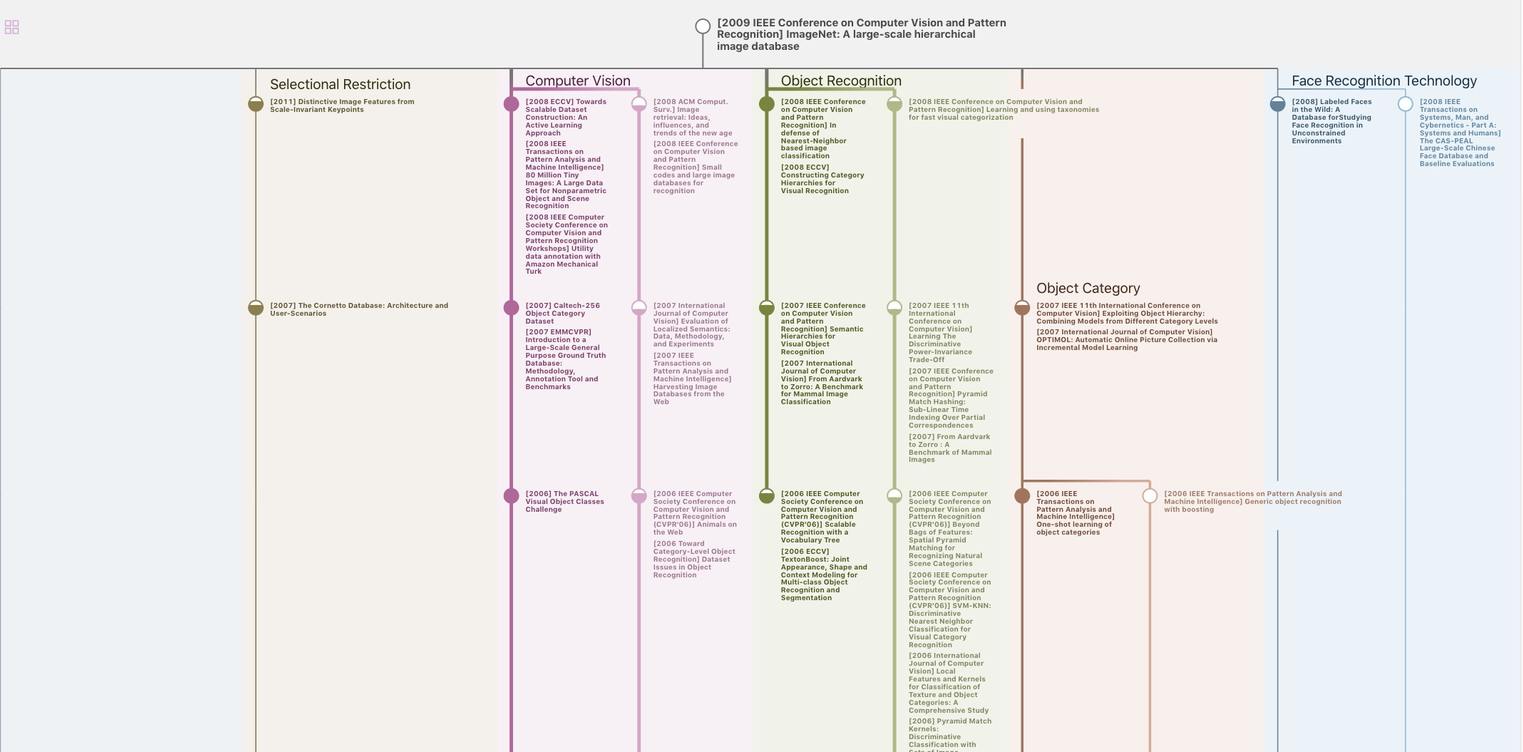
生成溯源树,研究论文发展脉络
Chat Paper
正在生成论文摘要