Toward deep-learning-assisted spectrally-resolved imaging of magnetic noise
arxiv(2022)
摘要
Recent progress in the application of color centers to nanoscale spin sensing makes the combined use of noise spectroscopy and scanning probe imaging an attractive route for the characterization of arbitrary material systems. Unfortunately, the traditional approach to characterizing the environmental magnetic field fluctuations from the measured probe signal typically requires the experimenter's input, thus complicating the implementation of automated imaging protocols based on spectrally resolved noise. Here, we probe the response of color centers in diamond in the presence of externally engineered random magnetic signals, and implement a deep neural network to methodically extract information on their associated spectral densities. Building on a long sequence of successive measurements under different types of stimuli, we show that our network manages to efficiently reconstruct the spectral density of the underlying fluctuating magnetic field with good fidelity under a broad set of conditions and with only a minimal measured data set, even in the presence of substantial experimental noise. These proof-of-principle results create opportunities for the application of machine-learning methods to color-center-based nanoscale sensing and imaging.
更多查看译文
关键词
imaging,deep-learning-assisted deep-learning-assisted,magnetic
AI 理解论文
溯源树
样例
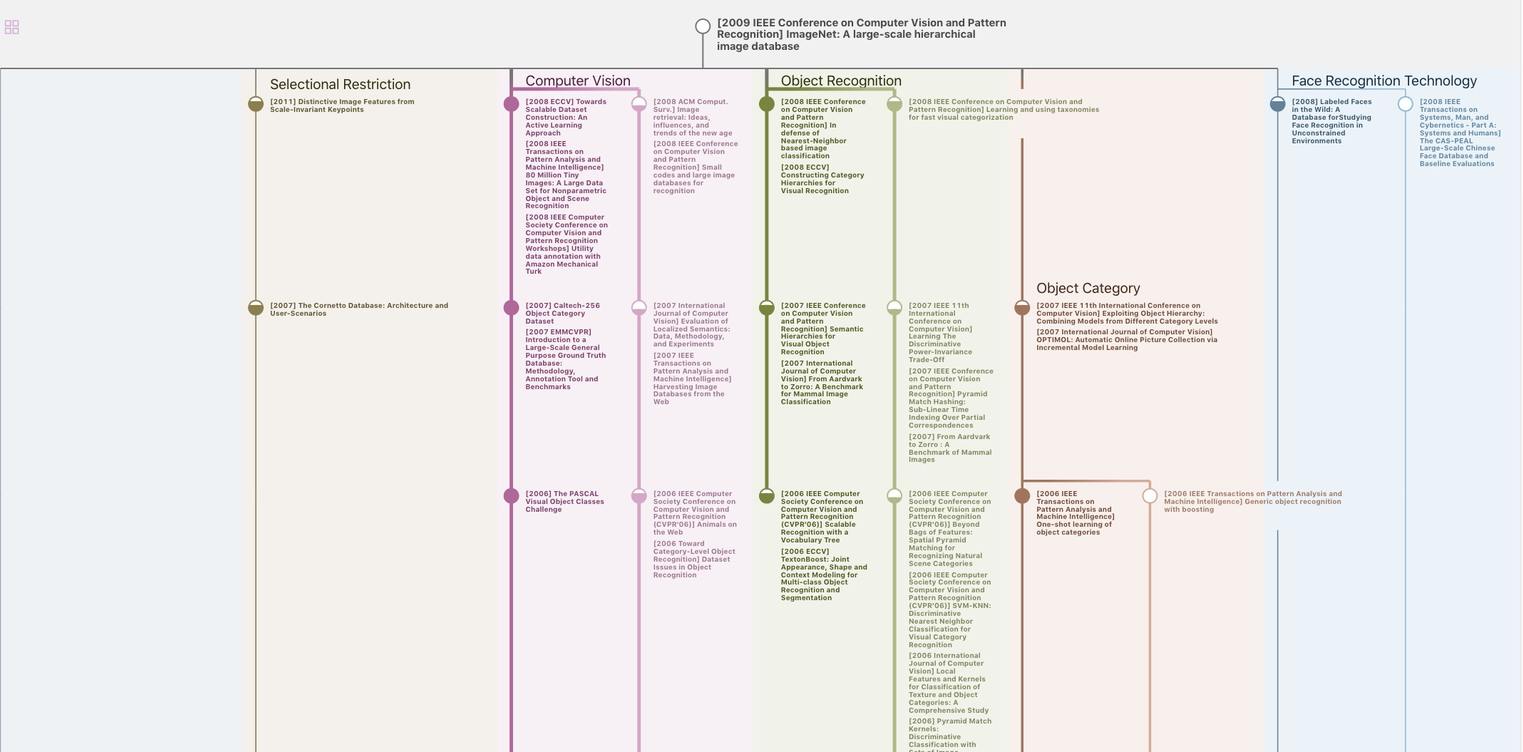
生成溯源树,研究论文发展脉络
Chat Paper
正在生成论文摘要